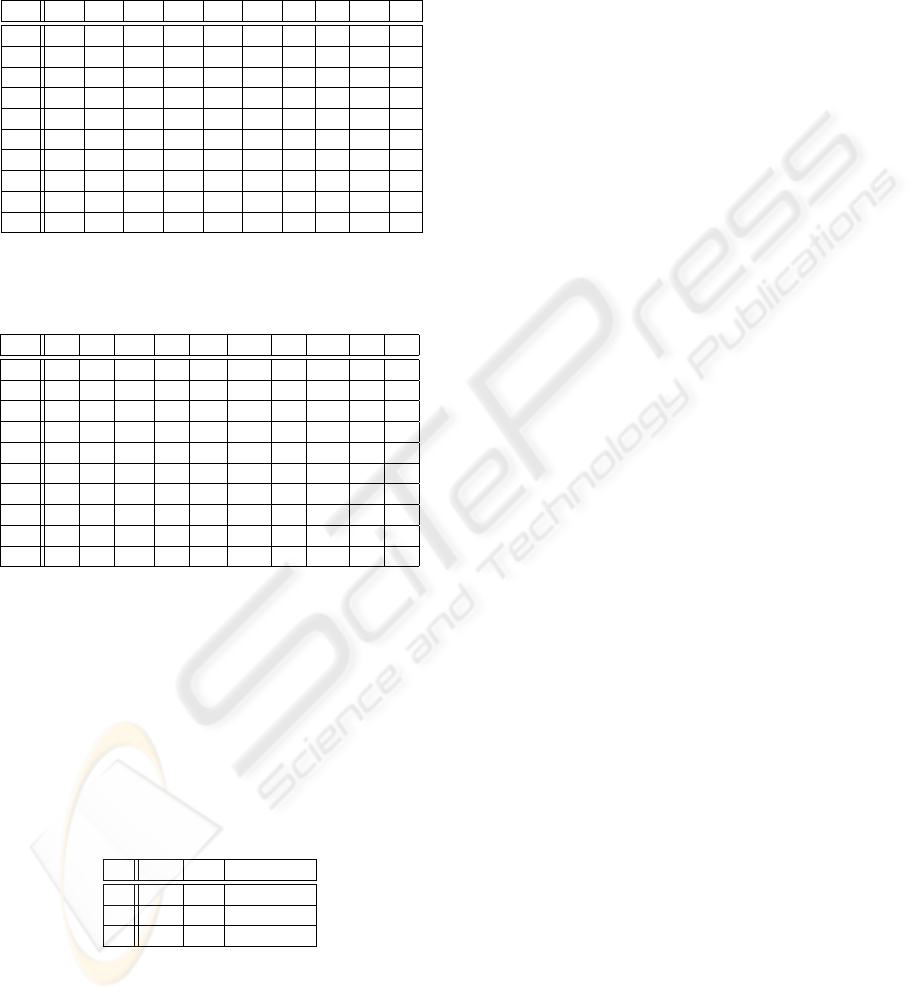
Table 4: Repeatability gain. For each couple of detectors
D
1
&D
2
, we show gain
rep
D
1
&D
2
(ε = 3). To determine which
detector is the most complementary in terms of repeatability
with HA, for instance, look at the HA row and column (here
in blue), it shows that it is HEL. For each detector, the best
result appears in bold.
BE FA HAL HA HEL KA KR MO SI SR
FA 1.55 0
HAL 0.75 -0.36 0
HA 3.77 0.32 -0.36 0
HEL 4.48 2.12 0.36 4.20 0
KA -0.17 -1.69 -0.39 -0.38 2.03 0
KR 4.71 0.22 0.90 3.88 5.75 1.81 0
MO 3.78 -0.69-0.62 3.11 4.47 -1.34 2.83 0
SI 3.57 1.19 0.76 3.40 2.37 0.82 4.98 3.00 0
SR 1.01 0.38 0.09 0.66 -2.06 1.48 1.70 0.82 -0.19 0
SU 3.53 -1.21 1.65 2.24 5.00 2.60 3.50 2.23 2.46 2.63
Table 5: Distribution gain. For each couple of detectors
D
1
&D
2
, we show gain
RD
D
1
&D
2
,S2
(ε = 3) (see 4 for an exam-
ple of how to read the table).
