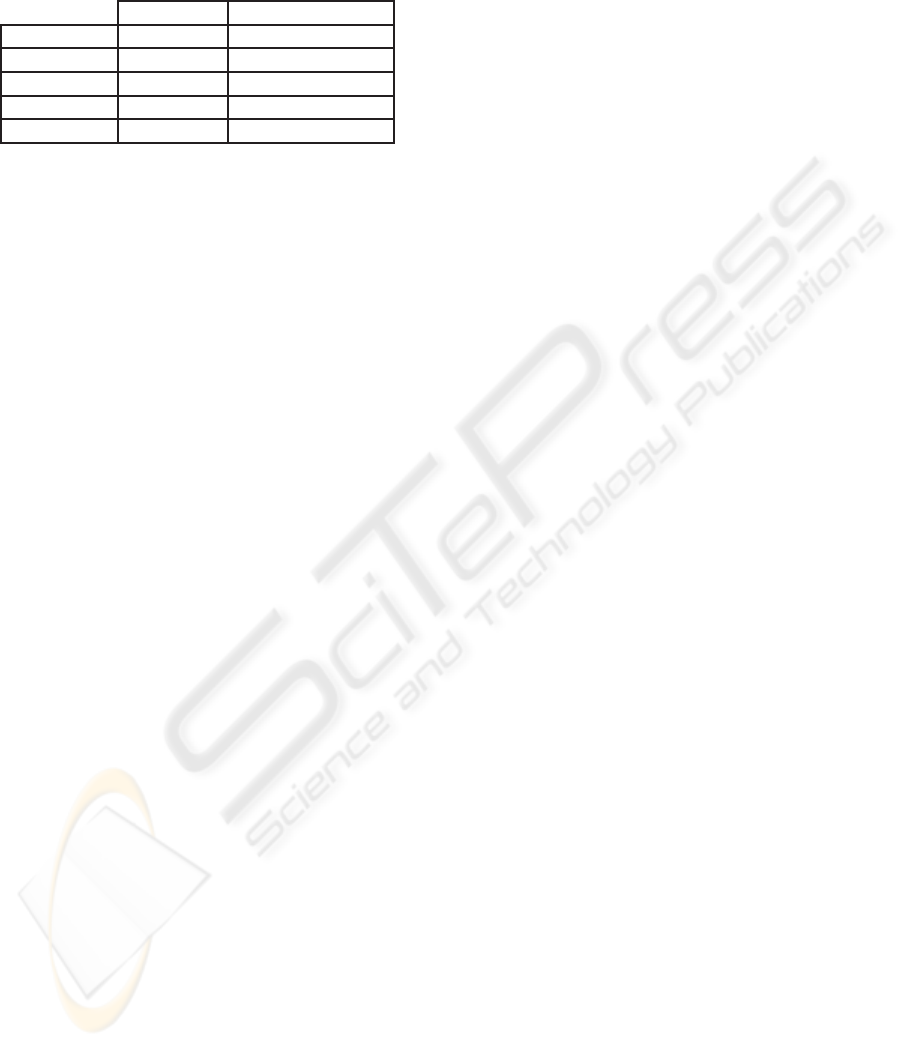
Table 2: DICE of pre-treated images: the three numbers
show the average values: with the 10 images (with the 4
images acquired in static way, with the 6 images acquired
in a dynamic manner).
AFMM MRDH, C
Tr
= 50
None 46 (49,44) 55 (52,57)
Threshold 64 (68,61) 55 (60,50)
Smoothing 66 (77,59) 52 (63,42)
Erosion 59 (58,60) 57 (64,51)
Restoration 52 (49,55) 57 (59,55)
When pre-processed with the threshold, smooth-
ing, erosion and restoration, the AFMM’s algorithm
is better, with the best results being obtained after a
smoothing. Also, smoothing and threshold have an
even more beneficial effect when static images are
used.
According to the DICE measure, the perfor-
mances of MRDH applied after pre-treatment are
quite disparate. In fact, it illustrates how this new
method is globally quite robust against the acquisition
conditions. Images do not need to be pre-processed in
order to increase the performances and this is an im-
portant superiority compared to the AFMM method
which is highly dependent on the acquisition condi-
tions. Analyzed in details, we notice that for static
images, DICE values seem to be enhanced by each
pre-processing, while DICE values seem to decrease
for "dynamic" images.
6 CONCLUSIONS
A methodology for adapting a multi-resolution-based
segmentation to the field of road crack detection has
been introduced. The experimental results demon-
strate the efficient results obtained on 14 synthetic
images and 10 significant real images. Moreover, it
shows how it outperforms a previous method based
on adapted filtering, in particular with the most dif-
ficult images acquired dynamically and that present
illumination defaults.
The next step of this work will be to validate this
work on more data (32 more images will be avail-
able soon). It has been shown that MRDH improves
the percentage of true detections (this percentage is
higher than with AFMM), but the AFMM presents
less false negatives. The next improvement will be
to reduce this phenomenon by introducing more con-
straints on the final step, like for example, an active
contour constraint instead of using only the constraint
on the contrast.
REFERENCES
Bray, J., Verma, B., Li, X., and He, W. (2006). A neural
nework based technique for automatic classification
of road cracks. In International Joint Conference on
Neural Networks, pages 907–912.
Chambon, S., Gourraud, C., Moliard, J.-M., and Nicolle, P.
(2010). Road crack extraction with adapted filtering
and markov model-based segmentation. In VISAPP.
Coudray, N., Buessler, J.-L., Kihl, H., and Urban, J.-P.
(2007). Tem images of membranes: a multiresolu-
tion edge-detection approach for watershed segmenta-
tion. In Physics in Signal and Image Processing (PSIP
2007).
Elbehiery, H., Hefnawy, A., and Elewa, M. (2005). Sur-
face defects detection for ceramic tiles using image
processing and morphological techniques. Proceed-
ings of World Academy of Science, Engineering and
Technology (PWASET), 5:158–162.
Frangi, A. F., Niessen, W. J., Vincken, K. L., and Viergever,
M. A. (1998). Muliscale vessel enhancement filtering.
In MICCAI, pages 130–137.
Geman, D. and Jedynak, B. (1996). An active testing model
for tracking roads in satellite images. IEEE Pattern
Analysis and Machine Intelligence, 18(1):1–14.
Iyer, S. and Sinha, S. (2005). A robust approach for auto-
matic detection and segmentation of cracks in under-
ground pipeline images. Image and Vision Computing,
23(10):921–933.
Koutsopoulos, H. and Downey, A. (1993). Primitive-based
classification of pavement cracking images. ASCE,
Journal of Transportation Engineering, 119(3):402–
418.
Redding, N. (1996). The autoscaling of oblique ionograms.
Technical report, Defence science and technology or-
ganization canberra.
Schmidt, B. (2003). Automated pavement cracking assess-
ment equipment – state of the art. Technical Report
320, Surface Characteristics Technical Committee of
the World Road Association (PIARC).
Subirats, P., Fabre, O., Dumoulin, J., Legeay, V., and Barba,
D. (2006). Automation of pavement surface crack de-
tection with a matched filtering to define the mother
wavelet function used. In European Signal Process-
ing Conference.
Tanaka, N. and Uematsu, K. (1998). A crack detection
method in road surface images using morphology.
In Workshop on Machine Vision Applications, pages
154–157.
MULTI-RESOLUTION APPROACH FOR FINE STRUCTURE EXTRACTION - Application and Validation on Road
Images
147