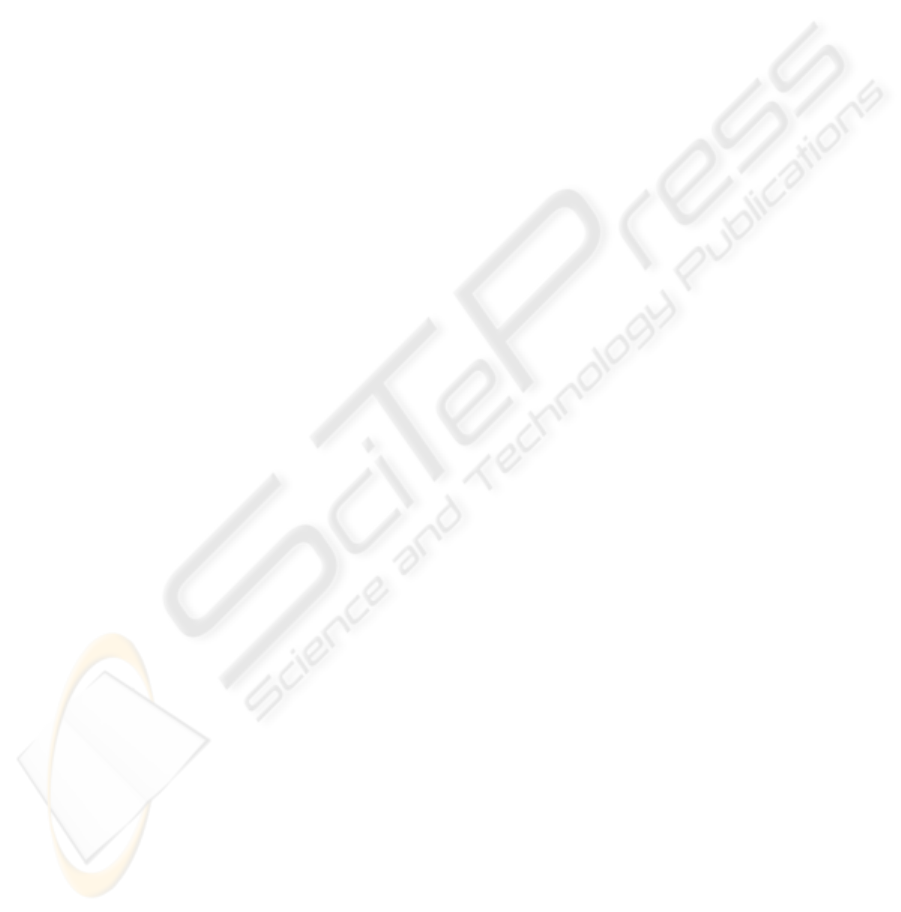
also conducted eye-tracking experiments, in order to
investigate how our method can aggressively direct
the visual attention of the viewers. The original and
visually enhanced versions of a natural scene were
displayed in a random sequence with the resolution
of 800x600 pixels. Subjects (8 males and 2 females)
were asked to freely look at each image for 10 seconds
while their gaze movements were recorded with a To-
bii X120 non-intrusive eye tracker. Figure 6 shows
the comparison of the results, which indicates the fact
that we pay more visual attention to the warning signs
on the top right in the enhanced version of the scene.
Careful analysis of the recorded gaze movements also
suggests that the first gaze fixation on the warning
sizes has been reduced from 6.15s to 4.95s in aver-
age due to the DOF effects, while we count the time
as 10.0s in the case that the subject did not notice the
warning signs.
5 CONCLUSIONS
In this paper, we have first introduced the definition
of the importance map, which represents the percep-
tual importance of a visual scene by extraction and
combination of low-level features. Based on this im-
portance map, we enhance the salient features in the
input scene by applying semantic depth of field effects
to naturally guide the visual attention of the viewers.
