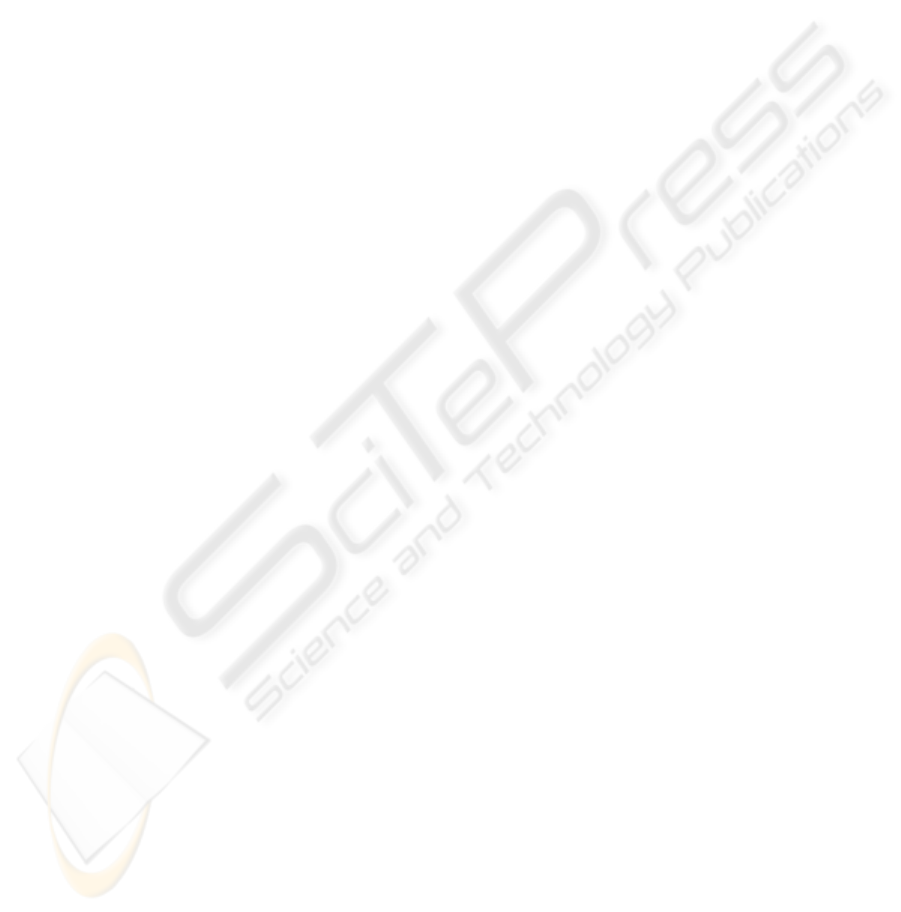
jointly regulate the operon Z. Alon terms the X the
‘general transcription factor’, Y the ‘specific
transcription factor’ and Z the ‘effector operon’.
Feedforward loops are also significant network
motifs in networks with more than three nodes; in
this case, transcription factors X and Y jointly
regulate one or more operons Z(1)…Z(n). The
feedforward loop has other significant
characteristics, of which the most important is
coherence. Shen-Orr describes coherence, “A
feedforward loop motif is ‘coherent’ if the direct
effect of the general transcription factor on the
effector operons has the same sign (negative or
positive) as its net indirect effect through the
specific transcription factor. For example, if X and
Y both positively regulate Z, and X positively
regulates Y, the feedforward loop is coherent. If, on
the other hand, X represses Y, then the motif is
incoherent” (Shen-Orr, Shai, Milo, Mangan and
Alon, 2002). Most feedforward loops are coherent
(85%). The feedforward loop occurs much more
often within the E. coli transcriptional regulation
network than would be expected by random chance.
Shen-Orr suggests that the coherent feedforward
loop has a significant functional structure; the ability
to act as a circuit that rejects transient activation
signals from the general transcription factor and
responds only to persistent signals, while at the same
time allowing a rapid system shut down through the
control of the general transcription factor. This
structure is useful way to coordinate a rapid
response to an external signal. Also, the abundance
of coherent feedforward loops over incoherent loops
suggests a functional design. Lee’s research on the
transcriptional regulatory networks of
Saccharomyces cerevisiae suggest that the
feedforward loop is also a significant network motif
within that system (Lee et al., Science 02). This
suggests that the feedforward loop may also be
significant within other biological system networks.
Additional network motifs emerge as subgraphs of
increasing numbers of nodes are evaluated. When
subgraphs of four nodes are evaluated, the
overlapping regulation motif becomes apparent. In
the overlapping regulation motif, two operons are
regulated by the same two transcription factors.
This type of overlapping regulation motif is a
smaller and specific form of dense overlapping
regulons (DORs), which are discussed later.
Other significant motif patterns within the
transcriptional regulation network of E. coli can be
seen when graphs with higher numbers of nodes are
evaluated. When subgraphs of larger than three
nodes are evaluated, the single input module (SIM)
network motif becomes significant. The SIM is
defined by a set of operons that are controlled by a
single transcription factor, where all of the operons
are under control of the same sign (positive or
negative). There is no additional transcriptional
regulation of the operons. The transcription factors
involved in SIM systems are mostly autoregulatory
(70%). Most of the autoregulatory transcription
factors are autorepressive. There is a higher rate of
autoregulatory transcription factors within SIM
motifs than in the overall system. In the E. coli
transcription regulation network, 70% of the
transcription factors involved in SIM motifs are
autoregulatory, compared to 50% in the overall
dataset. SIMs are found in systems of genes that
function stochiometrically to form a protein
assembly (e.g. flagella) or a metabolic pathway (e.g.
amino acid biosynthesis). SIM systems may involve
temporal ordering, where the first gene activated is
the last to be deactivated.
Dense Overlapping Regulons (DORs) are a type
of network motif found within E. coli when
evaluating larger subnetworks. DORs are composed
of layers of overlapping interactions between
operons and a group of input transcription factors
organized in a bipartite graph that is much more
dense than corresponding structures in randomized
networks. DORs are not a homogenous mesh of
interconnections; rather, they contain several loosely
connected, internally dense regions of combinatorial
interactions. The regions are somewhat overlapping,
and different criteria can yield slightly different
groupings. One way to quantify DORs is by the
frequency of pairs of genes regulated by the same
two transcription factors. Shen-Orr uses a clustering
approach to define DORs. An algorithm detects
locally dense regions in the network with a high
ratio of connections to transcription factors. Within
the E. coli netw
ork, there are six DORs, where
operons in each DOR share common biological
functions. Usually, every output operon is
controlled by a different combination of input
transcription factors, but there are multi-input
modules in rare cases where several operons in a
DOR are regulated by precisely the same
combination of transcription factors with identical
regulation signs (termed ‘multi-input modules’.
DORs are significant in the larger structure of
biological networks; they seem to partition the
operons into biologically meaningful combinatorial
regulation clusters. DORs also govern how several
different network motifs connect together within the
larger network. Shen-Orr describes patterns in the
overall structure of the E. coli network, “A single
BIOINFORMATICS 2010 - International Conference on Bioinformatics
188