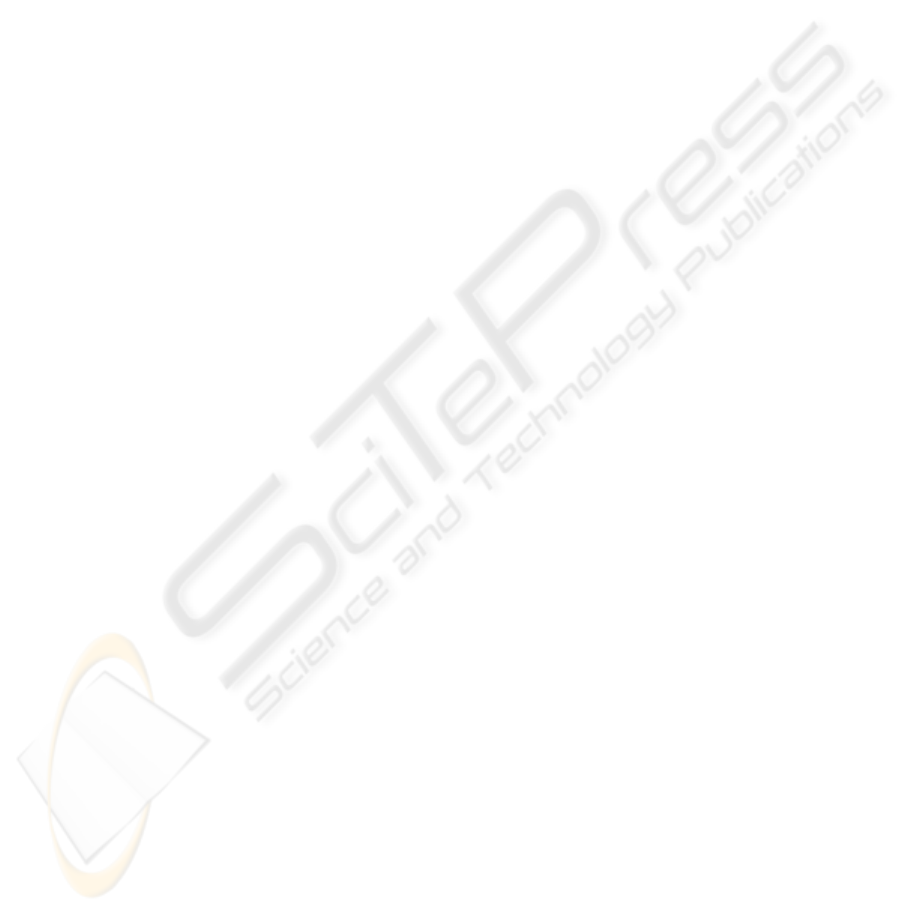
have similar effectiveness performance. It is impor-
tant to note that in this data set there is almost no scale
variance (see Figure 6).
5 CONCLUSIONS
This article presented a novel shape description algo-
rithm, the Scale-Invariant Multiscale Fractal Dimen-
sion (SIMFD). This descriptor is based on the use
of the Fractal Dimension concept – a real number
that describes boundary complexity and self-affinity
characteristics. The proposed method relies on three
steps: a pre-IFT area normalization, the use of a new
algorithm for obtaining the multiscale fractal dimen-
sion, and the use of a method for extracting the most
relevant fragment of the MFD curve.
An experimental validation was conduced, com-
paring SIMFD to the Multiscale Fractal Dimension
and to four other shape descriptors. Experiments re-
sults have shown that the new descriptor is at least as
effective as the Beam Angle Statistics and the Mul-
tiscale Fractal Dimension, outperforming other well-
known shape descriptors. Moreover, it has been em-
pirically demonstrated that SIMFD is scale-invariant.
Future work includes extending the proposed de-
scriptor to more complex binary images (several con-
tours, cavities inside the shape, etc.). Extending the
description algorithm to grey-scale images is also be-
ing studied.
ACKNOWLEDGEMENTS
Authors thanks CNPq, CAPES, and FAPESP for fi-
nancial support.
REFERENCES
Arica, N. and Vural, F. (2003). BAS: a perceptual shape
descriptor based on the beam angle statistics. Pattern
Recognition Letters, 24(9–10):31–41.
Bober, M. (2001). MPEG–7 visual shape descriptors. IEEE
Transactions on Circuits and Systems for Video Tech-
nology, 11(6):716–719.
Chaudhuri, B. and Sarkar, N. (1995). Texture segmentation
using fractal dimension. PAMI, 17(1):72–77.
Costa, L., Campos, A., and Manoel, E. (2001). An inte-
grated approach to shape analysis: Results and per-
spectives. In International Conference on Quality
Control by Artificial Vision, pages 23–34.
da S. Torres, R. and Falc˜ao, A. (2007). Contour salience
descriptors for effective image retrieval and analysis.
Image and Vision Computing, 25(1). 3–13.
da S. Torres, R., Falc˜ao, A., and da F. Costa, L. (2004). A
graph–based approach for multiscale shape analysis.
Pattern Recognition, 37(6):1163–1174.
Datta, R., Joshi, D., Li, J., and Wang, J. Z. (2008). Image
retrieval: Ideas, influences, and trends of the new age.
ACM Computing Surveys, 40(2):1–60.
Falc˜ao, A., Stolfi, J., and de A. Lotufo, R. (2004). The
image foresting transform: Theory, algorithms, and
applications. PAMI, 26(1):19–29.
Fisher, Y. (1995). Fractal image compression: theory and
applications. Springer-Verlag, New York.
Gonzalez, R., Woods, R., and Eddins, S. (2004). Digital im-
age processing using MATLAB. Prentice Hall Upper
Saddle River, NJ.
Hu, M. (1962). Visual pattern recognition by moment in-
variants. IRE Trans. on Inf. Theory, 8(2):179–187.
Ling, H. and Jacobs, D. (2007). Shape classification using
the inner-distance. PAMI, 29(2):286–299.
Liu, Y., Zhang, D., Lu, G., and Ma, W. (2007). A survey of
content-based image retrieval with high-level seman-
tics. Pattern Recognition, 40(1):262–282.
Mandelbrot, B. (1982). The Fractal Geometry of Nature.
W. H. Freeman, San Francisco, CA, USA.
Muller, H., Muller, W., Squire, D., Maillet, S., and Pun, T.
(2001). Performance evaluation in content-based im-
age retrieval: Overview and proposals. Pattern Recog-
nition Letters, 22(5):593–601.
Sebastian, T. B., Klein, P. N., and Kimia, B. B. (2004).
Recognition of shapes by editing their shock graphs.
PAMI, 26(5):550–571.
Yang, X., Bai, X., Latecki, L. J., and Tu, Z. (2008). Improv-
ing shape retrieval by learning graph transduction. In
ECCV, pages 788–801.
Zhang, D. and Lu, G. (2004). Review of shape representa-
tion and description. Pattern Recognition, 37(1):1–19.
VISAPP 2010 - International Conference on Computer Vision Theory and Applications
190