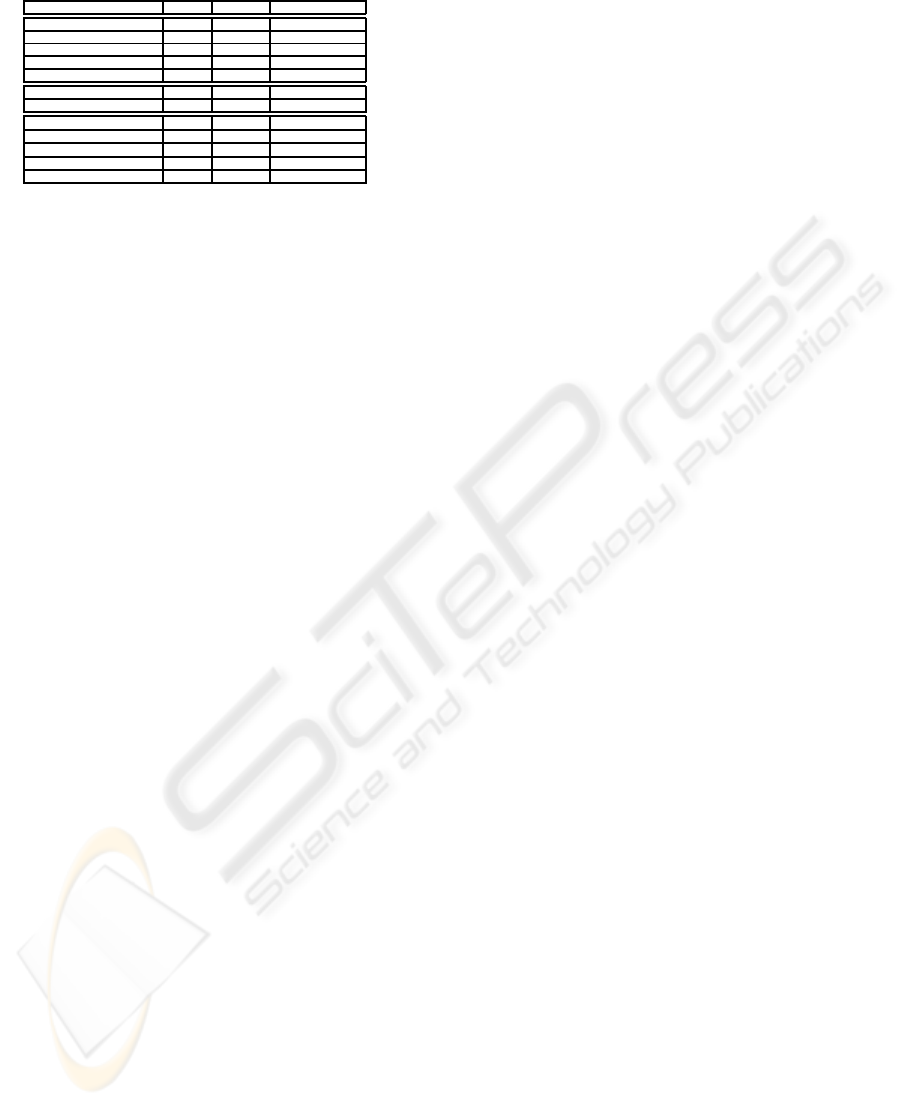
Table 4: Comparison with previous methods.
algorithm spatial classifier recognition rate
CIPHLAC(without VH) relative linear 80.37(± 0.37)
CIPLAS(without VH) relative linear 77.46(± 0.33)
CIPHLAC(with VH) relative linear 80.65(± 0.57)
CIPLAS(with VH) relative linear 82.63(± 0.25)
ALL relative linear 81.64(± 0.53)
SPM(linear)(Baseline) grid linear 72.60(± 0.27)
SP-PHLAC(0th)(Basel.) grid linear 68.29(± 0.22)
(Bosch et al., 2008) grid kernel 83.7
(Wu and Rehg, 2008) grid kernel 83.3(± 0.5)
(Lazebnik et al., 2006) grid kernel 81.4(± 0.5)
(Yang et al., 2009) grid linear 80.28(± 0.93)
(Zheng et al., 2009) grid kernel 74.0
extended to auto/crosscorrelations between posterior
probability images. The autosubtraction operator for
describing another spatial relationship between poste-
rior probability images, and vertical/horizontal mask
patterns for spatial layout of auto/crosscorrelations
are also proposed. Since the combination of cate-
gory index is large, the features are compressed by
2DPCA. Experiments using Scene-15 dataset have
demonstrated that the crosscorrelations between pos-
terior probabilities improves classification perfor-
mances of PHLAC, and auto/crosssubtraction with
vertical/horizontal mask patterns indicated the best
performance in our methods. The classification per-
formances of our methods in Scene-15 dataset were
competitive to the recent proposed methods without
using spatial grid informations and even using linear
classifiers.
