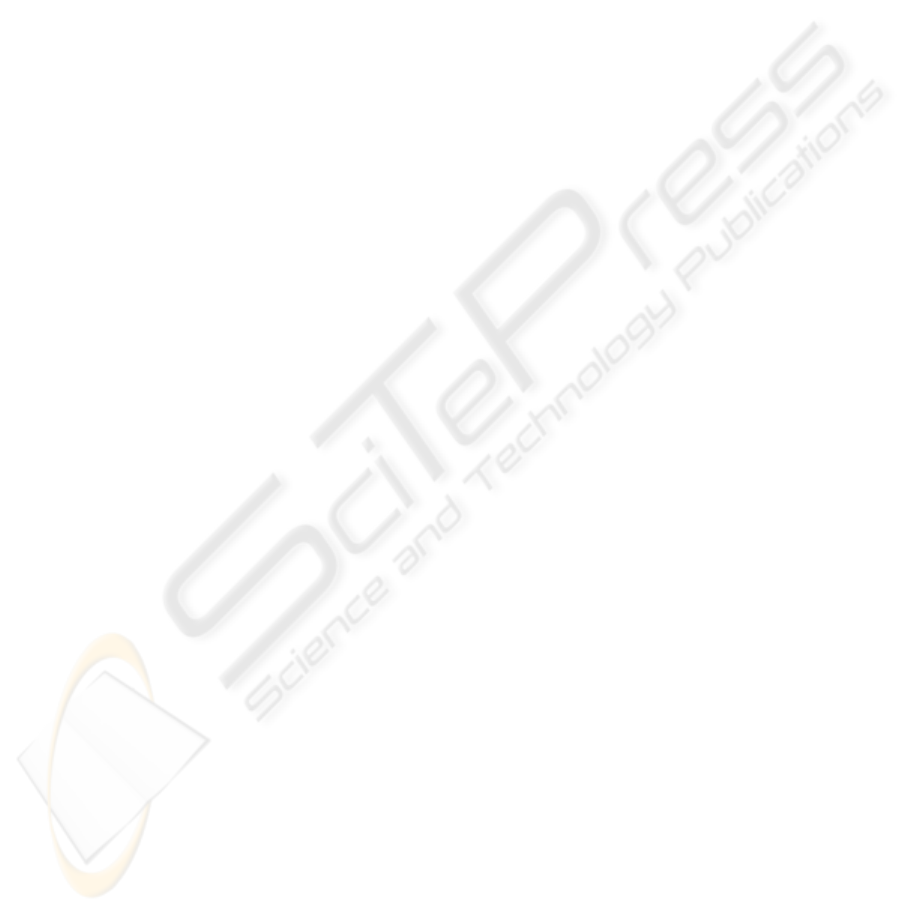
4 SUMMARY AND
CONCLUSIONS
Perceived age estimation is highly useful in various
real-world applications such as developing efficient
marketing strategies. In this paper, we proposed a
novel method for perceived age estimation from face
images by combining two ideas. The first idea was
an efficient active learning strategy for reducing the
cost of labeling face samples. Experiments showed
that our active learning strategy together with man-
ifold regularization can improve the performance of
perceived age estimation even with a relatively small
number of labeled face samples. The second idea was
to take account of heterogeneous characteristics of
human age perception in the form of weighted regres-
sion. Experimental results showed that our weighted
regression method can properly handle heteroscedas-
tic noise and thus the prediction performance is qual-
itatively improved.
We have used characteristics of human age per-
ception as weights—error in younger age brackets is
more serious than that in older age groups. On the
other hand, our framework can accommodate arbi-
trary weights, which opens up new interesting re-
search possibilities. Higher weights lead to better
prediction in the corresponding age brackets, so we
can improve the prediction accuracy of arbitrary age
groups (but the price we have to pay for this is a
performance decrease in other age brackets). This
property could be useful, for example, in cigarettes
and alcohol retail, where accuracy around 20 years
old needs to be enhanced but accuracy in other age
brackets are not so important. Another possible usage
of our weighted regression framework is to combine
learned functions obtained from several different age
weights. This could further improve the age predic-
tion performance, which we would like to pursue in
our future work.
REFERENCES
Duda, R. O., Hart, P. E., and Stor, D. G. (2001). Pattern
Classification. Wiley, New York.
Fu, Y., Xu, Y., and Huang, T. S. (2007). Estimating human
age by manifold analysis of face pictures and regres-
sion on aging features. In Proceedings of the IEEE
Multimedia and Expo. 1383–1386.
Geng, X., Zhou, Z., Zhang, Y., Li, G., and Dai, H. (2006).
Learning from facial aging patterns for automatic age
estimation. In Proceedings of the 14th ACM Interna-
tional Conference on Multimedia, 307–316.
Guo, G., Fu, Y., Dyer, C. R., and Huang, T. S. (2008).
Image-based human age estimation by manifold learn-
ing and locally adjusted robust regression. In IEEE
Transactions on Image Processing, 17(7), 1178–1188.
Hoerl, A. E. and Kennard, R. W. (1970). Ridge regres-
sion: biased estimation for nonorthogonal problems.
In Technometrics, 12:55–67, 1970.
Horng, W. B., Lee, C. P., and Chen, C. W. (2001). Clas-
sification of age groups based on facial features.
In Tamkang Journal of Science and Engineering,
4(3):183–192.
Kwon, Y. H. and Lobo, N. V. (1999). Age classification
from facial images. In Computer Vision and Image
Understanding, 74(1):1–21.
Lanitis, A., Draganova, C., and Christodoulou, C. (2004).
Comparing different classifiers for automatic age esti-
mation. In IEEE Transactions on Systems, Man, and
Cybernetics Part B, 34(1):621–628.
Lanitis, A., Taylor, C. J., and Cootes, T. F. (2002). To-
ward automatic simulation of aging effects on face im-
ages. In IEEE Transactions on Pattern Analysis and
Machine Intelligence, 24(4):442–455.
Phillips, P. J., Flynn, P. J., Scruggs, W. T., Bowyer, K. W.,
Chang, J., Hoffman, K., Marques, J., Min, J., and
Worek, W. J. (2005). Overview of the face recognition
grand challenge. In Proceedings of the IEEE Com-
puter Society Conference on Computer Vision and
Pattern Recognition (CVPR 2005). 947–954.
Ricanek, K. J. and Tesafaye, T. (2006). Morph: A longitu-
dinal image database of normal adult age-progression.
In Proceedings of the IEEE 7th International Con-
ference on Automatic Face and Gesture Recognition
(FGR ’06), 341–345.
Sindhwani, V., Belkin, M., and Niyogi, P. (2006). The ge-
ometric basis of semi-supervised learning. In Semi-
Supervised Learning, The MIT Press, 2006.
Tivive, F. H. C. and Bouzerdoum, A. (2006). A shunting in-
hibitory convolutional neural network for gender clas-
sification. In Proceedings of the 18th International
Conference on Pattern Recognition (ICPR2006),
4:421–424.
Tivive, F. H. C. and Bouzerdoumi, A. (2006). A gender
recognition system using shunting inhibitory convolu-
tional neural networks. In Proceedings of the Interna-
tional Joint Conference on Neural Networks (IJCNN
’06), 5336–5341.
Ueki, K., Miya, M., Ogawa, T., and Kobayashi, T. (2008).
Class distance weighted locality preserving projection
for automatic age estimation. In Proceedings of the
2nd IEEE International Conference on Biometrics:
Theory, Applications and Systems (BTAS 08), 1–5.
VISAPP 2010 - International Conference on Computer Vision Theory and Applications
324