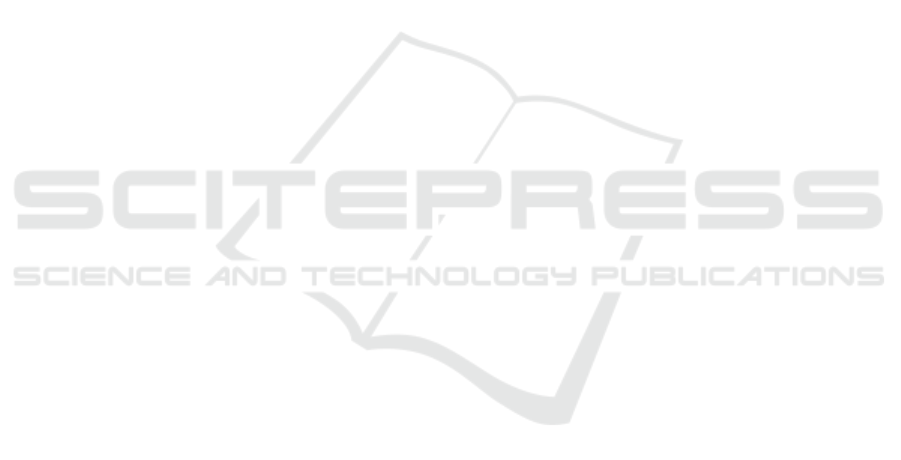
to color having red for negative, and blue for positive
news with increasing intensity. The news mainly re-
port on Central Asia and North Korea in the particular
time period. The majority of these news is negative
in their tonality. The Cartogram representation (right
figure) enhances the area of these important locations.
The bottom row shows the polarity score of
news on terrorism related topics. Polarity scores are
mapped to color having red for negative, and blue
for positive news with increasing intensity. The news
mainly report on the Middle East and Central Asia,
especially on the events in Sri-Lanka that occurred
in the particular time period. Although the majority
of the news is negative in its tonality, there are some
positive reports on successes in the fight on terrorism.
The Cartogram representation (right figure) enhances
the area of these important locations.
4 CONCLUSIONS
The current paper describes an application framework
for analyzing and exploring real-time news feed data.
Polarity analysis showed how to assess the ”tonal-
ity” of the published news articles using a technique
called Literature Fingerprinting. The geospatial anal-
ysis demonstrated that many insights can be gained
using pixel-based approaches. The great challenge
for further research is to integrate respective tech-
niques within the EMM-platform, make them scalable
to large datasets, and to cope with real-time require-
ments.
ACKNOWLEDGEMENTS
This material is based upon work supported by the
Science and Technology Directorate of the U.S. De-
partment of Homeland Security under Grant Award
Number 2008-ST-108-000002. The views and con-
clusions contained in this document are those of the
authors and should not be interpreted as necessarily
representing the official policies, either expressed or
implied, of the U.S. Department of Homeland Secu-
rity.
REFERENCES
Atkinson, M. and Van der Goot, E. (2009). Near real time
information mining in mulitlingual news. In WWW
’09: Proc. 18th int. conference on World Wide Web,
pages 1153–1154. ACM.
Bak, P., Keim, D. A., Schaefer, M., Stoffel, A., and Omer, I.
(2009a). Spatiotemporal analysis of sensor logs using
growth ring maps. In IEEE Trans. On Visualization
And Computer Graphics. IEEE Press.
Bak, P., Mansmann, F., Janetzko, H., and Keim, D. A.
(2009b). Density equalizing distrortion of large ge-
ographic point sets. In J. of Cartographic and Geo-
graphic Information Science, volume 36(3).
Gamon, M., Aue, A., Corston-Oliver, S., and Ringger, E.
(2005). Pulse: Mining customer opinions from free
text. In Advances in Intelligent Data Analysis VI,
pages 121–132.
Hu, M. and Liu, B. (2004). Mining and summarizing
customer reviews. In KDD ’04: Proc. 10th ACM
SIGKDD int. conference on Knowledge Discovery
and Data Mining, pages 168–177. ACM.
Keim, D. A., Bak, P., and Schaefer, M. (2009). Dense
pixel displays. In Encyclopedia of Database Systems.
Springer.
Keim, D. A. and Oelke, D. (2007). Literature fingerprint-
ing: A new method for visual literary analysis. In
IEEE Symposium on Visual Analytics and Technology
(VAST 2007), pages 115–122.
Liu, B., Hu, M., and Cheng, J. (2005). Opinion observer:
analyzing and comparing opinions on the web. In
WWW ’05: Proc. 14th int. conference on World Wide
Web, pages 342–351. ACM.
Morinaga, S., Yamanishi, K., Tateishi, K., and Fukushima,
T. (2002). Mining product reputations on the web. In
KDD ’02: Proc. 8th ACM SIGKDD int. conference on
Knowledge Discovery and Data Mining, pages 341–
349. ACM.
Pang, B. and Lee, L. (2008). Opinion mining and sentiment
analysis. Foundations and Trends in Information Re-
trieval, 2(1-2):1–135.
Titov, I. and McDonald, R. (2008). A Joint Model of Text
and Aspect Ratings for Sentiment Summarization. In
Proceedings of ACL-08: HLT, pages 308–316. Assoc.
for Computational Linguistics.
Tobler, W. R. (2004). Thirty five years of computer car-
tograms. In Association of American Geographers,
volume 94(1), pages 58–73.
Wanner, F., Rohrdantz, C., Mansmann, F., Oelke, D., and
Keim, D. A. (2009). Visual sentiment analysis of rss
news feeds featuring the us presidential election in
2008. In Workshop on Visual Interfaces to the Social
and the Semantic Web (VISSW 2009).
Wiegand, M. and Klakow, D. (2008). Optimizing language
models for polarity classification. In Macdonald, C.,
Ounis, I., Plachouras, V., Ruthven, I., and White,
R. W., editors, ECIR, volume 4956 of Lecture Notes
in Computer Science, pages 612–616. Springer.
Yu, H. and Hatzivassiloglou, V. (2003). Towards answer-
ing opinion questions: separating facts from opinions
and identifying the polarity of opinion sentences. In
Proc. 2003 conference on Empirical methods in Natu-
ral Language Processing, pages 129–136. Assoc. for
Computational Linguistics.
WEBIST 2010 - 6th International Conference on Web Information Systems and Technologies
268