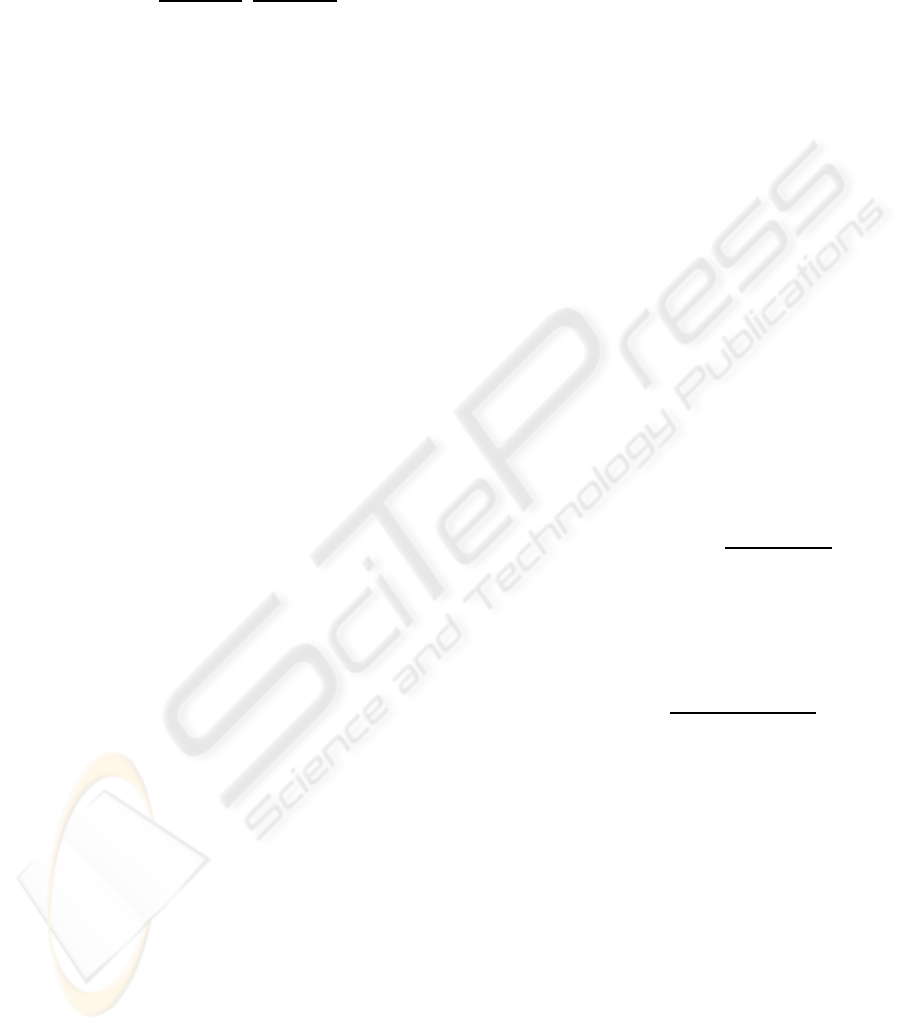
For the sake of clarity we remark its behavior on
the affine part:
P
d,θ
(u,v) = (x(u, v),y(u,v)) (2)
=
uf
vcosθ+ d
,
vf sinθ
vcosθ+ d
3 PROBLEM STATEMENT
Suppose that a certain number of points are uniformly
distributed on some portion of the world plane, and
that we look at them through our pinhole camera,
whose model is described in Section (2). Then, their
spatial distribution is not uniform anymore in the im-
age plane. We assume to have some good algorithm
to detect their position in the picture, and our aim is to
recover the parameters d and θ of the planar homogra-
phy studying such distortion. As stated in Section (2)
the “horizon” is assumed to be parallel to the horizon-
tal axes of the image, but it can be very well outside
the picture. In our framework, the density of points λ
in the world plane (i.e. the number of points per unit
area) needs to be known — it’s a consequence of the
formula (2), where P
ds,θ
(us,vs) = P
d,θ
(u,v) for every
s ∈ R, which means that changing the distance d is
equivalent to change scale in the world plane, and just
looking at the picture we cannot distinguish between
small distance with dense points and big distance with
sparse points.
4 THE ALGORITHM
In order to study the perspective distortion of uni-
formly distributed points, one need to capture the fol-
lowing intuitive notion: points get closer to each other
as approaching to the horizon. What is needed is a
statistical quantity able to discriminate between dif-
ferent perspective projections; our suggestion is to
measure “how much free space” S
p
is present around
each point p of our random configuration. Suppose
that we were able to know S
p
in the world plane for a
given p, and also its transformation, denoted by abuse
of notation P(S
p
); if S
p
was small enough, i.e. if
the points where sufficiently dense, the ratio of ar-
eas |P(S
p
)|/|S
p
| would have been a good estimation
of the determinant of the Jacobian matrix of P at the
point p — the Jacobian determinant measures the fac-
tor with which a function modifies volumes around a
point. And doing this for all the points of the config-
uration, one can have many samples of the Jacobian,
hopefully enough to do a regression and estimate the
parameters of interest d and θ.
But what does “free space around a point” means?
And what is |S
p
|? We don’t have any clue about the
world plane, we just have its perspective view. Again:
what is P(S
p
)? We don’t even know the function P.
A reasonable answer to the first question is given
by the 2-dimensional Voronoi diagram, a tessellation
of the plane generated by a set of points {p
i
} such
that a point q belongs to the cell of p
k
if it’s closer to
p
k
than to any other p
i
; small Voronoi cells mean that
the generating points are “dense”. So for us the free
space around a point is its Voronoi cell. The answer
to the second question is 1/λ, where λ is the density
of points in the world plane. In fact, this is the ex-
pected value for |S
p
|, assuming a Poisson distribution
for the points (Hayen and Quine, 2002); the key point
is its independence from p, which can be intuitively
understood observing that if we take some region A
in the world plane, the expected value of the num-
ber of points inside A is proportional to the area of
A, no matter of its location. In the third question we
ask how to approximate the projection of the Voronoi
cell S
p
; our answer is to compute the Voronoi diagram
generated by the projected points.
Before stating precisely our algorithm we write
the formula of the Jacobian determinant (from now on
just “Jacobian”) of our homography, using the same
notation as in eq. (2)
J
P
(u,v) = det
x
u
x
v
y
u
y
v
=
f
2
d sinθ
(vcosθ+ d)
3
(3)
and point out that since all our measurements are done
in the image plane, while the domain of the above Ja-
cobian is the world plane, what we are going to sam-
ple is the composition
(J
P
◦P
−1
)(x,y) =
( f sinθ−ycosθ)
3
f(d sinθ)
2
(4)
= (a+ yb)
3
At this point, the natural choice to recover the pa-
rameter a and b, hence d and θ, would be to set the
linear regression model
λ|P(S
p
)| = (a+ by)
3
+ ε (5)
where the zero mean noise is taken into account by
the random variable ε. Unfortunately, the variance of
ε varies with the location of the cell S
p
; furthermore,
it is reasonable to assume that such variance is trans-
formed under perspective likewise areas, i.e.
Var(ε) = (λ(a+ by)
3
)
2
Var(S
p
) (6)
This means that knowing Var(ε) is equivalent to know
the parameters a and b that we’re about to estimate.
Any heuristic we could use to estimate the variances
of the errors ε will result in a poor fitting to the 3rd
TWO DOF CAMERA POSE ESTIMATION WITH A PLANAR STOCHASTIC REFERENCE GRID
181