REFERENCES
Battiato, S., Farinella, G., Gallo, G., and Ravi, D. (2009).
Spatial hierarchy of textons distributions for scene
classification. In MMM, pages 333–343.
Bosch, A., Munoz, X., and Freixenet, J. (2007). Segmenta-
tion and description of natural outdoor scenes. Image
and Vision Computing, 25:727–740.
Bosch, A., Zisserman, A., and Munoz, X. (2008). Scene
classification using a hybrid generative/discriminative
approach. IEEE Trans. on PAMI, 30(4):712–727.
Fan, R.-E., Chang, K.-W., Hsieh, C.-J., Wang, X.-R., and
Lin, C.-J. (2008). Liblinear: A library for large linear
classification. JMLR, 9:1871–1874.
Farinella, G., Battiato, S., Gallo, G., and Cipolla, R. (2008).
Natural versus artificial scene classification by order-
ing discrete fourier power spectra. In SSPR&SPR.
FeiFei, L. and Perona, P. (2005). A bayesian hierarchical
model for learning natural scene categories. In CVPR.
Gorkani, M. and Picard, R. (1994). Texture orientation for
sorting phots at a glance. In ICPR.
Hotta, K. (2009). Scene classification based on local auto-
correlation of similarities with subspaces. In ICIP.
Kobayashi, T. and Otsu, N. (2008). Image feature extraction
using gradient local auto-correlations. In ECCV.
Ladret, P. and Gue’rin-Dugue’, A. (2001). Categorisation
and retrieval of scene photographs from a jpeg com-
pressed database. Pattern Analysis & Applications,
4:185 – 199.
Lazebnik, S., Schmid, C., and Ponece, J. (2006). Beyond
bags of features: Spatial pyramid matching for recog-
nizing natural scene categories. In CVPR.
Matsukawa, T. and Kurita, T. (2009). Image classification
using probability higher-order local auto-correlations.
In ACCV.
Oliva, A. and Torralba, A. (2001). Modeling the shape of
the scene: A holistic representation of the spatial en-
velope. IJCV, 42(3):145–175.
Otsu, N. and Kurita, T. (1988). A new scheme for prac-
tical flexible and intelligent vision systems. In IAPR
Workshop on Computer Vision, pages 431–435.
Quattoni, A. and Torralba, A. (2009). Recognizing indoor
scenes. In CVPR, pages 413–420.
Rasiwasia, N. and Vasconcelos, N. (2008). Scene classifica-
tion with low-dimensional semantic spaces and weak
supervision. In CVPR, pages 1 – 8.
Renninger, L. and Malik, J. (2004). When is scene iden-
tification just texture recognition? Vision Research,
44(19):2301–2311.
Shotton, J., Johnson, M., and Cipolla, R. (2008). Semantic
texton forests for image categorization and segmenta-
tion. In CVPR, pages 1 – 8.
Szummer, M. and Picard, R. (1998). Indoor-outdoor im-
age classification. In IEEE Intl. Workshop on Content-
Based Access of Image and Video Databases.
Torralba, A. (2003). Contextual priming for object detec-
tion. IJCV, 53:169 – 191.
Torralba, A. and Oliva, A. (2003). Statistics of natural im-
age categories. Network: Computing in Nueral Sys-
tems, 14:391 – 412.
Toyoda, T. and Hasegawa, O. (2007). Extension of higher
order local autocorrelation features. Pattern Recogni-
tion, 40:1466–1477.
Vogel, J. and Schiele, B. (2007). Semantic modeling of nat-
ural scenes for content-based image retrieval. IJCV,
72(2):133–157.
Wu, J. and Rehg, J. (2008). Where am i: Place instance and
category recognition using spatial pact. In CVPR.
Yang, J., Yu, K., Gong, Y., and Huang, T. (2009). Linear
spatial pyramid matching using sparse coding for im-
age classification. In CVPR.
Yang, J., Zhang, D., Frangi, A., and Yang, J. (2004). Two-
dimensional pca: a new approach to appearance-based
face representation and recognition. IEEE Trans. on
PAMI, 26(1):131–137.
Yao, B., Niebles, J., and Fei-Fei, L. (2009). Mining discrim-
inative adjectives and prepositions for natural scene
recognition. In The joint VCL-ViSU 2009 workshop.
Zheng, Y., Lu, H., Jin, C., and Xue, X. (2009). Incorporat-
ing spatial correlogram into bag-of-features model for
scene categorization. In ACCV.
Zheng, Y.-T., Zhao, M., Neo, S.-Y., Chua, T.-S., and Tian,
Q. (2008). Visual synset: towards a higher-level visual
representation. In CVPR, pages 1 – 8.
VISAPP 2010 - International Conference on Computer Vision Theory and Applications
332