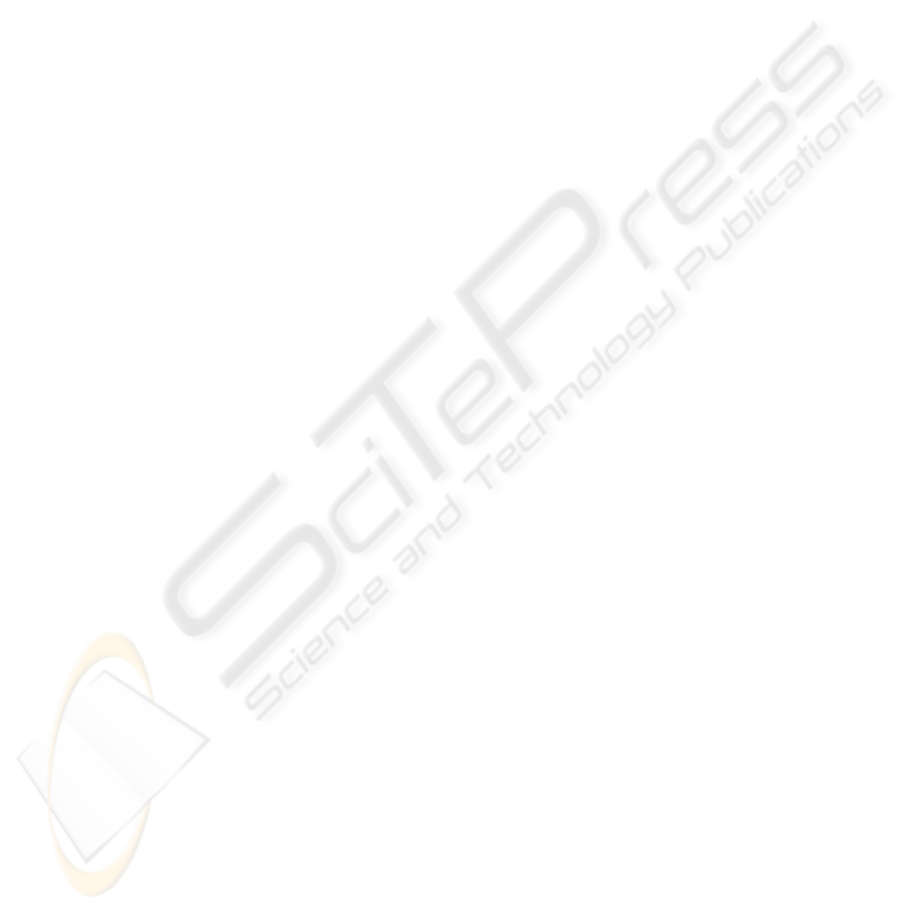
method for lower noise conditions.
Figure 3 shows some details extracted from the
real-noisy images. Results obtained with BLS-GSM
and SA-DCT are omitted, as they introduced an unac-
ceptable amount of artifacts. The joint approach pro-
posed in (Hirakawa and Parks, 2006) performs rea-
sonably well, however it has the tendency to introduce
zipper artifacts, blurriness (especially in highlight re-
gions), and in some cases fails in preserving high fre-
quency details. More difficult is the comparison be-
tween the BM3D and the joint approach LPA-ICI: the
former is obviously the best in restoring the details
which were present in the original image; on the other
hand the results suffers from the presence of artifacts,
which are anyhow usually tolerable. The latter ap-
proach instead, produces almost artifacts-free images,
but images lack of details where instead the BM3D
performed well; also a considerable amount of noisy
grain is still present. We believe that, in this experi-
ment session, where the noise amount was not drastic,
the BM3D yielded the most satisfactory results. We
shall conclude that in general, for higher noise levels
the joint approach LPA-ICI performs best, while for
lower noise levels the Block-Matching 3D is prefer-
able.
