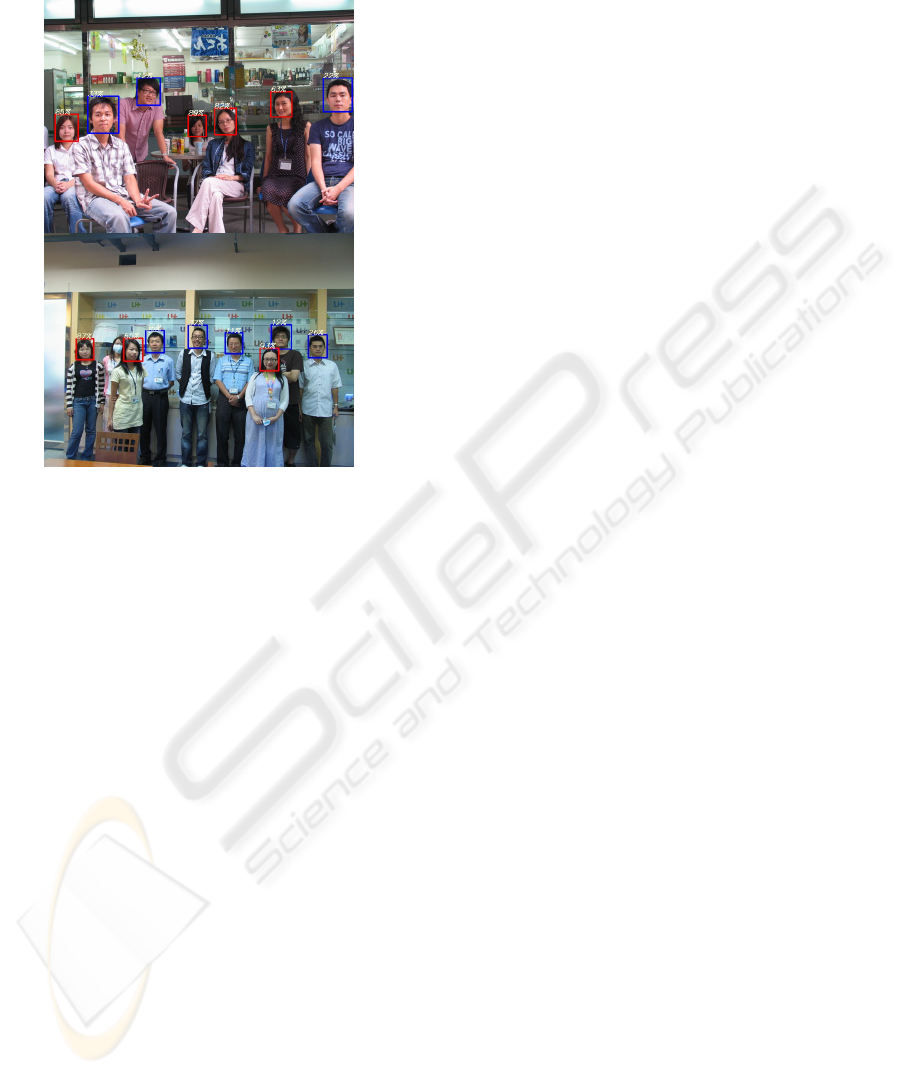
dataset have shown that our system is effective and
efficient in recognizing genders in uncontrolled real
life images.
Figure 3: Demonstration of the recognizing results.
ACKNOWLEDGEMENTS
This work is supported by the National Science
Council under Contract No. NSC98-2218-E-155-001.
REFERENCES
Wang, Y., Ai, H., and Wu, B., Huang, C., 2004. Real
Time Facial Expression Recognition with Adaboost.
ICPR’04, International Conference on Pattern
Recognition.
Andreu, Y., Mollineda, R.A., and Garc´ıa-Sevilla, P.,
2009. Gender Recognition from a Partial View of the
Face Using Local Feature Vectors. Lecture Notes in
Computer Science.
Gallagher, A.C. and Chen, T., 2009. Understanding
Images of Groups of People. CVPR’09, IEEE
Conference on Computer Vision and Pattern
Recognition.
Cao, L., Dikmen, M., Fu, Y., and Huang, T.S., 2008.
Gender Recognition from Body. ACM international
conference on Multimedia.
Shen, B.C., Chen, C.S., and Hsu, H.H., 2009. Fast Gender
Recognition by Using A shared-Integral-Image
Approach. ICASSP’09, IEEE International
Conference on Acoustics, Speech and Signal
Processing.
Lu, H. and Lin, H., 2007. Gender Recognition using
Adaboosted Feature. International Conference on
Natural Computation.
Lin, H., Lu, H., and Zhang, L., 2006. A New Automatic
Recognition System of Gender, Age and Ethnicity.
The Sixth World Congress on Intelligent Control and
Automation.
Balci, K. and Atalay, V., 2002. PCA for gender
estimation: which eigenvectors contribute? ICPR’02,
International Conference on Pattern Recognition.
Rodrigo, V., Javier, R. D. S., and Mauricio, C., 2006.
Gender Classification of Faces Using Adaboost.
CIARP’06.
Fang, Y. and Wang, Z., 2008. Improving LBP Features for
Gender Classification. International Conference on
Wavelet Analysis and Pattern Recognition.
Lian, H.C. and Lu, B.L., 2007. Multi-View Gender
Classification Using Multi-Resolution Local Binary
Patterns and Support Vector Machines. International
Journal of Neural Systems.
Wu, B., Ai, H. and Huang, C., 2003. LUT-Based
Adaboost for Gender Classification. Audio- and
Video-Based Biometric Person Authentication.
Wu, B., Ai, H. and Huang, C., 2004. Facial image retrieval
based on demographic classification. ICPR’04,
International Conference on Pattern Recognition.
Lu, H., Huang, Y., Chen, Y. and Yang, D., 2003.
Automatic gender recognition based on pixel-pattern-
based texture feature. Journal of Real-Time Image
Processing.
Nikolaus, R., 2007. Learning the Parts of Objects using
Non-negative Matrix Factorization. Term Paper, Feb
2007.
Lee, D.D. and Seung, H.S., 1999. Learning the parts of
objects with nonnegative matrix factorization. Nature.
Lapedriza, A., Masip, D. and Vitria, J., 2005. Are External
Face Features Useful for Automatic Face
Classification? CVPR’05, Computer Vision and
Pattern Recognition.
Lapedriza, A., Marin-Jimenez, M.J. and Vitria, J., 2006.
Gender Recognition in Non Controlled Environments.
International Conference on Pattern Recognition.
Mayo, M. and Zhang, E., 2008. Improving Face Gender
Classification By Adding Deliberately Misaligned
Faces to The Training Data. Image and Vision
Computing New Zealand.
Osuna, E. and Freund, R., 1997. An Improved training
algorithm for Support Vector Machine. IEEE
Workshop on Neural Networks for Signal Processing.
Moghaddam, B. and Yang, M.H., 2000. Gender
Classification with Support Vector Machines. Int'l
Conf. on Automatic Face and Gesture Recognition.
Freund, Y. and Schapire, R. E., 1996. Experiments
with a New Boosting Algorithm. Machine Learning:
Proceedings of the Thirteenth International
Conferenc.
Schapire, R. E. and Singer, Y., 1999. Improved boosting
algorithms using confidence-rated predictions.
Machine. Learning.
Viola, P. and Jones, M., 2001. Rapid object detection
using a boosted cascade of simple features. IEEE
Conference on Computer Vision and Pattern
Recognition.
VISAPP 2010 - International Conference on Computer Vision Theory and Applications
362