The whole pipeline can be executed in real time with-
out any user-intervention, and the experiment results
suggest that our method can actually assist people in
rapidly finding significant features in the scene.
ACKNOWLEDGEMENTS
We would like to thank Radhakrishna Achanta et al.
for sharing their implementation results of previous
researches, and anonymous reviewers for their valu-
able comments. This work has been partially sup-
ported by Japan Society of the Promotion of Sci-
ence under Grants-in-Aid for Scientific Research (B)
No. 20300033 and No. 21300033.
REFERENCES
Achanta, R., Hemami, S., Estrada, F., and Susstrunk, S.
(2009). Frequency-tuned Salient Region Detection. In
Proc. IEEE International Conf. Computer Vision and
Pattern Recognition (CVPR2009), pages 1597–1604.
Bruce, N. and Tsotsos, J. (2007). Attention based on infor-
mation maximization. Journal of Vision, 7(9):950.
Gao, D. and Vasconcelos, N. (2007). Bottom-up saliency
is a discriminant process. In Proc. IEEE International
Conf. Computer Vision (ICCV2007), pages 1–6.
Harel, J., Koch, C., and Perona, P. (2006). Graph-based vi-
sual saliency. In Proc. Neural Information Processing
Systems (NIPS2006), pages 570–577.
Hou, X. and Zhang, L. (2007). Saliency detection: A
spectral residual approach. In Proc. IEEE Interna-
tional Conf. Computer Vision and Pattern Recognition
(CVPR2007), pages 1–8.
Itti, L. and Baldi, P. F. (2009). Bayesian surprise attracts
human attention. Vision Research, 49(10):1295–1306.
Itti, L. and Koch, C. (2001). Computational modelling
of visual attention. Nature Reviews Neuroscience,
2(3):194–203.
Itti, L., Koch, C., and Niebur, E. (1998). A model of
saliency-based visual attention for rapid scene anal-
ysis. IEEE Trans. Pattern Analysis and Machine In-
telligence, 20(11):1254–1259.
Koch, C. and Ullman, S. (1985). Shifts in selective visual
attention: towards the underlying neural circuitry. Hu-
man Neurobiology, 4(4):219–227.
Kosara, R., Miksch, S., and Hauser, H. (2001). Semantic
depth of field. In Proc. IEEE Symp. Information Visu-
alization 2001 (INFOVIS2001), pages 97–104.
Kosara, R., Miksch, S., and Hauser, H. (2002a). Fo-
cus+Context taken literally. IEEE Computer Graphics
and Applications, 22(1):22–29.
Kosara, R., Miksch, S., Hauser, H., Schrammel, J., Giller,
V., and Tscheligi, M. (2002b). Useful properties of
semantic depth of field for better F+C visualization. In
Proc. Symp. Data Visualisation 2002 (VISSYM2002),
pages 205–210.
Ma, Y.-F. and Zhang, H.-J. (2003). Contrast-based image at-
tention analysis by using fuzzy growing. In Proc. 11th
ACM International Conf. Multimedia (MULTIME-
DIA2003), pages 374–381.
Simons, D. J. (2000). Current approaches to change blind-
ness. Visual Cognition, 7:1–15.
Tsotsos, J. (1990). Analyzing vision at the complexity level.
Behavioral and Brain Sciences, 13(3):423–445.
Zhang, L., Tong, M. H., and Cottrell, G. W. (2009). Sunday:
Saliency using natural statistics for dynamic analysis
of scenes. In Proc. 31st Annual Cognitive Science So-
ciety Conf. (CogSci2009), pages 2944–2949.
Zhang, L., Tong, M. H., Marks, T. K., Shan, H., and Cot-
trell, G. W. (2008). SUN: A Bayesian framework for
saliency using natural statistics. Journal of Vision,
8(7):1–20.
REAL-TIME ENHANCEMENT OF IMAGE AND VIDEO SALIENCY USING SEMANTIC DEPTH OF FIELD
375