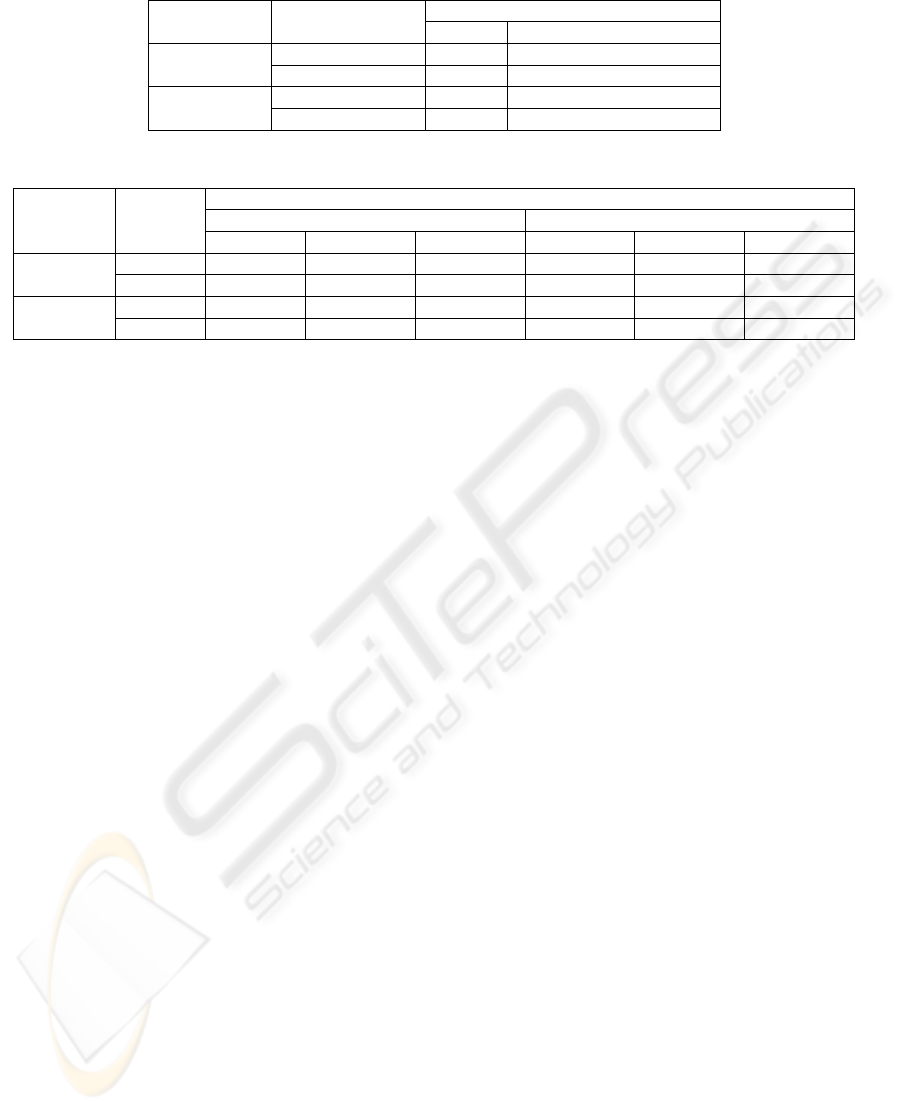
Table 2: Comparison of registration methods.
DataSet Range
Success Rate (%)
Our Uniform sub-sampling
BrainWeb
[-15, 15] 98 96
[-20, 20] 84 86
RREP
[-10, 10] 100 70
[-15, 15] 72 58
Table 3: Comparison of means and standard deviations of registration errors.
