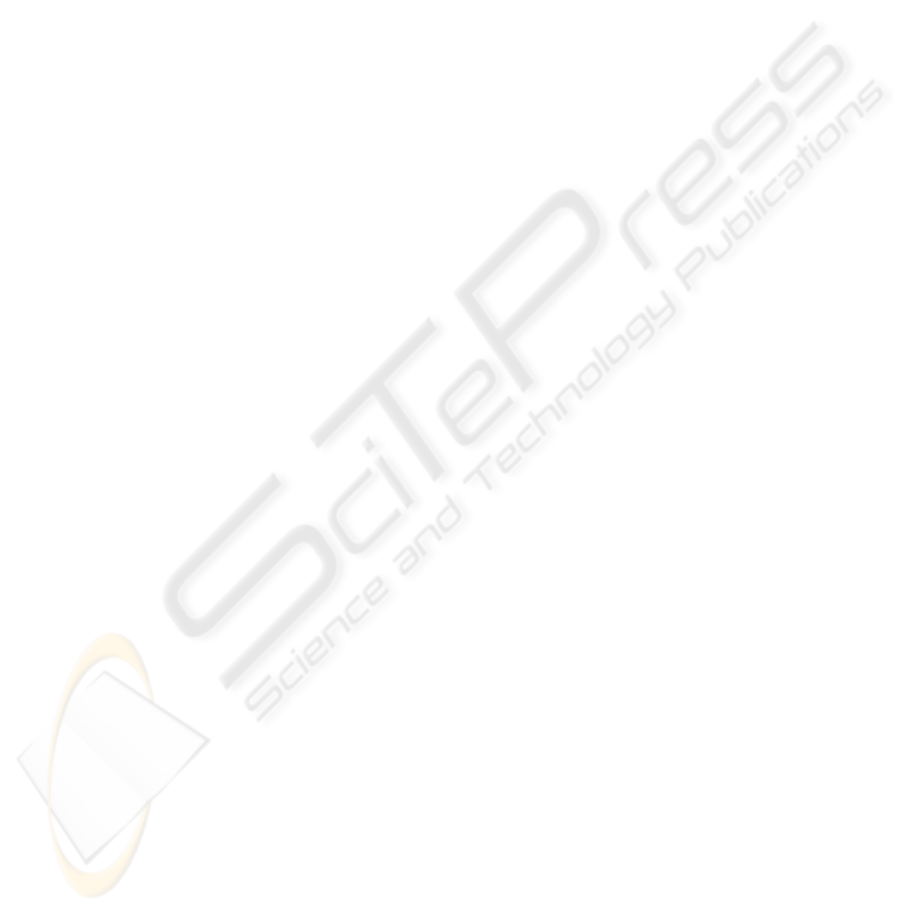
ject relations in every frame from the test sequence
is manually annotated. It should be noted that this
is an ambiguous task depending on the judgement of
the human observer. The AUC ROC results of OR
MLN and SCOR MLN for the test sequence visu-
alised in Figure 7 are listed in Table 2. Hereby the
inferred probabilities of 3384 groundings per mod-
elled object relation were evaluated. The SCOR MLN
achieved significantly improved results for most rela-
tions, supporting the claim for considering scene con-
text in complex relational classification tasks.
6 CONCLUSIONS
This contribution introduced an approach for inferring
a conceptual representation of relations between ob-
jects in traffic scenes using Markov logic. Soft defini-
tions for object relations in terms of discretised sen-
sor data were learned, as well as typical combinations
of such object relations. These learned models were
tested on automatically segmented traffic videos from
an on-board stereo camera platform. Taking into ac-
count both the soft definitions and typical scene con-
text, the conditional probability of several object rela-
tions given the learned model and evidence was com-
puted for each object pair in each frame of a test se-
quence. The results complied in most cases with the
judgement of a human observer. The proposed ap-
proach can be seen as a promising step towards bridg-
ing the gap between low-level image processing and
high-level situation interpretation. Future work con-
siders verifying the proposed approach on a broader
statistical base, augmenting the model with temporal
dependencies and closing the loop to low-level scene
segmentation.