8 CONCLUSIONS
We compared several solutions for noise removal with
RAW images, and evaluated their performances based
on the quality of the demosaicked output images.
Both joint denoise-demosaic, and separate (denoise,
then demosaic) approaches were considered. The
methods have been selected among the state-of-the-
art ones, both in terms of PSNR and perceived qual-
ity. Also, one state-of-the-art demosaicking method
was used whenever it was necessary to demosaic a
previously denoised image. Two different kinds of
comparisons were carried out: one with 23 high-
quality images (Kodak database), which were artifi-
cially degraded, and another one with 10 RAW im-
ages, corrupted by real noise. In the former case the
ground-truths were available. We adopted as quality
measures, the PSNR, the Mean-SSIM-Index, and our
extension to the SSIM-Index for color images (the
CSSIM-Index). We showed how the proposed mea-
sure behaves in satisfactory agreement with the per-
ceptual subjective quality. We also concluded that
among the method considered, the joint approach pro-
posed in (Paliy et al., 2007) is preferable when the
image is severely impaired by noise, while the Block-
Matching-3D is preferable when the amount of noise
is reasonably low. We finally confirmed this fact, by
visually inspecting the denoised RAW images which
were originally degraded by real noise. We believe
that our work can shed more light on which are the
most promising research directions for further im-
provements in RAW image denoising.
ACKNOWLEDGEMENTS
The authors would like to thank Nokia Corporation
for providing the set of noisy RAW images.
REFERENCES
Dabov, K., Foi, A., Katkovnik, V., and K.Egiazarian (2006).
Image denoising with block-matching and 3d filtering.
In Proc. SPIE Electronic Imaging 2006.
Foi, A., Dabov, K., Katkovnik, V., and Egiazarian, K.
(2006). Shape-adaptive dct for denoising and im-
age reconstruction. In Proc. SPIE Electronic Imaging
2006.
Hirakawa, K. and Parks, T. W. (2006). Joint demosaicing
and denoising. In IEEE TIP August 2006.
ISIT (2007). Recent trends in denoising tutorial. htpp:
//www.stanford.edu/
˜
slansel/tutorial/.
Kurimo, E., Lepisto, L., Nikkanen, J., Gren, J., Kunttu, I.,
and Laaksonen, J. (2009). The effect of motion blur
and signal noise on image quality in low light imag-
ing. In Proceedings of SCIA2009.
Li, X., Gunturk, B., and Zhang, L. (2008). Image demosaic-
ing: a systematic survey. In Visual Communications
and Image Processing 2008.
Paliy, D., Katkovnik, V., Bilcu, R., Alenius, S., and Egiazar-
ian, K. (2007). Spatially adaptive color filter array
interpolation for noiseless and noisy data. In Int. J.
Imaging Sys. Tech., Sp. Iss. Appl. Color Image Pro-
cess., vol. 17, no. 3, pp. 105-122.
Portilla, J., Strela, V., Wainwright, M., and Simoncelli, E. P.
(2003). Image denoising using scale mixtures of gaus-
sians in the wavelet domain. In IEEE Transactions on
Image Processing. vol 12, no. 11, pp. 1338-1351.
Vansteenkiste, E., van der Weken, D., Philips, W., and
Kerre, E. (2006). Perceived image quality measure-
ment of state of the art noise reduction schemes. In
ACIVS06. Springer DOI Link 0609.
Wang, Y., Liu, W., and Wang, Y. (2008). Color image
quality assessment based on quaternion singular value
decomposition. In cisp, vol. 3, pp.433-439, 2008
Congress on Image and Signal Processing.
Wang, Z., Bovik, A. C., Sheikh, H. R., and Simoncelli, E. P.
(2004). Image quality assessment: From error visibil-
ity to structural similarity. In IEEE Transactions on
Image Processing, vol. 13, no. 4, Apr. 2004.
EVALUATION OF DENOISING METHODS WITH RAW IMAGES AND PERCEPTUAL MEASURES
173