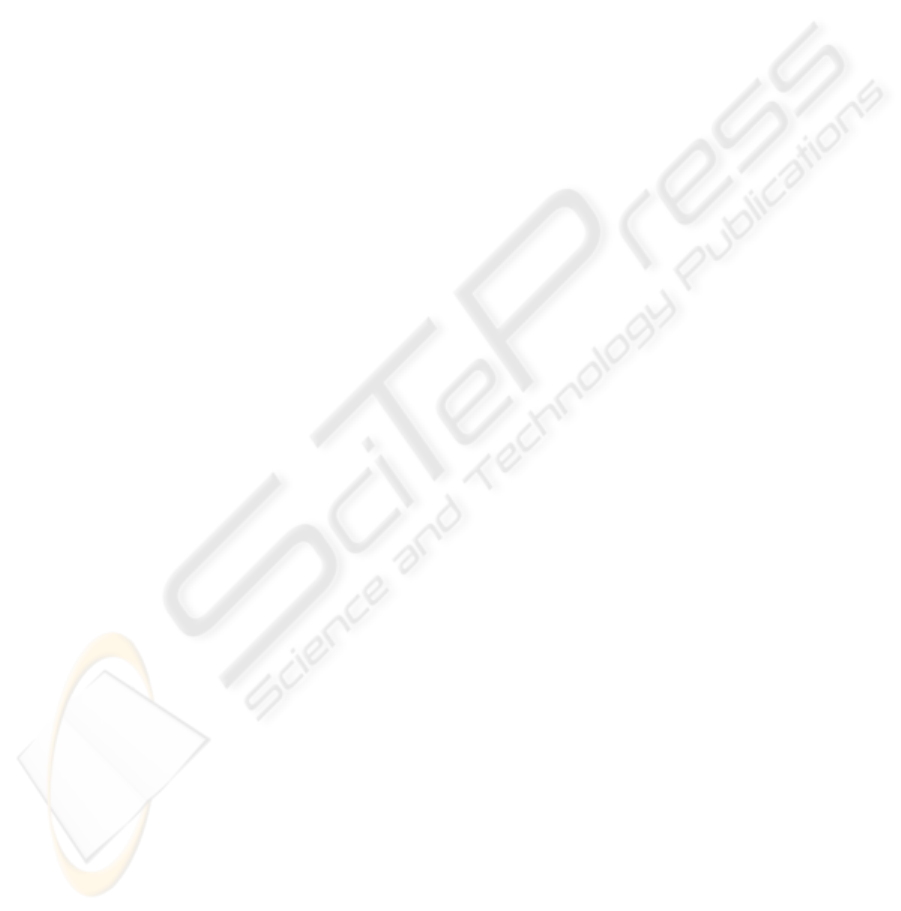
4 EXPERIMENTAL RESULTS
In this section, the results of our approach are pre-
sented which are applied to two different datasets
taken from our video database. The approach is first
tested on a synthetic video, later applied on real scene.
4.1 Synthetic Sequence
Figure 3 shows the tracking results for the synthetic
video. In this sequence, the segmentation is ideal and
visual features of the detected object during normal
motion remain the same. It can be seen from the
video sequence that the occlusion event is observed
four times in random intervals of time as indicated by
white circles. The labellings and status-tags are pre-
sented along with the tracking paths of moving ob-
jects.
In Figure 3(a), the results of missed associations
are identified in frame t + 25, t + 35, t + 37 and t + 41
which are indicated by red arrows. These ambigui-
ties are observed when the objects split after occlu-
sions. In Figure 3(b), the results of our proposed
approach are presented in which the qualitative rea-
soning modules are used with data association. The
resolved uncertainties are highlighted with yellow ar-
rows whereas the identities with status-tags are shown
on right side of images. It can be observed that the
tracking is successfully done by keeping all the real-
time tracking events under consideration.
4.2 Traffic Sequence
The robustness of the proposed approach is demon-
strated on a real-time traffic sequence where the ob-
jects are moving in both the parallel and opposite
tracks as shown in Figure 3. The multiple occlusions
and separations are taken place in short interval of
time which is the key challenge here. Moreover, the
camera position is not parallel to road but instead, it is
tilted which results in perspective view and therefore
a significant variation in object’s size is observed.
Figure 3(c) shows the outcome of tracking and
identity management before applying our proposed
approach. The errors due to wrong association
weights are indicated by red arrows during split in
t + 76, t + 83, t + 88, t + 96 and t + 103 where the
objects lost their identities when occlusion is over. In
Figure 3(d), these uncertainties are handled by our six
qualitative modules which are integrated for data as-
sociation. For example, it can be seen that in t + 76
and t + 83 the correct identities are successfully iden-
tified after split using our qualitative modules. The
respective identity and status-tag can be seen in right-
side of the frame. Throughout the tracking, it can
been seen that all the real-time events are occurred (
for example new entry, exit entry, occlusion and sep-
aration) in the sequence and plausible interpretations
(i.e. highlighted by yellow arrows) are efficiently han-
dled by our proposed technique.
5 CONCLUSIONS AND FUTURE
WORK
In this paper, limitations of data-association during
conflicted situations are resolved by assigning the log-
ical tags to the moving object which explicitly con-
trol these ambiguities even if the discrete data is in-
complete. The proposed approach is successfully
tested on synthetic simulation and real-time traffic se-
quences. Future work will be focused to interpret the
behaviors of moving objects.
ACKNOWLEDGEMENTS
This work is supported by Forschungspraemie
(BMBF:FKZ 03FPB00213) and Transregional Col-
laborative Research Center SFB/TRR 62 funded by
the German Research Foundation (DFG).
REFERENCES
Bar-Shalom, Y. and Fortmann, T. E. (1987). Tracking and
data association, volume 179 of Mathematics in Sci-
ence and Engineering.
Bennett, B. (2008). Combining logic and probability in
tracking and scene interpretation. In Cohn, A. G.,
Hogg, D. C., M
¨
oller, R., and Neumann, B., editors,
Logic and Probability for Scene Interpretation, num-
ber 08091 in Dagstuhl Seminar Proceedings.
Bennett, B., Magee, D., Cohn, A. G., and Hogg, D. (2008).
Enhanced tracking and recognition of moving objects
by reasoning about spatio-temporal continuity. Image
Vision Computer, 26(1):67–81.
Blake, A. (2006). Visual Tracking: A Short Research
Roadmap. Springer, New York.
Frintrop, S., Knigs, A., Hoeller, F., and Schulz, D. (2009).
Visual person tracking using a cognitive observation
model. In Workshop on People Detection and Track-
ing at the IEEE ICRA.
Halpern, J. Y. (1990). An analysis of first-order logics of
probability. Artificial Intelligence, 46(3):311–350.
Kailath, T. (1967). The divergence and bhattacharyya dis-
tance measures in signal selection. IEEE Transactions
on Communications[legacy, pre - 1988], 15(1):52–60.
RESOLVING DATA-ASSOCIATION UNCERTAINTY - In Mutli-object Tracking through Qualitative Modules
465