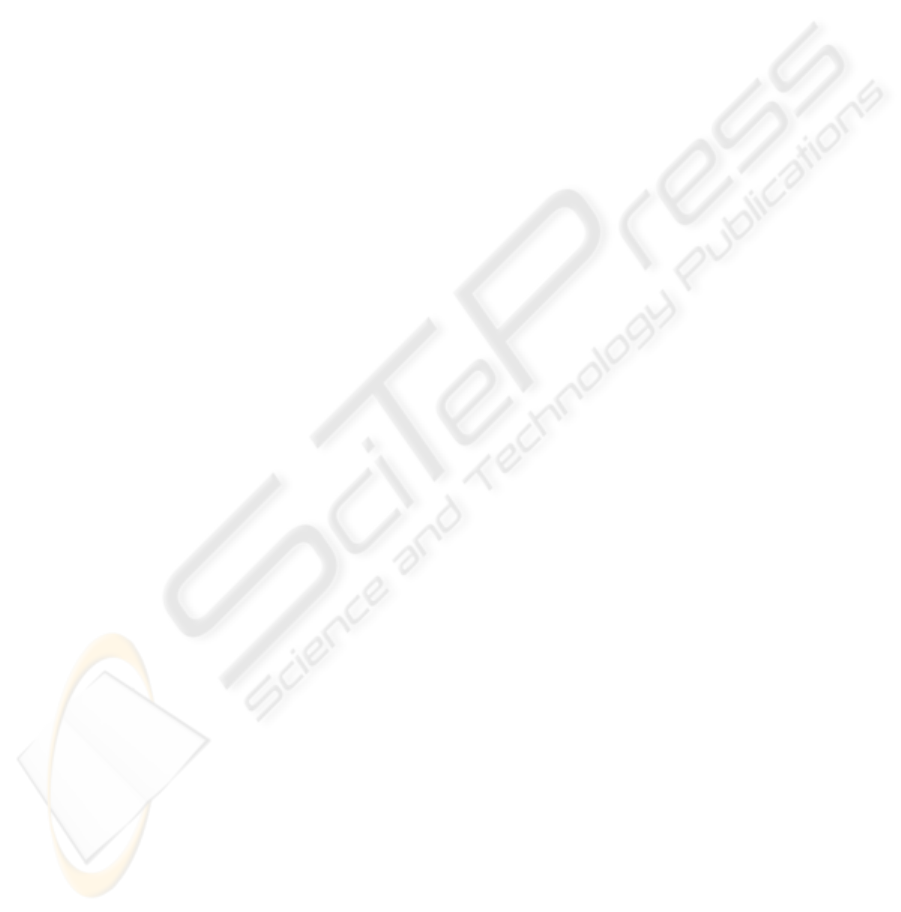
model permits us to identify new kinds of possible
issues for a Marine unit such as forgetting a step in a
procedure, performing steps in the wrong order, or
repeating steps unnecessarily.
5 EXTENDING THE
TECHNOLOGY TO NEW
APPLICATIONS
Our technology could make important contributions
to physical education and choreography which have
previously focused heavily on the performance of
the individual in isolation. Just looking at our
metrics, instruction in team sports could benefit
from measurements of dispersion, clustering,
coverage, mobility, speed, and leadership centrality.
Instruction in choreography (Smith-Autard, 2004)
could benefit from measurements of dispersion,
collinearity, lines of sight (from our "danger"
calculation), and being too close to objects. Our
more global behavior analysis could provide
valuable information about pacing for both.
In general, our technology should help quantify a
range of physical-motion skills that are historically
hard to evaluate fairly (Hay, 2006). (Coker, 2004)
provides a taxonomy of errors in motor skills: those
due to task constraints, comprehension, perceptions
for decisionmaking, decisionmaking itself, recall of
previous learning, neuromuscular limits, improper
speed-accuracy tradeoffs, visual errors, and
proprioceptive errors. Our noninvasive motion-
monitoring technology should help particularly with
perceptions for decisionmaking, decisionmaking
itself, and visual errors, and will indirectly help with
recall of previous learning, neuromuscular limits,
speed-accuracy tradeoffs, and proprioceptive errors.
However, there remain important instructional issues
to study in the kind, timeliness, and frequency of the
new kinds of feedback from our technology to
students, as with any instructional technology.
ACKNOWLEDGEMENTS
This work was sponsored by the U.S. Office of
Naval Research. Opinions expressed are not
necessarily those of the U.S. Government.
REFERENCES
Chen, Y.-J., Hung, Y.-C., 2009. Using real-time
acceleration data for exercise movement training with
a decision tree approach. Proc. 8th Intl. Conf. on
Machine Learning and Cybernetics, Baoding, CN,
July 2009.
Cheng, H., Kumar, R., Basu, C., Han, F., Khan, S.,
Sawhney, H., Broaddus, C., Meng, C., Sufi, A.,
Germano, T., Kolsch, M., and Wachs, J., 2009. An
instrumentation and computational framework of
automated behavior analysis and performance
evaluation for infantry training. Proc.I/ITSEC,
Orlando, Florida, December.
Coker, C., 2004. Motor learning and control for
practitioners. McGraw-Hill, New York.
Hay, P., 2006. Assessment for learning in physical
education. In Kirk. D., MacDonald, D., O'Sullivan,
M., The handbook of physical education, Sage,
London.
Hixson, J., 1995. Battle command AAR methodology: a
paradigm for effective training. Proc. 27th Winter
Simulation Conf., Arlington, Virginia, USA, pp. 1274-
1279.
Knight, J., Bristow, H., Anastopoulou, S., Baber, C.,
Schwritz, A., Arvantitis, T., 2007. Uses of
accelerometer data collected from a wearable system..
Personal and Ubiquitous Computing, 11, pp. 117-132.
Minnen, D., Westeyn, T., Ashbrook, D., Presti, P., Starner,
T., 2007. Recognizing soldier activities in the field.
Proc. Conf. on Body Sensor Networks, Aachen,
Germany, March.
Nakatsu, R., Tadenuma, M., Maekawa, T., 2001.
Computer technologies that support Kansei expression
using the body. Proc. Intl. Conf. on Multimedia,
September, Ottawa, Canada.
Rowe, N., 2009. Automated instantaneous performance
assessment for Marine-squad urban-terrain training.
Proc. Intl. Command and Control Research and
Technology Symposium, Washington, DC, June.
Sadagic, A., Welch, G., Basu, C., Darken, C., Kumar, R.,
Fuchs, H., Cheng, H., Frahm, J.-M., Kolsch, M.,
Rowe, N., Towles, H., Wachs, J. and Lastra, A., 2009.
New generation of instrumented ranges: enabling
automated performance analysis. Proc.I/ITSEC,
Orlando, Florida, December.
Smith-Autard, J., 2004. Dance composition, 5th ed. A&C
Black, London.
Stepan, V., Zara, J., 2002. Teaching tennis in virtual
environment. Proc. 18th Spring Conf. on Computer
Graphics, Budmerice, Slovakia, pp. 49-54.
Wachs, J., Goshorn, D., Kolsch, M., 2009. Recognizing
human postures and poses in monocular still images.
Proc. Intl. Conf. on Image Processing, Computer
Vision, and Pattern Recognition.
AUTOMATED ASSESSMENT OF PHYSICAL-MOTION TASKS FOR MILITARY INTEGRATIVE TRAINING
195