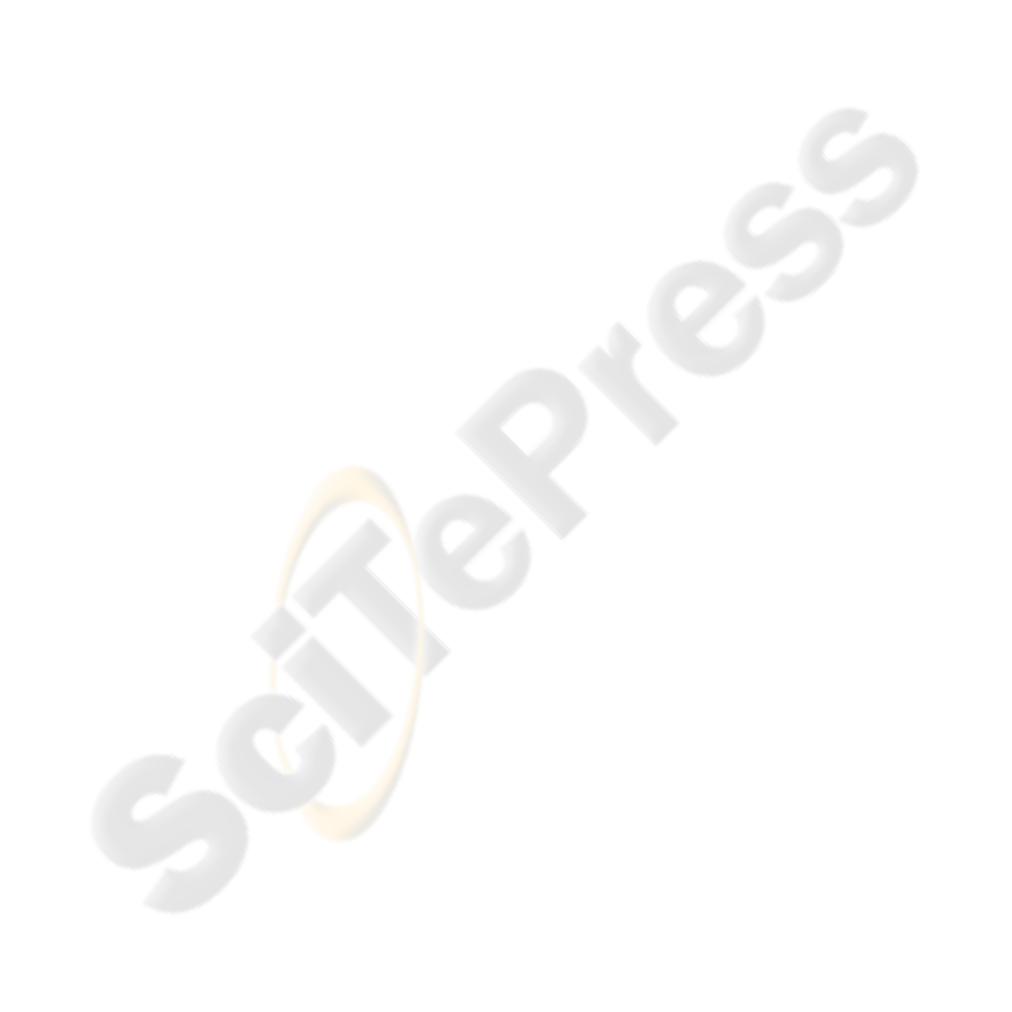
agents are employed, the adaptive behaviour of
societies. AB is for example the most used approach
for representing Game Theory, (e.g.: Power, 2009;
Remondino and Cappellini, 2005), spontaneous
aggregation phenomena, and so on.
4.1 Pros and Cons
Besides having different application fields, as
discussed, each of the reviewed paradigms has some
points of strength and weakness. DE modelling has,
as a first fundamental strength when used as a
teaching support, to be able to show, through a flow
chart (which is something very straightforward and
cognitively powerful) how a process works and
operates. Each activity can be explained in details,
through completion time, required resources,
preconditions, local outputs and so on. Besides, after
defining the framework defining each part of the
process, the output could be measured by means of
simulation; the average time for process completion
can be calculated, as well as the costs, possible
bottle necks, redundant resources. This is a very
strong way of showing how deterministic or
stochastic processes work, and can be the basis for
scenario and what-if analysis. Another point of
strength for DE modelling is the easiness of data
interpretation; if structural values are used correctly,
the results are directly comparable with the real
world situation that has been modelled. Besides, DE
models are not too difficult to build and modify,
thanks to well known and widespread standards
(e.g.: UML) and easy-to-use visual tools. Last but
not least, when building a DE model, the real world
situation has to be studied in detail and decomposed,
first. This requires a theoretical and deep pre-
modelling study, which is very useful for students.
On the other hand, DE modelling has some
weaknesses; first, it’s not always easy or even
possible to divide a process (especially if complex
and social) into basic activities, without severe
approximations: for example a Financial market.
Besides, the model has a deterministic or stochastic
nature, thus making it impossible to realistically
emulate human-like behaviour and human factor in
the most general extent. Another limitation is that
the whole schema is built a priori (the flow) and is
not subject to structural changes during the
simulation. Eventually, it’s worth mentioning that
it’s quite difficult to have more big processing to
cross interact among them, unless loosing detail or
expressive power, which is something fundamental
for educational purposes. As to SD, its biggest point
of strength for educational purposes is the easiness
to transmit the connections and interactions among
macro systems. This is a fundamental part for
Economic systems (markets) and Finance. Feedback
loops are highlighted and so cause/effect relations
among the components. Another important and
natural pro of SD is the fact that stock variables and
flow variables are identified and represented. This is
exactly the way in which many accounting and
Economic systems work; e.g.: the flow of new
investment (positive flow) and the depreciation or
depletion (negative flow) determine the stock of
capital. Last but not least, as for DE modelling, there
are many visual tools to build SD simulations, thus
overcoming possible technical difficulties. A first
drawback of SD technique is that it usually gives
general “average” results for the macro classes
represented. For example, in a market model, it will
represents the average investments for enterprises, or
the average value added for customers and so on.
The modeller has to think in terms of global
structural dependencies and has to provide accurate
quantitative data for them. Since SD is at an high
abreaction level, it’s difficult – or even impossible –
to explore lower levels, thus showing to students and
learners how individual sub-parts actually influence
the overall behaviour of the class that comprises it.
When building a SD model, it’s necessary to
define relations among the parts through
mathematical formalisms. This is not always
possible, especially in social and financial systems;
while a general and theoretical market can be well
described, some investment and negotiation
strategies based on perception cannot be represented.
The relations among the macro classes are then
static and immutable during the simulation process.
Essentially, as for DE simulation, the PC calculates
the overall result given a mathematical framework
and some initial parameters, but cannot change the
way in which these macro classes interact among
them. According to (Bonabeau, 2002), AB
modelling has three main benefits, over other
approaches: it captures emergent phenomena; it
provides a natural description of a system; and it is
flexible. In fact, as already noted, AB simulation is
the widest applicable approach, in the sense that it
can be used for exploring problems at different
levels (from an higher abstraction, like markets or
societies, to lower levels, like individual behaviours
for the agents). Besides this is possible in the same
model, making the different level interconnected
among them. The bottom-up approach used for
building AB models is most natural for educational
purposes, since it examine a situation from the
perspective of the single entities involved. The
TEACHING MANAGEMENT AND FINANCE THROUGH SIMULATION - Choosing the Proper Paradigm
467