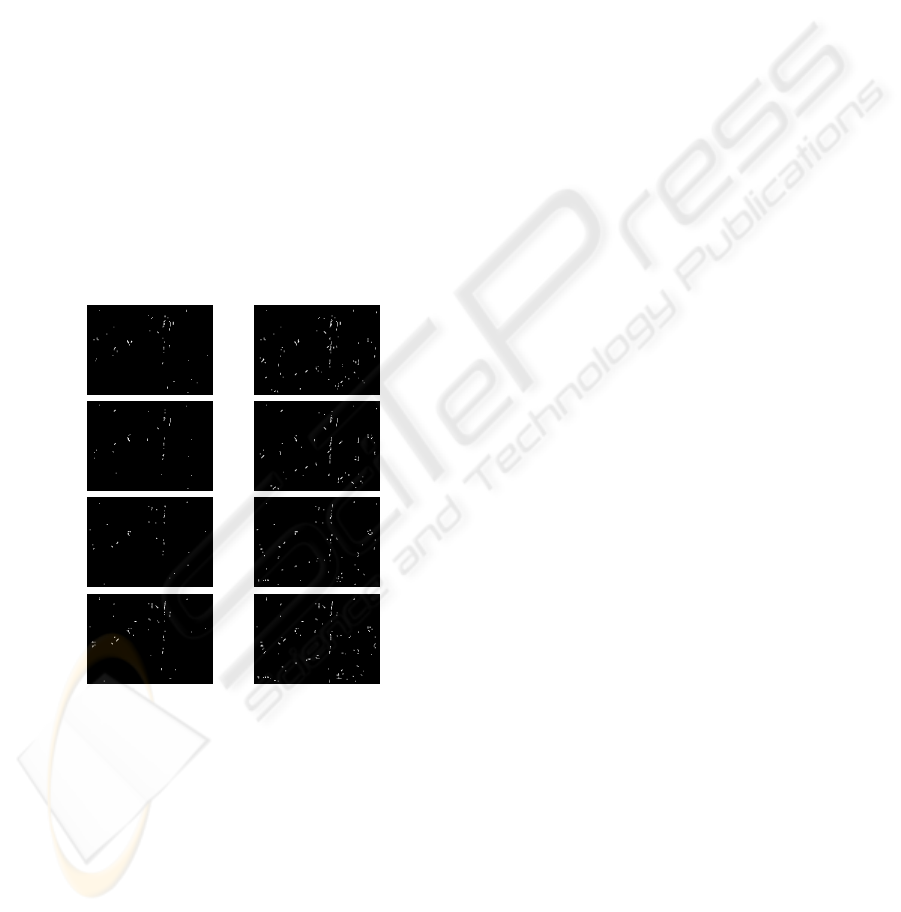
fluence of different illumination conditions, the intro-
duction of different kind of correlation measures for
the matching step and how all the results are merged.
The results are quite encouraging and they highlight
the best conditions: acquisition with ambient illumi-
nation, detection with the method that considers the
crack as a Gaussian function and matching with a cor-
relation measure based on the gradients of the image.
Even if we have a specific application, i.e. crack de-
tection, this method can be used to detect other types
of defects in other type of difficult objects (low con-
trasted defects in highly textured objects). This work
can be improved and future work will introduce a
more systematic study of the influence of the light
position using (Drbohlav and Chantler, 2005). The
matching step will be evaluated quantitatively and the
tested images will be completed (with natural images
and different kind of textures). Finally, the 3D re-
construction step will be added to complete the crack
detection and include other defect detection, like the
snatching of pieces of road.
GC SMAD
Fusion
with
Init
Fusion
with
Gaus
Fusion
with
InMM
Fusion
with
GaMM
Figure 7: Detection maps with fusion method – Results are
shown for the same image as the results in Figure 3 and
only for two of the best correlation measures and for each
detection methods studied. It illustrates that the best perfor-
mances are reached with the Gaus detection method com-
bined with a GC matching.
REFERENCES
Acosta, J., Adolfo, L., and Mullen, R. (1992). Low-Cost
Video Image Processing System for Evaluating Pave-
ment Surface Distress. TRR: Journal of the Trans-
portation Research Board, 1348:63–72.
Augereau, B., Tremblais, B., Khoudeir, M., and Legeay, V.
(2001). A Differential Approach for Fissures Detec-
tion on Road Surface Images. In International Con-
ference on Quality Control by Artificial Vision.
Chambon, S. and Crouzil, A. (2003). Dense matching using
correlation: new measures that are robust near occlu-
sions. In BMVC.
Chambon, S., Gourraud, C., Moliard, J.-M., and Nicolle, P.
(2010). Road crack extraction with adapted filtering
and markov model-based segmentation. In VISAPP.
Drbohlav, O. and Chantler, M. (2005). On optimal light
configurations in photometric stereo. In ICCV, pages
1707–1712.
Fukuhara, T., Terada, K., Nagao, M., Kasahara, A., and
Ichihashi, S. (1990). Automatic pavement-distress-
survey system. ASCE, Journal of Transportation En-
gineering, 116(3):280–286.
Kaseko, M. and Ritchie, S. (1993). A neural network-based
methodology for pavement crack detection and clas-
sification. Transportation Research Part C: Emerging
Technologies information, 1(1):275–291.
Lee, H. and Oshima, H. (1994). New Crack-Imaging Proce-
dure Using Spatial Autocorrelation Function. ASCE,
Journal of Transportation Engineering, 120(2):206–
228.
Petrou, M., Kittler, J., and Song, K. (1996). Automatic sur-
face crack detection on textured materials. Journal of
Materials Processing Technology, 56(1–4):158–167.
Scharstein, D. and Szeliski, R. (2002). A Taxomomy and
Evaluation of Dense Two-Frame Stereo Correspon-
dence Algorithms. IJCV, 47(1):7–42.
Schmidt, B. (2003). Automated pavement cracking assess-
ment equipment – State of the art. Technical Report
320, Surface Characteristics Technical Committee of
the World Road Association (PIARC).
Subirats, P., Fabre, O., Dumoulin, J., Legeay, V., and Barba,
D. (2006). Automation of pavement surface crack de-
tection with a matched filtering to define the mother
wavelet function used. In EUSIPCO.
Sutton, M., Yan, J., Tiwari, V., Schreier, H., and Orteu, J.
(2008). The effect of out-of-plane motion on 2D and
3D digital image correlation measurements. Optics
and Lasers in Engineering, 46:746–757.
Tanaka, N. and Uematsu, K. (1998). A Crack Detection
Method in Road Surface Images Using Morphology.
In Workshop on Machine Vision Applications, pages
154–157.
Wang, K. and Gong, W. (2002). Automated Pavement Dis-
tress Survey: A Review and A New Direction. In
Pavement Evaluation Conference, pages 21–25.
Zabih, R. and Woodfill, J. (1994). Non-parametric Local
Transforms for Computing Visual Correspondence. In
ECCV, pages 151–158.
VISAPP 2010 - International Conference on Computer Vision Theory and Applications
354