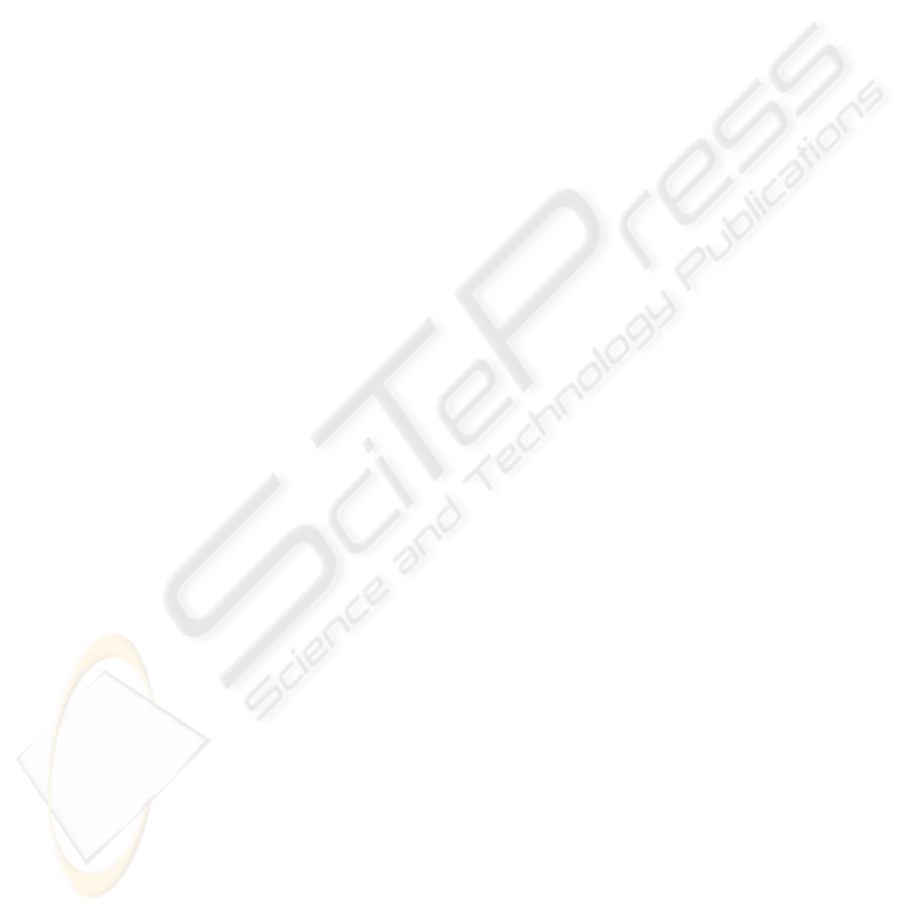
6 CONCLUSIONS
Machine learning based on SVM classifier with re-
gion descriptors as input has been improved by multi
view management. We have combined the region
classification results coming from several 2D images
of a skew surface, using the matched vertices of the
reconstructed 3D model. This approach has been
applied to the design of a complete 3D and colour
wound assessment tool. Experimental results show
that the fusion of 2D classification enables more ac-
curate tissue classification. Moreover, as the results
can be mapped on the mesh surface of the wound 3D
model, real tissue surfaces and volumes can be com-
puted on it. Future works include several tests on
a larger image database. We also intend to improve
these results by matching regions from more than two
views and by testing colour descriptors invariant to
viewpoint and lighting conditions.
REFERENCES
Albouy, B., Koenig, E., Treuillet, S., and Y.Lucas: (2006).
Accurate 3d structure measurements from two uncali-
brated views. In ACIVS, pages 1111–1121,.
Callieri, M., Cignoni, P., Coluccia, M., Gaggio, G., Pingi,
P., Romanelli, M., and Scopigno, R. (2003). Derma :
monitoring the evolution of skin lesions with a 3d sys-
tem. In 8th Int. Work. on Vis. Mod. and Visualization,
pages 167–174.
