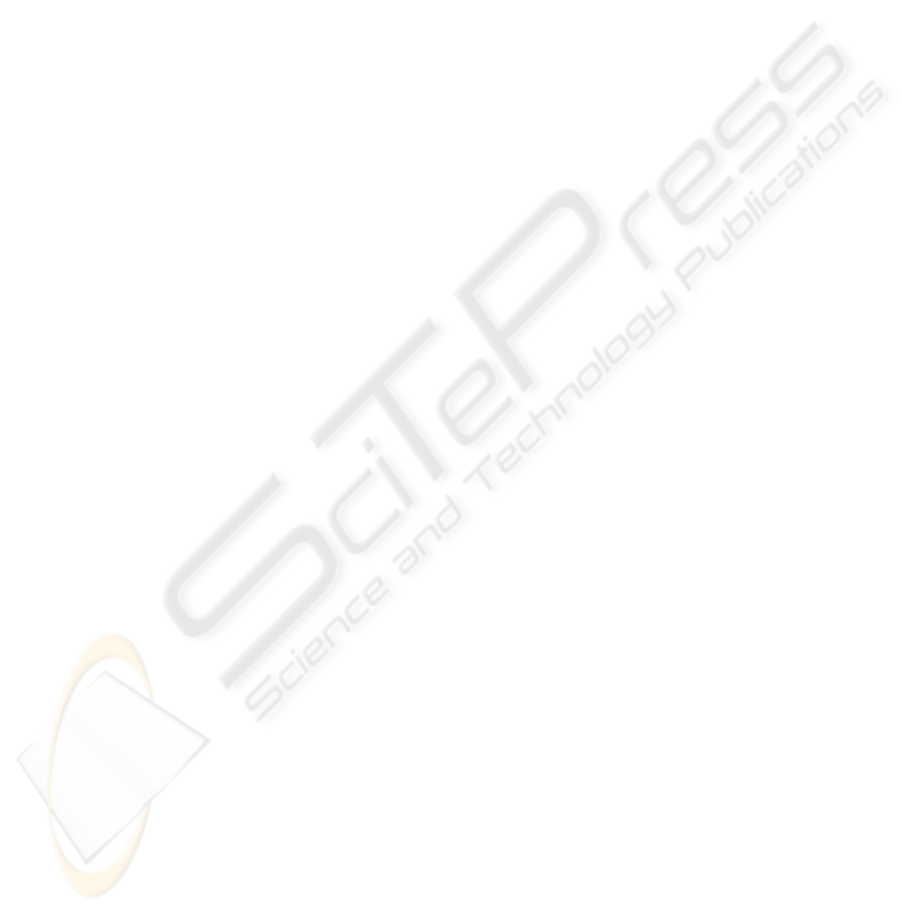
problem instance. Its computation implies an NP-
Hard problem but accurate approximationsare known
(Rish and Dechter, 2000). Given the information pro-
vided by the induced width we can decide whether a
problem instance can be solved only with inference or
not.
3.2 Incomplete Inference
To start with, we can limit the complete method intro-
duced in Section 3.1 using the value of the induced
width. Then, we can use it as a pre-processing or
during systematic search when the induced width of
the problem resulting of some variable assignments
is small enough. An initial work in this field can
be found Max-SAT in (Argelich et al., 2008). How-
ever, from previous approaches in other frameworks,
it seems that this approach is not very competitive in
general (Rish and Dechter, 2000).
Current approaches for solving the SAT problems
apply some kind of incomplete (or limited) inference.
For example, modern SAT solvers apply unit propaga-
tion in order to detect conflicting clauses and then to
learn new clauses in order to boost the search proce-
dure (Silva and Sakallah, 1996). Also, different pre-
processing methods based on limited inference are ap-
plied for local (Cha and Iwama, 1996) and systematic
search (E´en and Biere, 2005).
Recently some techniques from the SAT comunity
have been extended to Max-SAT. First, unit propa-
gation and clause learning can be applied directly to
Max-SAT when the clauses involved are hard (Lar-
rosa et al., 2008; Heras et al., 2008) (i.e, all clauses in-
volved are like (C, ⊤)). But, unit propagation is used
also in Max-SAT to produce as many empty clauses as
possible (i.e., (, m)). As consequence, the problem
is simplified and search is boosted. In (Chu Min Li
and Planes, 2005) a method for detecting inconsis-
tent subsets of clauses via unit propagation is pre-
sented and then in (Heras et al., 2008) such subset
is transformed to an equivalent but simpler subset by
applying the resolution rule for Max-SAT. Note that
the clauses involved in the inconsistent subset are de-
tected via a tree-like refutation.
Observe that one of the most important differences
between the classical resolution rule for SAT and the
novel resolution rule for Max-SAT is that clauses in
SAT can be used once and again during a resolution
process returning an equivalent formula. Differently,
in the Max-SAT context clauses may disappear after a
resolution step and they should be used only once or
at most as many times as their weight is greater than
0 to assure a resulting equivalent formula.
Based on the same idea of using unit propagation
to produce new weighted empty clauses, we propose
to use techniques of detecting unsatisfiable cores to
produceempty clauses. The idea is to apply SAT tech-
niques in order to detect unsatisfiable cores and then
apply Max-SAT resolution if and only if the refutation
is tree-like to assure the correctness of the transfor-
mation. In particular, the Max-SAT problem is solved
assuming all clauses are hard with a SAT solver like
(Silva and Sakallah, 1996) and the information about
the unsatisfiable core is recorded during search. Once
the SAT solver has proved the unsatisfiability, a refu-
tation is built using the information of the unsatisfi-
able core. Then, Max-SAT resolution is applied as
dictated by the refutation if and only if it is tree-like.
However, we think this is unlikely to happen in gen-
eral and time can be prohibitive (each detection of an
unsatisfiable core requires solving a SAT instance).
A more specific recent work on 2-SAT (i.e.,
all clauses have size two) (Buresh-Oppenheim and
Mitchell, 2006) shows how minimum tree-like refuta-
tions can be built for 2-SAT in polynomial time. Ob-
serve that the same process can be applied for Max-
SAT but in order to simplify the formula as much as
possible. This may be specially powerful for prob-
lems with lots of weighted clauses of arity 2 such as
the general Max-2-SAT problem, the Max-CUT prob-
lem or the spin-glass problem.
Finally, we propose to study new forms of lim-
ited inference for problems with a very specific struc-
ture. In (Heras and Larrosa, 2008) we presented pre-
processing that exploits the synergy of two resolution-
based rules in order to simplify problems by a set of
hard clauses of size 2 and a set of soft clauses of size
1. Observe that prominent optimization problems can
be encoded in this way such as the Maximum Clique
Problem, Minimum Vertex Covering, Maximum Inde-
pendet Set, etc. Results indicated that a systematic
search solver is boosted several orders of magnitude
after the pre-processing for some problem instances.
3.3 Local Search
The first successful local search solver for SAT was
GSAT (Selman et al., 1992) that is a simple best-
improvement search algorithm. Performance im-
provements were achieved by the usage of techniques
to avoid falling in local minima, that is, areas where
no improvements can be reached. Major improve-
ments were obtained with the development of the
WALKSAT architecture (Selman et al., 1993). In each
search step, WALKSAT algorithms first choose a cur-
rently unsatisfied clause and then flip a variable oc-
curring in this clause, that is, the value of the variable
is changed from true to false or viceversa. Current
ICAART 2010 - 2nd International Conference on Agents and Artificial Intelligence
650