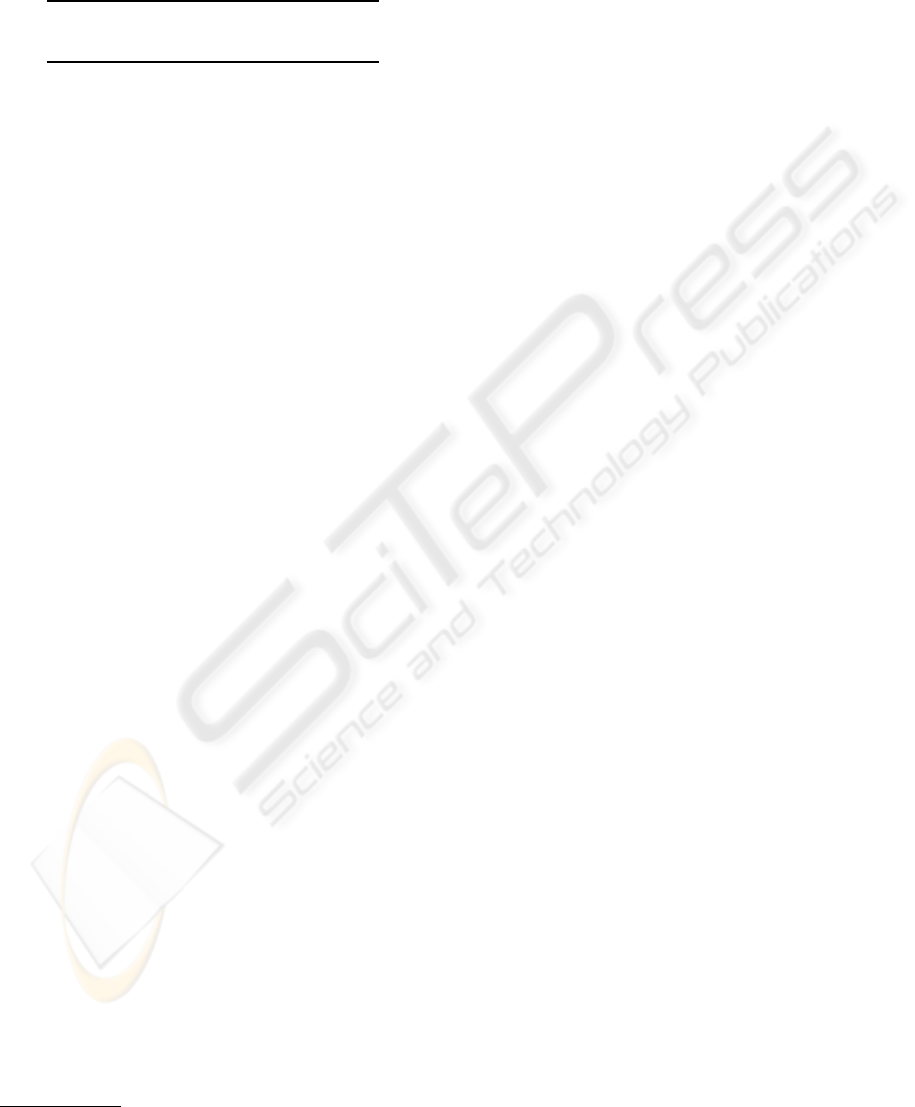
Table 4: The numbers of user pairs who have similar styles
of writing and submitted answers to the same questions.
frequency of submissions
to the same questions
category one or more ten or more
PC 87 12
healthcare 17 0
social issues 109 22
used a Japanese morphological analyzer, Mecab
5
, for
word segmentation of messages.
In experiment 1, we first developed user classifiers
by applying maximum entropy (ME) method to the
training data. Then, we varied the numbers of input
messages to the classifiers and measured the accuracy
of them. Input messages were extracted from the ex-
perimental data for examination. Figure 4 shows the
accuracy of the classifiers under the various numbers
(1 ∼ 5) of input messages and the various sizes (100,
200, and 300 messages) of training data. As shown in
Figure 4, we obtained more than 95% accuracy when
we set the size of training data and the number of in-
put messages to be 300 (including 150 target user’s
messages) and 4, respectively. Furthermore, we found
character 3-gram (s3) and 1 ∼ 10 characters at the
head and end of sentences (s5 and s6) are effective to
this experiment.
In experiment 2, we measured the accuracy of the
identifier. It consisted of N classifiers, the accuracy
of which are shown in Figure 4. Figure 5 shows the
accuracy of the identifier under the various numbers
(1 ∼ 25) of input messages and the various sizes (100,
200, and 300 messages) of training data. As shown in
Figure 5, we obtained more than 80% accuracy when
we set the size of training data and the number of in-
put messages to be 300 (including 150 target user’s
messages) and 15, respectively.
In experiment 3, because we wanted to use the
identifier with more than 85 % accuracy, we gave
training data consisting of 300 messages (including
150 target user’s messages) and set the number of in-
put messages to be 16. Table 4 shows the numbers
of user pairs who have similar styles of writing and
submitted answers to the same questions. In this ex-
periment, we found two user pairs suspected of pre-
tending to be someone else to manipulate communi-
cations. Those user pairs submitted answers to the
same questions in social issues category 43 and 17
times, respectively. We intend to examine whether
these user pairs are multiple account users, from var-
ious perspectives.
5
http://mecab.sourceforge.net/
5 CONCLUSIONS
In this paper, we proposed a method of detecting users
who have similar styles of writing and submitted an-
swers to the same questions in a community site fre-
quently. Our method detected some user pairs sus-
pected of pretending to be someone else and manip-
ulating communications in a community site. We in-
tend to examine this experimental results and refine
our method. Then, we wish to contribute to learners
in community sites.
ACKNOWLEDGEMENTS
This research has been supported partly by the
Grant-in-Aid for Scientific Research (C) under Grant
No.20500106.
REFERENCES
Craig: Authorial attribution and computational stylistics:
if you can tell authors apart, have you learned any-
thing about them?, Literary and Linguistic Comput-
ing, 14(1), (1999).
de Vel, Anderson, Corney, and Mohay: Mining e-mail con-
tent for author identification forensics, ACM SIG-
MOD Record, 30(4), (2001).
Koppel, Argamon, and Shimoni: Automatically Categoriz-
ing Written Text by Gender, Literary Linguistic and
Computing, 17(4), (2002).
Corney, de Vel, Anderson, and Mohay: Gender-Preferential
Text Mining of E-mail Discourse, ACSAC 2002,
(2002).
Argamon, Saric, and Stein: Style mining of electronic mes-
sages for multiple authorship discrimination: first re-
sults, 9th ACM SIGKDD, (2003).
Zheng, Li, Chen, and Huang: A Framework of Author-
ship Identification for Online Messages: Writing Style
Features and Classification Techniques, Journal of the
American Society for Information Science and Tech-
nology, 57(3), (2006).
Odaka, Murata, Gao, Suwa, Shirai, Takahashi, Kuroiwa,
and Ogura: A Proposal on Student Report Scoring
System Using N-gram Text Analysis Method, IEICE
trans., J86-D-I(9), (2003).
Tsuboi and Matsumoto: Authorship Identification for Het-
erogeneous Documents, ISPJ-NL-148, (2002).
Matsumoto, Takamura, and Okumura: Sentiment Classifi-
cation using Word Sequences and Dependency Trees,
FIT2004, (2004).
DETECTION OF SUBMITTERS SUSPECTED OF PRETENDING TO BE SOMEONE ELSE TO MANIPULATE
COMMUNICATIONS IN A COMMUNITY SITE
171