BE FA HAL HA HEL KA KR MO SI SR
FA 3.96 0
HAL 2.23 1.30 0
HA 6.14 5.11 1.19 0
HEL 8.13 7.80 1.30 6.09 0
KA 8.53 6.85 7.09 5.54 9.27 0
KR 6.47 4.23 3.28 5.86 7.98 6.08 0
MO 3.82 0.02 6.46 3.26 8.62 12.07 2.30 0
SI 5.32 4.96 1.52 5.95 5.39 6.88 6.12 2.92 0
SR 4.15 3.79 4.89 3.63 0.51 11.58 5.30 10.38 1.71 0
SU 6.16 0.10 3.30 4.18 9.72 10.17 3.20 3.27 4.45 7.12
rizes the most complementary detectors to the detec-
tors in terms of contribution, repeatability and region-
wise distribution. The most complementary detectors
between them are Kadir and SUSAN, Kitchen and
Rosenfeld and Hessian-Laplace, Moravec and Kadir
i.e. they return the most distinct sets of feature points.
Table 6: This table summarizes the most complementary
detectors to Harris, FAST and SIFT in terms of contribution
(Cont.) (ε = 3) (results are similar whether all the feature
points or only the repeated points are taken into account),
repeatability (R) and region based distribution (RD
S2
).
D Cont. R RD
D
1
&D
2
,S2
HA HEL HEL BE
FA HEL HEL HEL
SI HEL KR KA
6 CONCLUSIONS
We proposed an evaluation and a comparison of
eleven well-known feature point detectors based on
new criteria used to characterize spatial distribution
and complementarity. This study aims to be helpful
for any applications that need feature points well dis-
tributed in the image. It also helps to select the most
complementary detectors in terms of region based dis-
tribution. This work will be extended on larger trans-
formations between the images.
REFERENCES
Bay, H., Ess, A., Tuytelaars, T., and Gool, L. V. (2006).
SURF: Speeded up robust features. CVIU, pages 346–
359.
Beaudet, P. R. (1978). Rotationally invariant image opera-
tors. In ICPR, pages 579–583, Kyoto, Japan.
Gil, A., Martinez, O., Ballesta, M., and Reinoso, O. (2009).
A comparative evaluation of interest point detectors
and local descriptors for visual SLAM. MVA.
Harris, C. and Stephens, M. (1988). A combined corner
and edge detector. In Alvey Vision Conference, pages
147–151, Manchester, United-Kingdom.
Hartley, R. I. and Zisserman, A. (2004). Multiple View Ge-
ometry in Computer Vision. Cambridge University
Press, ISBN: 0521540518, second edition.
Kadir, T., Zisserman, A., and Brady, M. (2004). An affine
invariant salient region detector. In ECCV, pages 404–
416, Prague, Czech Republic.
Kitchen, L. and Rosenfeld, A. (1982). Gray level corner
detection. PRL, 1(2):95–102.
Lhuillier, M. and Quan, L. (2002). Match propagation
for image-based modeling and rendering. PAMI,
24(8):1140–1146.
Lowe, D. G. (1999). Object recognition from local scale-
invariant features. In ICCV, volume 2, pages 1150–
1157, Kerkyra, Greece.
Mikolajczyk, K., Leibe, B., and Schiele, B. (2005). Local
features for object classe recognition. In ICCV, vol-
ume 2, pages 1792–1799, Beijing, China.
Mikolajczyk, K. and Schmid, C. (2004). Scale & affine
invariant interest point detectors. IJCV, 60(1):63–86.
Moravec, H. P. (1977). Toward automatic visual obstacle
avoidance. In IJCAI, volume 2, pages 584–584, Mas-
sachusetts, USA.
Noble, J. A. (1988). Finding corners. IVC, 6(2):121–128.
Rosten, E. and Drummond, T. (2006). Machine learning
for high-speed corner detection. In ECCV, volume 1,
pages 430–443, Graz, Austria.
Schmid, C., Mohr, R., and Bauckhage, C. (2000). Evalua-
tion of interest point detectors. IJCV, 37(2):151–172.
Shi, J. and Tomasi, C. (1994). Good features to track. In
CVPR, pages 593–600, Seattle, USA.
Smith, S. M. and Brady, J. M. (1997). Susan – a new ap-
proach to low level image processing. IJCV, 23(1):45–
78.
COMPLEMENTARITY OF FEATURE POINT DETECTORS
339