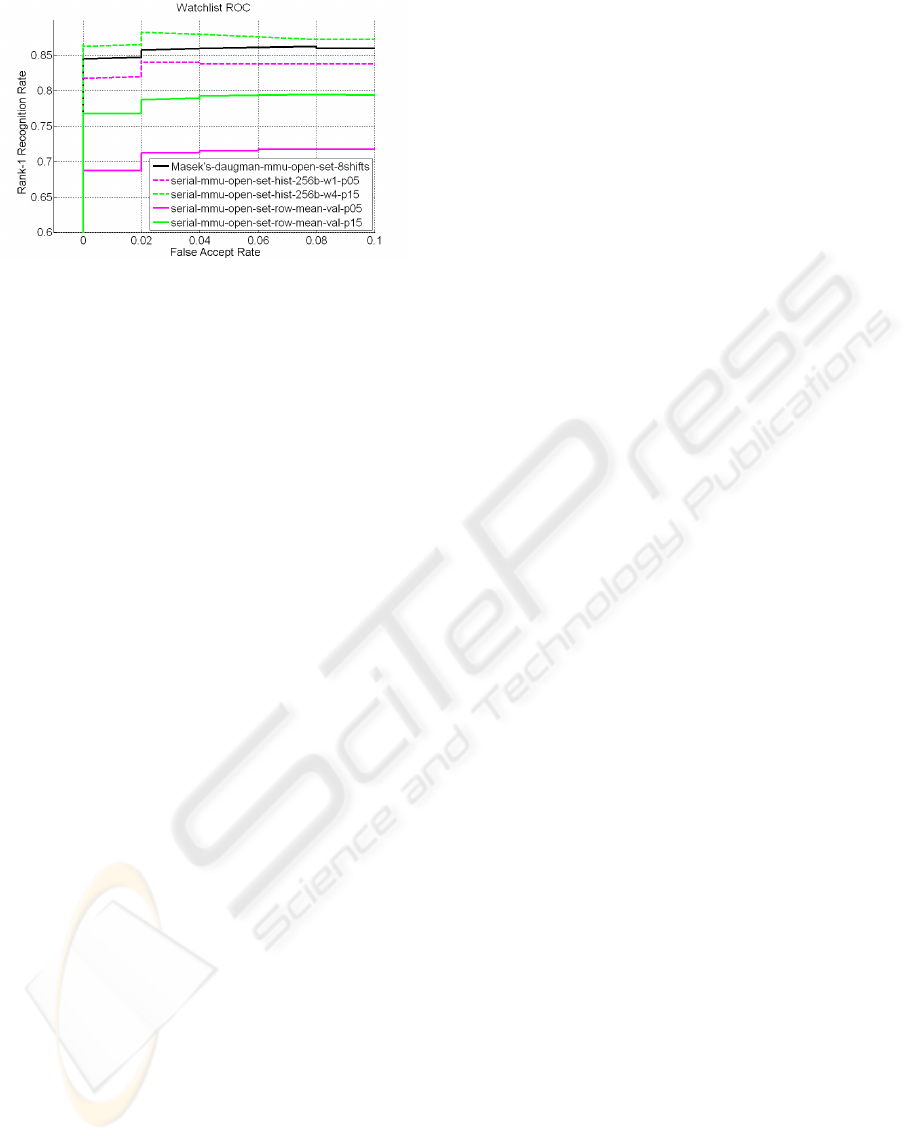
Figure 4: Performance of the 2D serial approach compared
to the iris code approach for the MMU dataset.
V3.0), the 2D variant (hist-256b-w1) improves per-
formance compared to 1D signatures considerably,
while weighting (hist-256b-w4) only results in minor
improvement. The performance of the serial approach
with 2D signatures is better as the iris code technique
for all cases for both CASIA datasets.
Figure 4 shows that also for the MMU dataset
improvements in terms of recognition performance
as compared to the iris-code approach are possible
if 2D signatures are used and p is set sufficiently
high. We observe that for p = 15 and 2D signa-
tures with weighting the iris code approach is outper-
formed across the entire range of considered false ac-
cept rates. Note that this improvement still is achieved
at a reduction of computational effort by a factor of
3.5 (compare Table 2).
