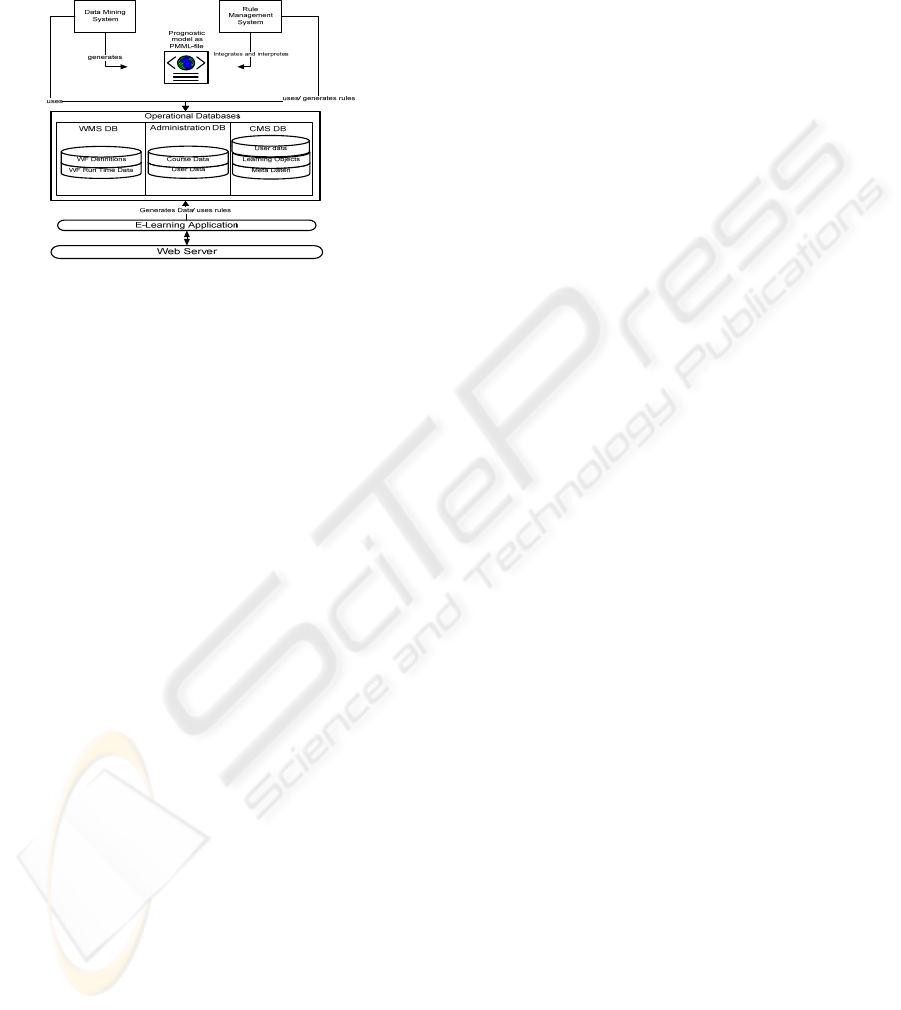
combined in a process model by the teacher. The
time for learning and studying learning objects built
according to this process model can be reduced by
ensuring a higher learning effecivity in contrast to a
scenario which does not employ the process model.
Figure 4: Implementation Model.
5 CONCLUSIONS
The presented approach partly responds to the need
for efficient technical systems as well as innovative
didactical methods to support the knowledge
exchange by enabling the control, analysis and
improvement of already executed e-learning
processes and can even support not yet executed e-
learning processes.
The approach reveals high potentials for use in
companies and educational institutions, e.g. gain of
valuable time and effort eliminating ineffective
learning procedures and accelerating the learning
process of complex topics. Nevertheless, there is still
a need for evaluations in practice. Further studies
could deal with more concrete implementation
scenarios going further into technical detail or a
substantiated cost-benefit-ratio.
To complete the analysis of the potentials of the
approach there is a need to render the application of
process mining in e-learning marketable and ready
for implementation. Furthermore the method can be
analyzed in order to identify possibilities to fill the
gap of missing learning process models and lack of
learning process adaptability.
ACKNOWLEDGEMENTS
The authors gratefully acknowledge comments
received on earlier versions of this paper from
Michael Doktor, Sathya Laufer and Matthias Barz.
REFERENCES
Cesarini, M., Monga, M., & Tedesco, R. (2004). Carrying
on the e-learning process with a workflow
management engine. In Proceedings of the 2004 ACM
symposium on Applied computing (S. 940 - 945).
Nicosia, Cyprus : Association for Computing
Machinery.
Datta, A. (1998). Automating the Discovery of As-Is
Business Process Models: Probabilistic and
Algorithmic Approaches. Information Systems
Research, 9 (3), 275-301.
de Medeiros, A. A., Weijters, T. A., & van der Aalst, W.
(September 2005). Genetic Process Mining: A Basic
Approach and its Challenges. In Proceedings of the
Workshop on Business Process Intelligence. Nancy,
France.
Federal Ministry of Education and Research (Germany)
(2009). bmbf.de – Lifelong Learning. Retrieved
09/09/2009 from bmbf.de:
http://www.bmbf.de/en/411.php.
Franconi, E., & Sattler, U. (1999). A Data Warehouse
Conceptual Data Model for Multidimensional
Aggregation. In Proceedings of the International
Workshop on Design and Management of Data
Warehouses.
Frosch-Wilke, D.; Sànchez-Alonso, S.; Dodero, J.M.
(2008). Evolutionary Design of Collaborative
Learning Processes through Reflective Petri Nets. In
Proceedings of the 8th IEEE International Conference
on Advanced Learning Technologies, Santander
(Spain), pp. 423-427
Grob, H. L., Bensberg, F. & Coners, A. (April 2008).
Regelbasierte Steuerung von Geschäftsprozessen -
Konzeption eines Ansatzes auf Basis des Process
Mining. Die WIRTSCHAFTSINFORMATIK, pp.
268-281.
Helic, D. (2006). Technology-Supported Management of
Collaborative Learning Processes. International
Journal of Learning and Change, Vol. 1, No. 3, pp.
285-298.
Ingvaldsen, J.E., & J.A. Gulla. (2006). Model Based
Business Process Mining. Journal of Information
Systems Management, Vol. 23, No. 1, Special Issue on
Business Intelligence, Auerbach Publications
Palmer, C.-E. (2003). E-Learning - die Revolution des
Lernens gewinnbringend einsetzen. In U. Scheffer, &
M. Charlier (Eds.), E-Learning (pp. 13 ). Stuttgart,
Germany : Klett-Cotta Verlag.
Schönwälder, S. (1997). Portfoliomanagement für
betriebliche Informationssysteme: computergestützter
Ansatz zur partizipativen Einführung und Gestaltung.
Wiesbaden, Germany: Gabler.
DISCUSSION OF THE BENEFIT POTENTIALS OF PROCESS MINING FOR E-LEARNING PROCESSES
411