ACKNOWLEDGEMENTS
The authors gratefully acknowledge support of this
work by the Deutsche Forschungsgemeinschaft (Ger-
man Research Foundation) within the Transregional
Collaborative Research Centre 28 “Cognitive Auto-
mobiles”.
REFERENCES
Arens, M., Ottlik, A., and Nagel, H. H. (2004). Using be-
havioral knowledge for situated prediction of move-
ments. In KI, pages 141–155. Springer-Verlag.
Bachmann, A. and Dang, T. (2008). Improving motion-
based object detection by incorporating object-
specific knowledge. International Journal of Intel-
ligent Information and Database Systems (IJIIDS),
2(2):258–276.
Bachmann, A. and Lulcheva, I. (2009). Combining low-
level segmentation with relational classification. In
ICCV2009; IEEE Workshop on Visual Surveillance
(VS), pages 1216–1221.
Cohn, A. G., Hogg, D., Bennett, B., Devin, V., Galata, A.,
Magee, D., Needham, C., and Santos, P. (2006). Cog-
nitive vision: Integrating symbolic qualitative repre-
sentations with computer vision. In Christensen, H. I.
and Nagel, H. H., editors, Cognitive Vision Systems:
Sampling the Spectrum of Approaches, volume 3948
of LNCS, pages 221–246. Springer.
Fern
´
andez, C., Baiget, P., Roca, X., and Gonz
´
ılez, J.
(2008). Interpretation of complex situations in a
semantic-based surveillance framework. Image Com-
mun., 23(7):554–569.
Georis, B., Mazire, M., Br
´
emond, F., and Thonnat, M.
(2006). Evaluation and knowledge representation for-
malisms to improve video understanding. In Proceed-
ings of the International Conference on Computer Vi-
sion Systems (ICVS’06), New-York, NY, USA.
Gerber, R. and Nagel, H. H. (2008). Representation of oc-
currences for road vehicle traffic. Artif. Intell., 172(4-
5):351–391.
Hotz, L., Neumann, B., and Terzic, K. (2008). High-level
expectations for low-level image processing. In KI,
pages 87–94. Springer-Verlag.
Howarth, R. J. and Buxton, H. (2000). Conceptual descrip-
tions from monitoring and watching image sequences.
Image Vision Comput., 18(2):105–135.
Hummel, B., Thiemann, W., and Lulcheva, I. (2008). Scene
understanding of urban road intersections with de-
scription logic. In Cohn, A. G., Hogg, D. C., M
¨
oller,
R., and Neumann, B., editors, Logic and Probability
for Scene Interpretation, number 08091 in Dagstuhl
Seminar Proceedings, Dagstuhl, Germany.
Kok, S., Sumner, M., Richardson, M., Singla, P., Poon, H.,
Lowd, D., and Domingos, P. (2007). The Alchemy
system for statistical relational AI. Technical report,
Department of Computer Science and Engineering,
University of Washington, Seattle, WA.
Neumann, B. and M
¨
oller, R. (2008). On scene interpreta-
tion with description logics. Image Vision Comput.,
26(1):82–101.
Richardson, M. and Domingos, P. (2006). Markov logic
networks. Machine Learning, 62(1-2):107–136.
Tran, S. D. and Davis, L. S. (2008). Event modeling and
recognition using markov logic networks. In ECCV
’08: Proceedings of the 10th European Conference on
Computer Vision, pages 610–623, Berlin, Heidelberg.
Springer-Verlag.
Vernon, D. (2006). The space of cognitive vision. In Chris-
tensen, H. I. and Nagel, H. H., editors, Cognitive Vi-
sion Systems: Sampling the Spectrum of Approaches,
volume 3948 of LNCS, pages 7–26. Springer.
UNDERSTANDING OBJECT RELATIONS IN TRAFFIC SCENES
395