4 CONCLUSIONS
As expected, we are able to reduce computational de-
mands with our proposed serial classifier combination
considerably. At a comparable recognition accuracy
we suffice with 20% - 30% or even less computa-
tion time for identification (the actual value depends
on the specific dataset considered). Interestingly, we
are even able to outperform the recognition accuracy
of iris code based recognition, since the serial clas-
sifier combination technique turns out to be more ro-
bust against false acceptances. This is especially in-
teresting, since we reveal that the rotation-invariant
first stage of the serial combination is able to exclude
candidates, which lead to false accepts in the entirely
rotation-compensating iris-code approach.
ACKNOWLEDGEMENTS
This work has been partially supported by the Aus-
trian Research Promotion Agency (FFG) FIT-IT Trust
in IT-Systems project 819382.
REFERENCES
Daugman, J. (2004). How iris recognition works. IEEE
Transactions on Circiuts and Systems for Video Tech-
nology, 14(1):21–30.
Du, Y., Ives, R., Etter, D., and Welch, T. (2006). Use
of one-dimensional iris signatures to rank iris pattern
similarities. Optical Engineering, 45(3):037201–1 –
037201–10.
Gentile, J. E., Ratha, N., and Connell, J. (2009). An effi-
cient, two-stage iris recognition system. In Biomet-
rics: Theory, Applications and Systems (BTAS’09),
pages 1–5.
Matschitsch, S., St
¨
ogner, H., Tschinder, M., and Uhl, A.
(2008). Rotation-invariant iris recognition: boosting
1D spatial-domain signatures to 2D. In Filipe, J.,
Cetto, J., and Ferrier, J.-L., editors, ICINCO 2008:
Proceedings of the 5th International Conference on
Informatics in Control, Automation and Robotics, vol-
ume SPSMC, pages 232–235. INSTICC Press.
Park, C.-H. and Lee, J.-J. (2006). Extracting and com-
bining multimodal iris features. In Proceedings of
the 1st IAPR International Conference on Biometrics
(ICB’06), number 3832 in Lecture Notes on Computer
Science, pages 389–396.
Qui, X., Sun, Z., and Tan, T. (2007). Coarse iris classifi-
cation by learned visual dictionary. In Proceedings of
the 2nd IAPR/IEEE International Conference on Bio-
metrics (ICB’07), number 4642 in Lecture Notes on
Computer Science, pages 770–779.
Ross, A., Nandakumar, K., and Jain, A. (2006). Handbook
of Multibiometrics. Springer.
Sun, Z., Wang, Y., Tan, T., and Cui, J. (2005). Improv-
ing iris recognition accuracy via cascaded classifiers.
IEEE Transactions on Systems, Man and Cybernetics,
35(3):435–441.
Uhl, A. and Wild, P. (2009). Parallel versus serial classifier
combination for multibiometric hand-based identifica-
tion. In Tistarelli, M. and Nixon, M., editors, Pro-
ceedings of the 3rd International Conference on Bio-
metrics 2009 (ICB’09), volume 5558 of LNCS, pages
950–959. Springer Verlag.
Vatsa, M., Singh, R., and Noore, A. (2005). Reducing the
false rejection rate of iris recognition using textural
and topological features. Int. Journal of Signal Pro-
cessing, 2(2):66–72.
Yu, L., Wang, K., and Zhang, D. (2006). A novel
method for coarse iris classification. In Proceedings of
the 1st IAPR International Conference on Biometrics
(ICB’06), number 3832 in Lecture Notes on Computer
Science, pages 404–410.
Zhang, P.-F., Li, D.-S., and Wang, Q. (2004). A novel iris
recognition method based on feature fusion. In Pro-
ceedings of the International Conference on Machine
Learning and Cybernetics, pages 3661–3665.
VISAPP 2010 - International Conference on Computer Vision Theory and Applications
90