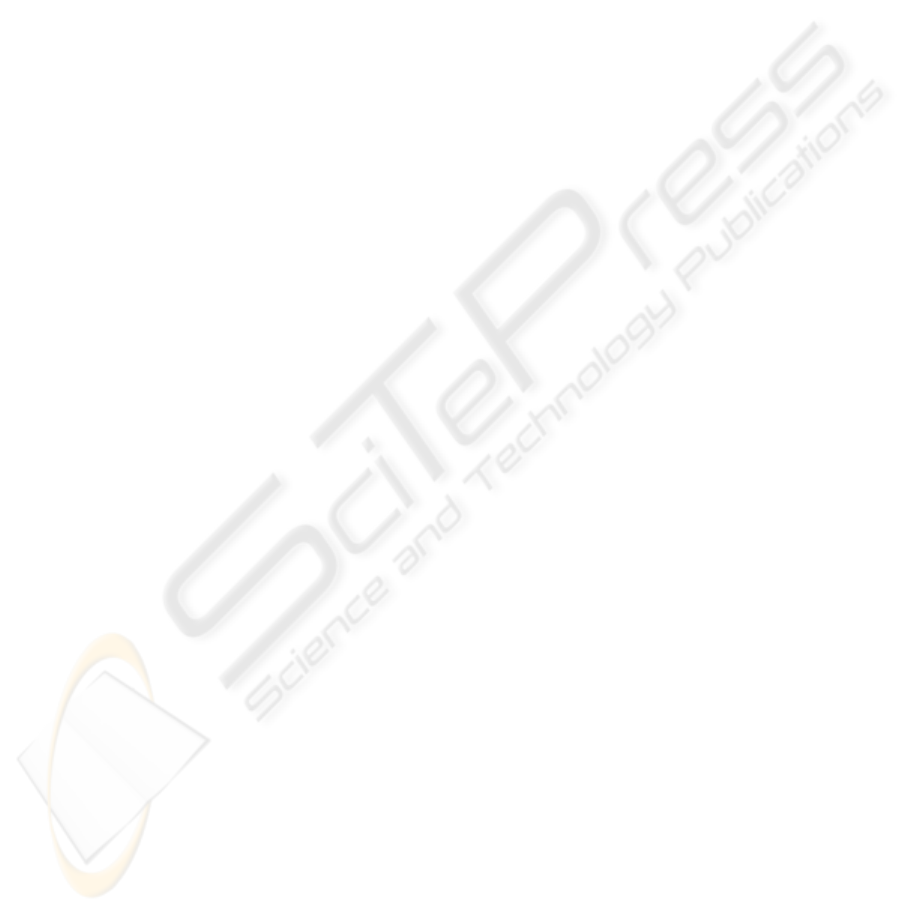
ment between expert biologists and 71-75% accura-
cies from similar works by (Blaschko et al., 2005)
and (Lisin, 2006), our techniques perform very well
on this very complex marine data set.
We compare the performance of the Standard
Model with no modification versus when the number
of extracted training patches is limited. The latter re-
duces running time by over 40%. Not only is the com-
putational cost significantly lower, but also the clas-
sification results improve (using t-statistic confirms
a significant difference at a 95% confidence level).
These results suggest that, without modification, over-
lap among patches contains redundant information
and decreases the separability among classes.
Measuring the information content of patches us-
ing entropy effectively removes patches that contain
little to no information relevant to a categorization.
The difference between the two accuracies, both with
overlapping patches constraint applied, but with and
without the use of entropy to select patches, show no
statistical significance. The system performs equally
well with 30% less features (without lowest-entropy
training patches), a significant saving of a computa-
tional cost. The running time with the entropy selec-
tion process is reduced by over 20% compared to the
system just using constrained patch overlap and by
over 50% compared to the original Standard Model.
7 CONCLUSIONS
The potential of an artificial vision system based on
biological principles is shown to be quite promising.