DataSet Range
Mean and Standard deviation
Our Uniform sub-sampling based
Tx Ty Rz Tx Ty Rz
BrainWeb
[-15, 15] 0.27±0.17 0.21±0.16 0.20±0.17 0.25±0.17 0.16±0.11 0.21±0.14
[-20, 20] 0.21±0.14 0.19±0.15 0.20±0.14 0.27±0.17 0.16±0.14 0.23±0.17
RREP
[-10, 10] 0.66±0.43 0.58±0.43 0.66±0.32 1.03±0.56 0.59±0.43 0.51±0.45
[-15, 15] 0.93±0.57 0.79±0.58 0.69±0.34 0.93±0.54 0.58±0.49 0.47±0.36
clearly that the proposed method outperformed
traditional uniform sub-sampling based method.
For those successful cases of registration, mean
and standard deviations of rotation errors and
translation errors were calculated and summarized in
Table 3. We can observe that the accuracy of our
proposed method is comparable to that of uniform
sub-sampling based method.
4 CONCLUSIONS
In this paper, we have presented a novel method of
constructing minimal spanning tree for multi-
modality image registration. The new method
hierarchically fuses multi-salient points to construct
MST. This new method integrates not only more
information obtained from multi-salient points to
improve robustness of image registration, but also
hierarchical mechanism to produce relatively
accurate registration results. Experiment results
show that on the simulated and real brain datasets,
the algorithm achieves better robustness while
maintaining good registration accuracy.
REFERENCES
Beirlant, J., Dudewicz, E. J., Gyorfi, L., and van der
Meulen, E.C. (1997). Nonparametric entropy
estimation: An overview. International Journal of
Mathematical and Statistical Sciences, 6(1):17–39.
Collins,D.L., Zijdenbos,A.P., Kollokian,V., Sled, J.G.,
Kabani, N.L., Holmes, C.J., and Evans, A.C. (1998).
Design and construction of a realistic digital brain
phantom. IEEE Trans. Med. Imag., 17:463-468.
Harris, C., Stephens, M. (1988). A Combined Corner and
Edge Detector. In Alvey Vision Conference, pages
147-151.
Hero, A., Ma, B., Michel, O., and Gorman, J. (2002).
Applications of entropic spanning graphs. IEEE Signal
Processing Magazine, 19(5):85–95.
Ma, B., Hero, A., Gorman, J., and Michel, O. (2000).
Image registration with minimal spanning tree
algorithm. In ICIP.
Miller, E. (2003). A new class of entropy estimators for
mult-dimensional densities. In Proc. ICASSP.
Redmond, C., Yukich, J. (1996). Asymptotics for
Euclidean functionals with power weighted edges.
Stochastic Processes and their Applications,
61(2):289-304.
Sabuncu, M. R., Ramadge, P.J. (2004). Gradient based
nonuniform subsampling for information-theoretic
alignment methods. In EMBC.
Sabuncu, M. R., Ramadge, P. J. (2008). Using Spanning
Graphs for Efficient Image Registration. IEEE
Transactions on Image Processing, 17(5):788-797.
Shen, D., Davatzikos, C. (2002). HAMMER: hierarchical
attribute matching mechanism for elastic registration.
IEEE Trans. Med. Imaging, 21(11): 1421–1439.
Steele, J. M. (1988). Growth rate of Euclidean minimal
spanning trees with power weighted edges. Ann.
Probab., pages 1767–1787.
West, J., Fitzpatrick, J.M., Wang, M.Y., Dawant, B.M.,
Maurer Jr., C.R., Kessler, R.M., Maciunas, R.J.,
Barillot, C., Lemoine, D., Collignon, A., Maes, F.,
Suetens, P., Vandermeulen, D., van den Elsen, P.A.,
Napel, S., Sumanaweera, T.S., Harkness, B., Hemler,
P.F., Hill, D.L.G., Hawkes, D.J., Studholme, C.,
Maintz, J.B., Viergever, M.A., Malandain, G., and
Woods, R.P. (1997). Comparison and evaluation of
retrospective intermodality brain image registration
techniques. J. Comput. Assist. Tomogr, (4):554–566.
Yang, G., Stewart, C., Sofka, M., Tsai C. (2007).
Registration of challenging image pairs: initialization,
estimation, and decision. IEEE Trans. Pattern Anal.
Mach. Intell., 29(11):1973-1989.
VISAPP 2010 - International Conference on Computer Vision Theory and Applications
36