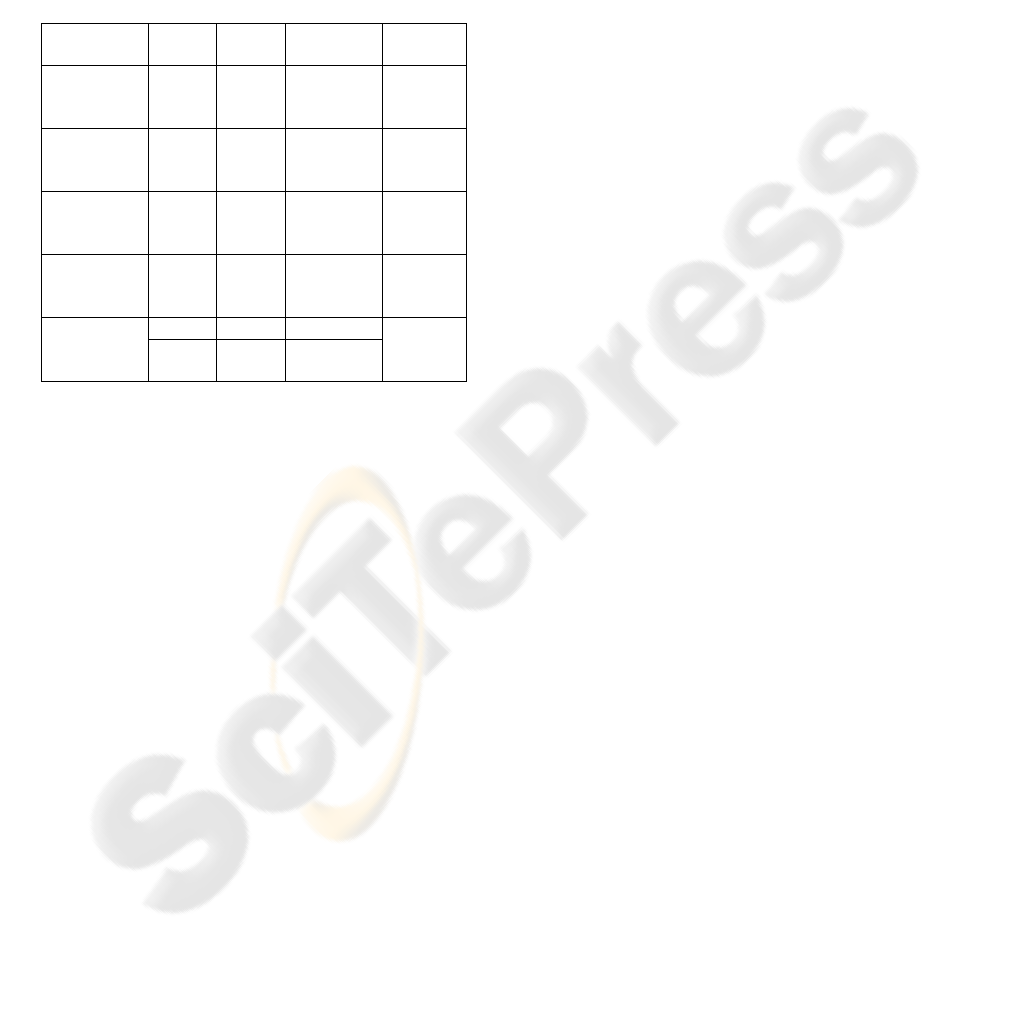
given by our combination of features. But, despite
acceptable results found by our proposed features,
we can not conclude that we have the best results
because we did not use the same database used by
other works. In fact, the digitization can reflect the
final result. Also, the other works use an automatic
system for detection of masses whereas in our work
the task of mass detection is realized of a semi-
automatic manner.
Table 7: Results of comparison.
However, we can say that the use of our
proposed features in the other works can be
important in the increase of the rate of success of the
distinguishing between the benign and malignant
mass.
5 CONCLUSIONS
Characterization of mammographic mass and its
classification as being benign or malignant is
difficult. In this paper, we have tried to improve the
performance of the mass classification. We have
proposed a shape features that based on the region
features and the boundary ones. The results have
been validated by two algorithms of classification:
KNN and MLP. The found results were acceptable
with a rate of sensitivity and of specificity that
passed 95%.
The shape features can characterize the types of
mammographic masses. Since the signs of
malignancy of breast tumour are related to shape and
texture, shape features are insufficient, by
themselves, for a description of the masses more
effective. For this reason, it is better to add texture
features to our descriptors in order to increase the
accuracy rate for discriminating between benign
masses and malignant ones. In future work, we will
illustrate the effectiveness of the combination of the
texture features and shape ones in the diagnostic
process.
Detection phase is the most difficult step in a
CAD system. For this reason, the future work will be
dedicated to the automation of detection by using of
our approach of mass detection, based on Level Set,
presented in (Boujelben. A. et al, 2009) and using of
our approach of mass description indicated in this
paper.
REFERENCES
Alvarenga, A. V., Fernando, A., Wagner Coelho de
Albuquerque, C. I., (2006). Assessing the performance
of the normalised radial length and convex polygons
in distinguishing breast tumors on ultrasound images.
Pereira Revista Brasileira de Engenharia Biomédica,
Vol. 22, pp. 181-189.
Boujelben, A., Chaabani, A. C., TMAR, H., ABID, M.,
(2009). An approach based on RDM for analysis in
breast cancer detection. In International Conference on
Applied Informatics, pp. 103-108, Algerie.
Boujelben. A. Chaabani, A. C., TMAR, H., ABID, M.,
(2009). Level Set method for breast regions detection.
In International Conference on Medical Imaging from
technology to Application (ICMITA09), pp. 169-174,
Tunisie.
Cascio, U., Fauci, D., Golosio, B., Magro, R., Masala, L.,
Oliva, P., Raso, G., Stumbo, S., (2006). Massive
Lesions Classification using Features based on
Morphological Lesion Differences. In Transactions on
engineering computing and technology, IMTC.
Chang, R.F., Wu, W., Moon, W., Chen, D.R., (2005).
Automatic ultrasound segmentation and morphology
based diagnosis of solid breast tumors. Springer, Vol.
89, pp. 179-185.
Chettaoui, C., Djamel, K., Djouak, A., Maaref, H., (2005).
Etude de formes des globules drépanocytaires par
traitement numérique des images. In Int. Conf. SETIT,
Tunisia.
Delogu, P., Fantacci, M. E., Kasae, P., Retico, A., (2008).
Characterization of mammographic masses using a
gradient-based segmentation algorithm and a neural
classifier. In Comput Biol Med.
Denise, G., Rangayyan, R. M., Juliano Carvalho, D.,
Sérgio Santiago, A., (2008). Polygonal Modeling of
Contours of Breast Tumors With the Preservation of
Spicules. In IEEE Transations In Biomedical
Engineering, Vol. 55.
Heath, M., Bowyer, K., Kopans, D., Moore, R.,
Kegelmeyer, P. Jr., (2001). The Digital Database for
Screening Mammography. In Proceedings of the 5th
International Workshop on Digital Mammography,
SHAPE FEATURES FOR MASS DIAGNOSIS IN MAMMOGRAPHIC IMAGES
261