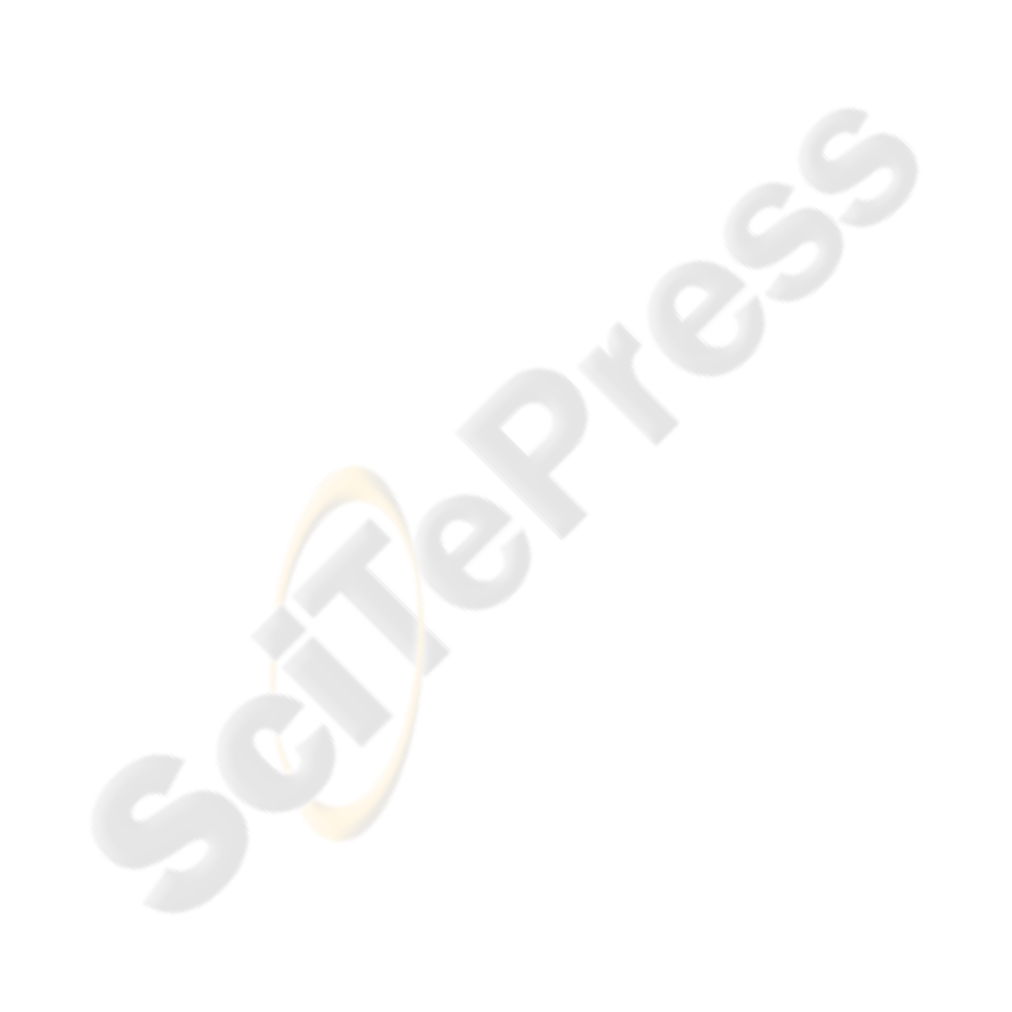
role (e.g., in transition countries: market economy,
ownership, etc.). Students are only ones who would
potentially introduce simulation methodology into
practice. However, how to present modelling and
simulation (MS) is of enormous importance to the
students, whose personal experience is limited.
This paper describes over 30 year of experience
in the teaching of a modelling and simulation course
for students of the Faculty for Organizational
Sciences. The main objective of the course has been
to show the role of modelling and simulation in
management science in teaching the students the
methods and techniques of modelling as well as
general notation in the form of computer simulator,
accompanied by presenting the field of application,
effectiveness and facets at the support of business
decisions. Our course consists of two parts:
continuous simulation based on systems dynamics
and discrete event simulation (DES). The course is
in the third year and students by the time they start
the course they have already taken courses of
mathematics, statistics, theory of systems, as well as
organizational and economic courses. The final
grade of the course is derived from the student’s
project and written exam.
In this paper, we will discuss methods of
teaching SD. Of course, by definition, simulation is
experimentation on a computer model. It is a typical
virtual reality method, which can alienate students
from real management problems. In order to
motivate students in learning and understanding the
subject, many authors have developed business
simulators of various types. One of most popular is
the beer game simulator developed at MIT (Sterman,
2000). Therefore, we have also developed a
simulation model (Škraba et. al, 2003) in order to
clarify the usefulness of the simulation in solving
management problems. Students took part in an
experiment where they had to solve a managerial
decision problem supported by the simulation
model. They were assigned to work under different
experimental conditions. Experimental results were
then analyzed and discussed in the students’
projects. Students’ contributions were rewarded as a
part of their final grade. Also, students were kept
motivated throughout the course by special rewards
for their in-class participation. After the experiment,
students had to complete a questionnaire on their
opinions. The results show that management
students, taking the course Modelling and
Simulation, thought that application of the
simulation model contributes to a greater
understanding of the problem in comparison to those
who did not participate in the course with the
business simulator. In this paper, we analyzed
methods of teaching MS and the impact of the
business simulator and active participation of the
students during lecturing on their grades.
2 BUSINESS SIMULATOR – A
TOOL TO IMPROVE
LEARNING PROCESS
In order to improve our method of teaching
modelling and simulation and to persuade students
that simulation methods in management science are
not only a tool for solving already known academic
problems, we built a business simulator aimed at
presenting decision processes in enterprises more
realistically. Students have to take active part in an
experiment and then make reports about their
results. In this way, they were motivated to regularly
attend and understand lectures. However, if one
wants to persuade participants to experiment with a
stimulator, it has to be carefully prepared; the design
of the experiment has to be as realistic as possible in
order to show this advantage of using a simulation
model in decision support. For that purpose, the
business simulator has to reasonably reflect the
business situation and its utility.
A simulation model developed by the SD
method, which was used in the experiment, is shown
in Figure 1. The model described by Škraba et. al
(2003), consists of production, workforce and
marketing segments, which are well known in
literature (Sterman, 2000). It was stated that product
price (r1) positively influences income. However, as
prices increase, demand decreases below the level it
would otherwise have been. Therefore, the proper
pricing that customers would accept can be
determined. If marketing costs (r3) increase, demand
increases above what it would have been as a result
of marketing campaigns. The production system
must provide the proper inventory level to cover the
demand, which is achieved with the proper
determination of the desired inventory value (r4).
Surplus inventory creates unwanted costs due to
warehousing; therefore, these costs have to be
considered. The number of workers employed is
dependent on the production volume and workforce
productivity, which is stimulated through salaries
(r2). Proper stimulation should provide reasonable
productivity.
CSEDU 2010 - 2nd International Conference on Computer Supported Education
28