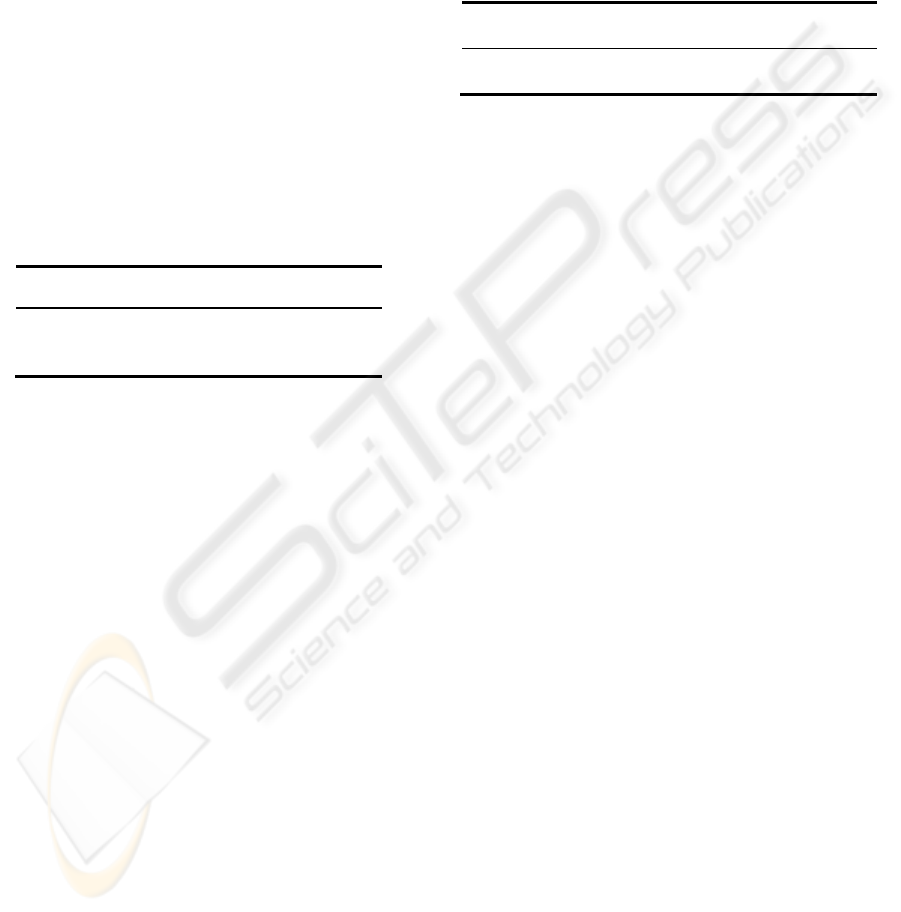
was made up of 15 young elite skaters. Thirty is a
reasonable number of patients, taking into account
that the tests are quite complex (a single patient
check-up, including the above three tests, occupies
2-3 Mb).
The ultimate aim of the evaluation is to measure
how good the model generation method is. To do
this, we have created two models from each of the
above groups of sportspeople. These two models are
actually composed of three submodels, one for each
individual test (UNI, RWS and LOS). The first
model (M
basketball
) was created from a training set
composed of 10 of the 15 basketball players. The
other 5 players constituted the test set. The second
model (M
skating
) was generated from a training set
composed of 10 of the 15 skaters. The other 5
skaters were used as test set. The sportspeople in the
test set were chosen at random from all the
sportspeople in each group. Table 1 summarizes the
above.
Table 1: Model data distribution.
Model #Training Set #Test Set
M
basketbal
l
10 5
M
skatin
10 5
To evaluate both models, they were used to
classify patients in the test sets. The aim is to check
whether the M
basketball
model properly represents the
group of professional basketball players and whether
the M
skating
model is representative of the group of
elite skaters. Note that the method proposed here is a
modelling not a classification method. To test how
good the method is at creating models, we are going
to evaluate whether the created models are useful for
classification. However, time series modelling has
many other applications like, for example, feature
identification across a group of time series or model
comparison measuring the likeness among groups of
time series or the evolution of one and the same
group over time. In actual fact, in many domains,
like medicine, the mere observation of the model by
the expert can turn out to be very useful in the
decision-making process.
To enact the classification process, we have
compared each of the ten individuals in the test
group against each of the two created models,
making use of the stabilometric time series
comparison method described in (Lara et al., 2008).
All sportspeople have been classified taking into
account how similar they are to the two created
models, selecting the model most like the patient in
question as the class. As regards the five skaters in
the test set, four were correctly classified as skaters.
The fifth could not be successfully classified
because it was very similar to both models. On the
other hand, the five basketball players were correctly
classified as basketball players.
Table 2 summarizes the results. It shows that, of
the ten elite sportspeople that were used to test the
created models, nine were classified correctly within
the respective model of their sports speciality.
Table 2: Sportspeople classification results.
Sport
#Successfully
Classified
#Wrongly
Classified
Basketball 5 0
Skatin
41
Considering the results, we conclude that the models
generated by our method represent reliably
population groups according to their balance-related
abilities. These results were considered very
satisfactory by both the research team and the expert
physicians. This has encouraged the physicians to
continue cooperating in this field.
REFERENCES
Chan, P. K., Mahoney, M. V., 2005. Modeling Multiple
Time Series for Anomaly Detection, Fifth IEEE
International Conference on Data Mining, ICDM.
Chen, Z., Yang, B. R., Zhou, F. G., Li, L. N., Zhao, Y. F.,
2008. A New Model for Multiple Time Series Based
on Data Mining, International Symposium on
Knowledge Acquisition and Modeling, KAM.
Lara, J. A., Moreno, G., Pérez, A., Valente, J. P., López-
Illescas, A., 2008. Comparing Posturographic Time
Series through Event Detection, 21st IEEE
International Symposium on Computer-Based Medical
Systems, CBMS.
Papadimitriou, S., Sun, J., Faloutsos, C., 2005. Streaming
Pattern Discovery in Multiple Time-Series, 31st VLDB
Conference, Trondheim, Norway.
Povinelli, R., 2000. Using Genetic Algorithms to find
Temporal Patterns indicative of Time Series Events,
GECCO Workshop: Data Mining with Evolutionary
Algorithms.
HEALTHINF 2010 - International Conference on Health Informatics
488