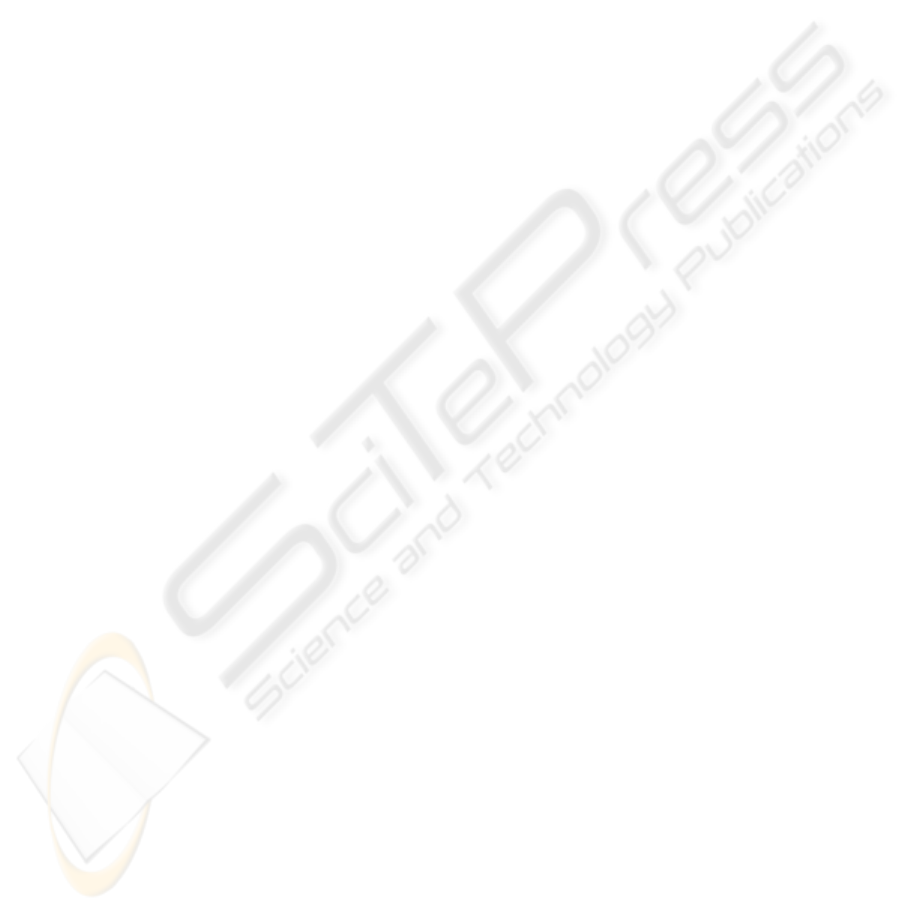
Society Conference on Computer Vision and Pattern
Recognition.
Huang, J. and Ling, C. X. (2005). Using auc and accuracy
in evaluating learning algorithms. IEEE Transactions
on Knowledge Data Eng, 17(3):299–310.
Janasievicz, R., Pinheiro, G., and Facon, J. (2005). Mea-
suring the quality evaluation for image segmentation.
Lecture Notes of Computer Science, 3773:120–127.
Joo, S., Yang, Y., Moon, W., and Kim, H. (2004).
Computer-aided diagnosis of solid breast nodules:
Use of an artificial neural network based on multiple
sonographic features. IEEE Transactions on Medical
Imaging, pages 1292–1300.
Kass, M., Witkin, A., and Terzopoulos, D. (1988). Snakes:
Active contour models. International Journal of Com-
puter Vision, pages 321–331.
Kiyan, T. and Yildirim, T. (2004). Breast cancer diagnosis
using statistical neural networks. Journal of Electrical
& Electronics Engineering, 4:1149–1153.
Martin, D., Fowlkes, C., Tal, D., and Malik, J. (2001). A
database of human segmented natural images and its
application to evaluating segmentation algorithms and
measuring ecological statistics. In Proc. 8th Interna-
tional Conference Computer Vision, volume 2, pages
416–423.
Meyer, F. (1992). Color image segmentation. In Proceed-
ings of the International Conference on Image Pro-
cessing and Its Application, pages 303–306.
Monteiro, F. and Campilho, A. (2006). Performance
evaluation of image segmentation. Lecture Notes of
Computer Science, 4141:248–259.
Polak M, Zhang H, P. M. (2009). An evaluation metric for
image segmentation of multiple objects. Image and
Vision Computing, 27(8):1223–1227.
Popovic, A., de la Fuente, M., Engelhardt, M., and Rader-
macher, K. (2007). Statistical validation metric for
accuracy assessment in medical image segmentation.
International Journal of Computer Assisted Radiology
and Surgery, 2:169–181.
Pratt, W. K. (1997). Digital image processing. John Wiley
and Sons, New York.
Pratt, W. K., Faugeras, O. D., and Gagalowicz, A. (1978).
Visual discrimination of stochastic texture fields.
IEEE Transactions on Systems, Man and Cybernetics
Part B, 8(11):796–804.
Rosenberger, C. (2006). Adaptative evaluation of image
segmentation results. International Conference on
Pattern Recognition, 2:399–402.
Sezgin, M. and Sankur, B. (2004). Survey over image
thresholding techniques and quantitative performance
evaluation. Journal of Electronic Imaging, 13(1):146–
165.
Shentona, M., Gerigb, G., McCarleya, R., Szekelyc, G., and
Kikinisd, R. (2002). Amygdalahippocampal shape
differences in schizophrenia: the application of 3d
shape models to volumetric mr data. Psychiatry
Research Neuroimaging, 115:15–35.
Vilalta, R. and Oblinger, D. (2000). A quantification of
distance bias between evaluation metrics in classifi-
cation. In Proceedings of the Seventeenth Interna-
tional Conference on Machine Learning, pages 1087–
1094.
Yang-Mao, S.-F., Chan, Y.-K., and Chu, Y.-P. (2008). Edge
enhancement nucleus and cytoplast contour detector
of cervical smear images. IEEE Transactions on
Systems, Man and Cybernetics Part B: Cybernetics,
38(2):353–366.
Zhang, H., Cholleti, S., Goldman, S. A., and Fritts, J.
(2006). Meta-evaluation of image segmentation using
machine learning. In IEEE Computer Society Confe-
rence on Computer Vision and Pattern Recognition.
Zhang, H., Frits, J. E., and Goldman, S. A. (2008). Image
segmentation evaluation: A survey of unsupervised
methods. Computer Vision and Image Understanding,
11:260–280.
Zhang, Y. J. (1996). A survey on evaluation methods
for image segmentation. Pattern Recognition,
29(8):1335–1346.
ON SUPERVISED METRICS FOR SHAPE SEGMENTATION
473