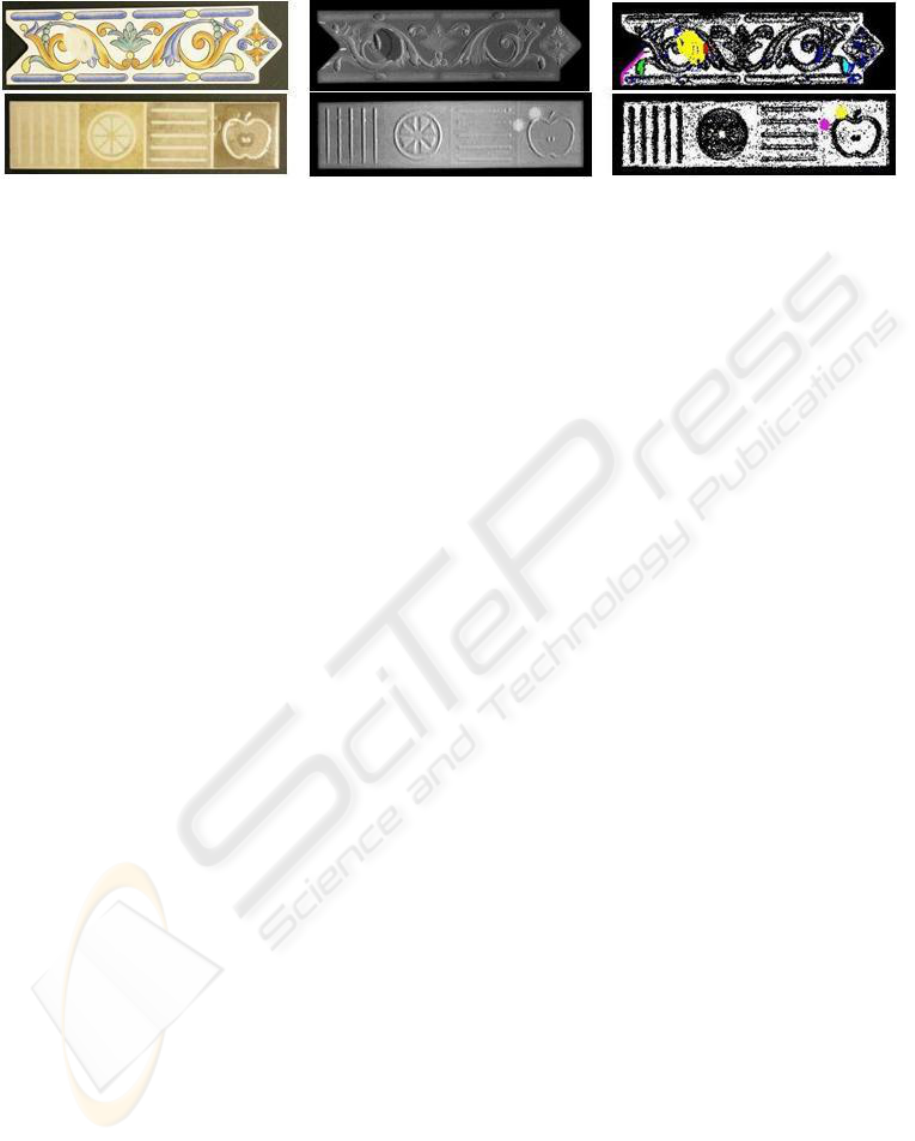
Figure 6: From left to right: Original tiles, Range images and Defect marked on uncertainty image.
atives (TN) and false negatives (FN). This approach
keeps the success rate on expected levels, but de-
creases to 94% when test tiles is rotated. The weight
factor of a defect increases when its position is be-
tween areas of high and low uncertainty, see figure 5.
Our proposed approach add the quantification of
detected defects in contrast to other systems e.g.
(Smith, 2000). We achieved our goal inspecting the
entire surface of tiles and to work with no planar sur-
faces, irregular shapes and glossy or glazed surfaces.
The proposed system has a final resolution of 0.06
mm in the Z direction. This extracts a set of features:
quantity of defective pixels tile, centroid, bounding
box, area, volume, perimeter, confidence measure,
and dispersion each tile defect. Also, it can detect
silk-screened defects because the color affects some
depth measurement. Experiments with commercial
pieces show reconstruction errors caused by the com-
bination of color and varnish.
5 CONCLUSIONS
A system for industrial inspection of ceramic tiles is
presented, showing a sophisticated inspection strat-
egy, with promising results. The proposed algorithm
use range images, to improve small defects detection
so difficult to see for human eyes.
Our algorithm is aimed to characterize 3D de-
fects of range images with novelty detection tasks,
which that only needs the normal samples for train-
ing purposes and usually uses a distance measure and
a threshold for decision making. We tested success-
fully the algorithm in some orientations with test tiles
from different shapes and textures. With novelty de-
tection method the system will have the advantage of
being able to detect new defects that were unknown at
the time of training.
Our future efforts: improve the response of the al-
gorithm about color, shadows, brightness effects and
time response time. This will include a stage that au-
tomatically corrects the false measurements deep in
the 3D reconstruction.
ACKNOWLEDGEMENTS
This work has been supported partially by research
project DPI2007-51166596-C02-01 (VISTAC).
REFERENCES
Boukouvalas, C., Kittler, J., Marik, R., Mirmehdi, M., and
Petrou, M. (1995). Ceramic tile inspection for color
and structural defects. In Proceedings of AMPT95.
pages 390–399.
Boukouvalas, C., Kittler, J., Marik, R., and Petrou, M.
(1999). Color grading of randomly textured ceramic
tiles using color histograms. In IEEE Transactions on
Industry Electronics. pages 219–226.
Boukouvalas, C. and Petrou, M. (2000). Perceptual correc-
tion for color grading of random textures. In Machine,
Vision and Applications. pages 129–136.
Gottesfeld, L. (1992). A survey of image registration tech-
niques. ACM Computing Surveys. pages 327–376.
L
´
opez, F., Acebr
´
on, F., Valiente, J., , and Perez, E. (2001). A
study of registration methods for ceramic tile inspec-
tion purposes. In Proc. of the IX Spanish Symposium
on Pattern Recognition and Image Analysis. pages
145–150.
L
´
opez, F., Valiente, J., Baldrich, R., and Vanrell, M. (2005).
Fast surface grading using color statistics in the CIE
Lab space. In Iberian Conference on Pattern Recogni-
tion and Image Analysis. In LNCS 3523. pages 666–
673.
Markou, M. and Singh, S. (2003). Novelty detection: a
review - part 1: statistical approaches. In Signal Pro-
cessing 83. pages 2481–2497.
Smith, M., S. R. (2000). Automated inspection of textured
ceramic tiles. In Computers in Industry 43. pages 73–
82.
Valiente, J., Acebr
´
on, F., and L
´
opez, F. (1998). An Au-
tomatic Visual Inspection System for Ceramic Tile
Manufacturing Defects. In Proc.. IASTED Int. Conf.
on Signal Processing and Communications. pages
257–260.
Xie, X. (2008). A Review of Recent Advances in Surface
Defect Detection using Texture analysis Techniques.
In Electronic Letters on Computer Vision and Image
Analysis. pages 1–22.
3D INSPECTION SYSTEM IN CERAMIC TILES SURFACES WITH RANGE IMAGES
163