Carson, C., Belongie, S., Greenspan, H., and Malik,
J. (2002). Blobworld: image segmentation using
expectation-maximization and its application to image
querying. IEEE Trans. PAMI, 24(8):1026–1038.
Chapelle, O., Vapnik, V., Bousquet, O., and Mukherjee, S.
(2001). Choosing kernel parameters for support vector
machines. Machine Learning, pages 131–160.
Comaniciu, D. and Meer, P. (2002). Mean shift: a robust
approach toward feature space analysis. IEEE Trans.
PAMI, 24(5):603–619.
Deng, Y., Kenney, S., Moore, M., and Manjunath, B. S.
(1999). Peer group filtering and perceptual color im-
age quantization. In IEEE Inter. Symp. on Circ. and
Sys. VLSI (ISCAS’99), volume 4, pages 21–24, Or-
lando, FL.
Deng, Y. and Manjunath, B. S. (2001). Unsupervised
segmentation of colour-texture regions in images and
video. IEEE Trans. PAMI ’01, 23:800–810.
Huang, T., Weng, R. C., and Lin, C.-J. (2006). Generalized
bradley-terry models and multi-class probability esti-
mates. Journal of Machine Learning Research, 7:85–
115.
Jones, C. D., Plassmann, P., Stevens, R. F., Pointer, M. R.,
and McCarthy, M. B. (2006). Good practice guide to
the use of mavis ii. Technical report, Medical Imaging
Research Unit TR-07-06, Univ. of Glamorgan.
Kolesnik, M. and Fexa, A. (2004). Segmentation of wounds
in the combined color-texture feature space. SPIE
Medical Imaging, 5370:549–556.
Krouskop, T. A., Baker, R., and Wilson, M. S. (2002). A
noncontact wound measurement system. J Rehabil
Res Dev, 39(3):337–345.
Landis, J. and Koch, G. (1977). The measurement of ob-
server agreement for categorical data. Biometrics,
33:159–174.
Lin, H.-T. and Li., L. (2005). Infinite ensemble learning
with support vector machines. J. Gama et al., eds.,
Machine Learning: ECML ’05, Lecture Notes in Arti-
ficial Intelligence, 3720:242–254, Springer–Verlag.
Malian, A., Heuvel van den, F., and Azizi, A. (2002). A
robust photogrammetric system for wound measure-
ment. In International Archives of Photogrammetry
and Remote Sensing, volume 34, pages 264 –269,
Corfu, Greece.
Oduncu, H., Hoppe, H., Clark, M., Williams, R., and Hard-
ing, K. (2004). Analysis of skin wound images using
digital color image processing: a preliminary commu-
nication. Int J Low Extrem Wounds, 3(3):151–156.
Ojala, T., Pietikainen, M., and Maenpaa, T. (2002). Mul-
tiresolution gray-scale and rotation invariant texture
classification with local binary patterns. IEEE Trans.
PAMI, 24(7):971–987.
Treuillet, S., Albouy, B., and Lucas, Y. (2009). Three-
dimensional assessment of skin wounds using a stan-
dard digital camera. IEEE Trans. on Medical Imaging,
28:752–762.
Wannous, H., Treuillet, S., and Lucas, Y. (2007). Su-
pervised tissue classification from color images for a
complete wound assessment tool. In 29th Inter. Conf.
of the IEEE Eng. in Med. and Bio. Soc. EMBS’07,
pages 6031–6034.
Wannous, H., Treuillet, S., and Lucas, Y. (2010). Robust tis-
sue classification for reproducible wound assessment
in telemedicine environments. to appear in the Jour-
nal of Electronic Imaging.
Zheng, H., Bradley, L., Patterson, D., Galushka, M., and
Winder, J. (2004). New protocol for leg ulcer tissue
classification from colour images. In 26th Inter. Conf.
of the IEEE Eng. in Med. and Bio. Soc. EMBS’04, vol-
ume 1, pages 1389–1392.
VISAPP 2010 - International Conference on Computer Vision Theory and Applications
104