A crucial advantage is its independence of image seg-
mentation, a potentially high complexity processing
step. When experimenting with the Standard Model,
we were able to apply it, without re-tuning, and ob-
tain accuracies as good as or better than a traditional
approach, indicating good generalization capability.
To our knowledge, this is the first study that ap-
plied a biologically inspired model to a difficult un-
derwater imaging domain. Our experimental results
offer great promise for the analysis of large marine
image data sets collected from unique open-ocean
ecosystems. Automatic identification and quantifica-
tion of the plankton and particle components, coupled
with chemical and taxonomic composition analysis,
will facilitate the production of a refined carbon and
nitrogen budgets for this vast region, significant to our
understanding of how climate change affects the dy-
namics of ocean ecosystems.
ACKNOWLEDGEMENTS
This work was supported by NSF (OCE-0325167).
REFERENCES
Benfield, M. et al. (2007). RAPID: Research on automated
plankton identification oceanography. Oceanography.
Blaschko, M. et al. (2005). Automatic in situ identification
of plankton. In Proc. IEEE WACV, pages 79–86.
Blu, T. and Unser, M. (2003). A complete family of scaling
functions: The (α, τ)-fractional splines. In Proc. IEEE
Intl. Conf. Acoustics, Speech, and Signal Processing.
Byun, J. et al. (2006). Automated tool for the detection of
cell nuclei in digital microscopic images: Application
to retinal images. Molecular Vision, 12:949–960.
Culverhouse, P. et al. (2003). Do experts make mistakes?
A comparison of human and machine identification of
dinoflagellates. Marine Ecology Progress Series, 247.
De Valois, R. L. and De Valois, K. K. (1988). Spatial Vision.
Haralick, R. M. (1979). Statistical and structural approaches
to texture. Proceedings of the IEEE, 67(5):786–804.
Hays, G. C., Richardson, A. J., and Robinson, C. (2005).
Climate change and marine plankton. Trends in Ecol-
ogy and Evolution, 20(6):337–344.
Honjo, S., Manganini, S., Krishfield, R., and Francois, R.
(2008). Particulate organic carbon fluxes to the ocean
interior and factors controlling the biological pump:
A synthesis of global sediment trap programs since
1983. Progress In Oceanography, 76(3).
Hu, M. (1962). Visual pattern recognition by moment in-
variants. IEEE Trans. Information Theory, 8:179–187.
Hubel, D. and Wiesel, T. (1959). Receptive fields of single
neurons in the cat’s striate cortex. J. of Physiology.
Lisin, D. A. (2006). Image Classification with Bags of Local
Features. PhD thesis, Univ. of Mass., Amherst, MA.
Ojala, T., Pietik¨ainen, M., and M¨aenp¨a¨a, T. (2002). Mul-
tiresolution gray-scale and rotation invariant texture
classification with local binary patterns. IEEE PAMI.
Pilskaln, C. et al. (2005). High concentrations of marine
snow and diatom algal mats in the north pacific sub-
tropical gyre: Implications for carbon and nitrogen cy-
cles in the oligotrophic ocean. Deep Sea Research.
Ponce, J. et al. (2006). Dataset issues in object recognition.
In Towards Category-Level Object Recognition.
Ravela, S. (2003). On Multi-Scale Differential Features and
their Representations for Image Retrieval and Recog-
nition. PhD thesis, Univ. of Mass., Amherst, MA.
Riesenhuber, M. and Poggio, T. (1999). Hierarchical mod-
els of object recognition in cortex. Nat. Neurosci.
Serre, T., Wolf, L., and Poggio, T. (2005). Object recogni-
tion with features inspired by visual cortex. In Proc.
IEEE CVPR. http://cbcl.mit.edu/software-datasets.
Sieracki, C., Sierackia, M., and Yentsch, C. (1998). An
imaging-in-flow system for automated analysis of ma-
rine microplankton. Marine Ecology Progress Series.
VISAPP 2010 - International Conference on Computer Vision Theory and Applications
406