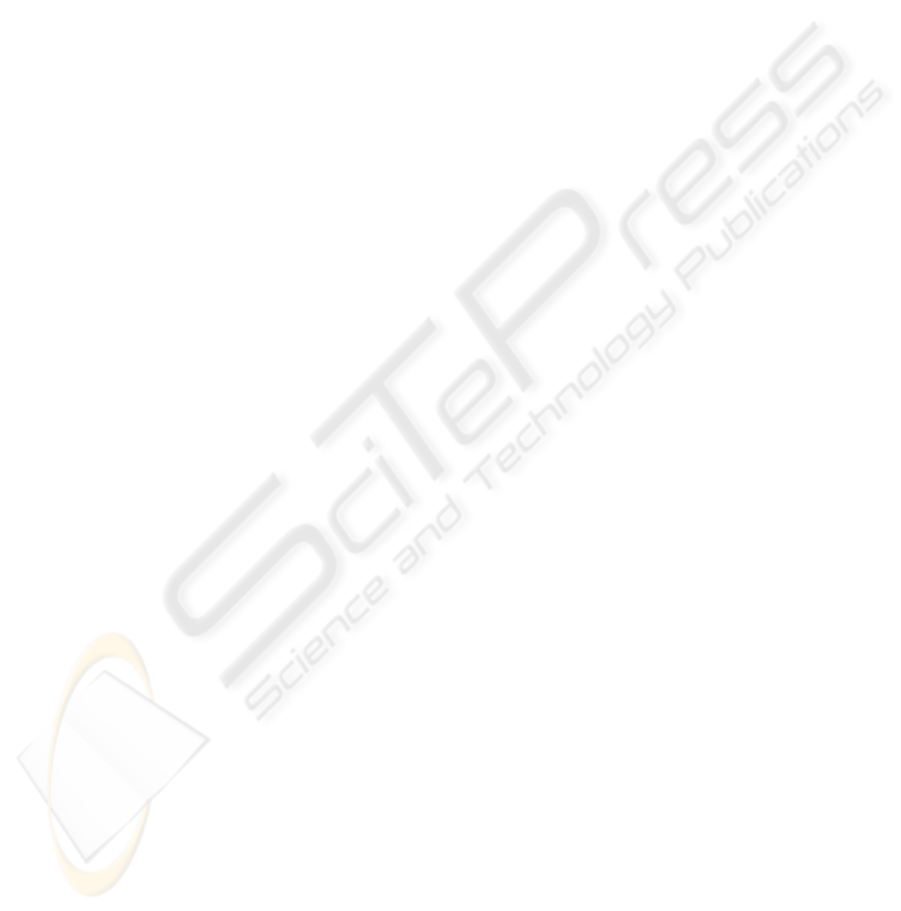
on a 10-word vocabulary of English digits. A good performance could be achieved even
when words were spoken non-audibly, i.e. when no acoustic signal was produced [3],
suggesting this technology could be used to communicate silently. While the former ap-
proaches used words as model units, [4] successfully demonstrated that phonemes can
be used as modeling units for EMG-based speech recognition, paving the way for large
vocabulary continuous speech recognition. Recent results include advances in acoustic
modeling using a clustering scheme on phonetic features, which represent properties of
a given phoneme, such as the place or the manner of articulation. In [5], we report that
a recognizer based on such bundled phonetic features performs more than 30% better
than a recognizer based on phoneme models only.
While reliable automatic recognition of silent speech is currently heavily investi-
gated and recent performance results come within useful reach, little is known about
the EMG signal variations resulting from differences in human articulation between au-
dible and silent speech production. Therefore, this paper studies the variations in the
EMG signal caused by speaking modes. We distinguish audible EMG, i.e. EMG signals
recorded on normally pronounced speech, whispered EMG, i.e. EMG signals recorded
on whispered speech, and silent EMG, i.e. signals from silently mouthed speech.
Maier-Hein [6] was the first to investigate cross-modal speech recognition perfor-
mance, i.e. models were trained on EMG signals from audible speech and tested on
EMG signals from silent speech, and vice versa. The results suggested that the EMG
signals are impacted by the speaking mode. Also, it was found that performance differ-
ences were lower for those speakers who had more practice in speaking silently while
using the system.
Since the capability to recognize silent speech is the focus of Silent Speech Inter-
faces in general, and EMG-based speech recognition in particular, we consider it very
crucial to investigating how the difference between speaking audibly or silently affects
the articulation and the measured EMG signal. Furthermore, it is of very high interest
to the silent speech research community how to compensate for these differences for
the purpose of speech recognition.
In [7] we performed first experiments on cross-modal recognition of continuous
speech based on units which were smaller than words. We showed that the difference
between audible and silent speaking modes has a significant negative impact on recog-
nition performance. We also conducted preliminary experiments on comparing the dif-
ferences between recordings of audible and silent EMG and postulated a correlation be-
tween signal energy levels and cross-modal recognition performance. The current study
is a continuation of these initial experiments. Here, we investigate the spectral content
of the EMG signals of audible, whispered and silent speech, showing that there is a
correlation between similar spectral contents and good recognition performance across
different speaking modes. We then present a spectral mapping method which serves
to reduce the discrepancies between spectral contents in different speaking modes. We
perform additional experiments on whispered speech, since this speaking mode can be
seen as an in-between of audible and silent speech: On the one hand, it is generally
softer than audible speech and does not involve any vocal chord vibration, on the other
hand, whispered speech still provides acoustic feedback to the speaker.
23