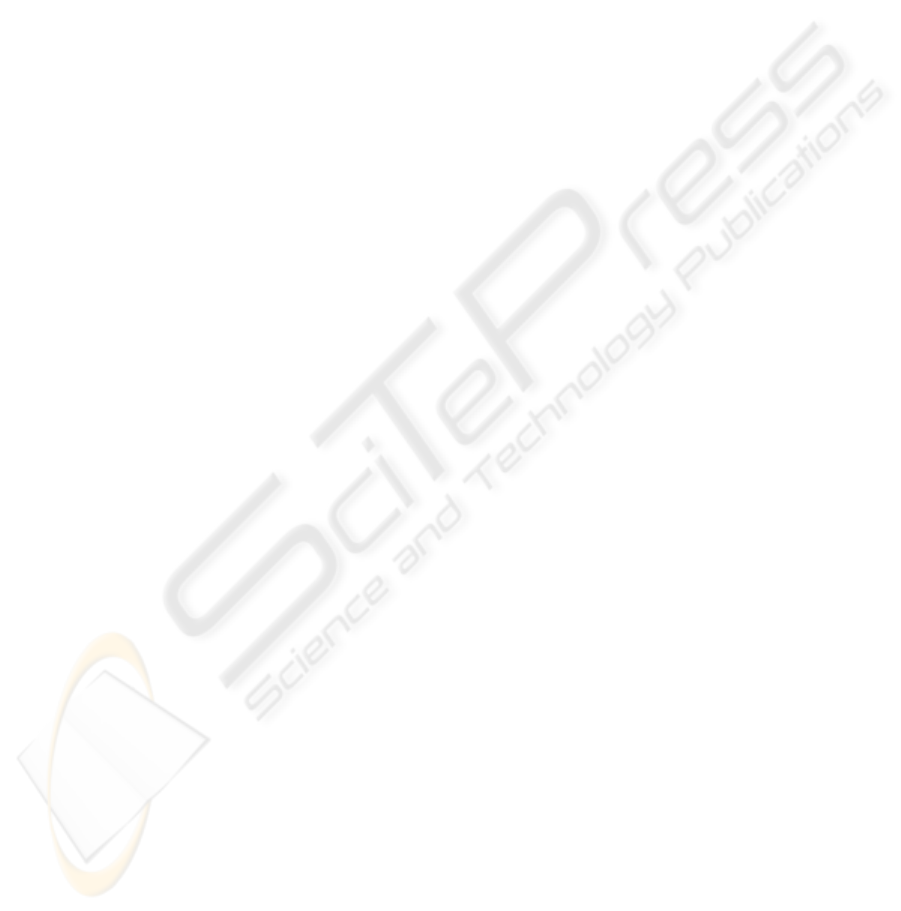
case), but in the end our coarse estimation of the
global illumination changes and the update of the set
of templates was enough to successfully track all the
sequences with a small loss of accuracy compared
to (Lef
`
evre and Odobez, 2009). Remember however
that using this recursive approach in our modeling of-
ten failed on longer sequences, which showed that it
was not really stable. When comparing our approach
to three other trackers in the literature, we notice that
it perform noticeably better than (Cascia et al., 2000)
on both datasets. The performances on the Uniform-
light dataset are comparable to those demonstrated
in (Morency et al., 2008; Xiao et al., 2003). How-
ever, we handle the much more challenging Varying-
light dataset while none of (Morency et al., 2008;
Xiao et al., 2003) demonstrated successfully on this
dataset.
5 CONCLUSIONS
In this paper we introduced a face tracking method
that uses information collected on the head sides to
robustly track challenging head movements. We ex-
tended an existing 3D face model so that the mesh
reaches the ears. In order to handle appearance vari-
ation (mainly due to head pose changes in practice),
our approach builds online a set of view-based tem-
plates. These two distinctive features were proved
to be particularly useful when the tracker has to deal
with extreme head poses like profile views. More-
over we showed the ability of our approach to follow
both natural and exaggerated facial actions. However
we are aware that one limitation of our system is that
there is no mechanism to recover from a potential fail-
ure. One solution would be to add a set of detectors
for specific points that could help to set the system
back on track.
ACKNOWLEDGEMENTS
This work was supported by the EU 6th FWP IST In-
tegrated Project AMIDA (Augmented Multiparty In-
teraction with Distant Access) and the NCCR Inter-
active Multimodal Information Management project
(IM2). We thank Vincent Lepetit for useful discus-
sions on the model.
REFERENCES
Ahlberg, J. (2001). Candide 3 - an updated parameterised
face. Technical Report LiTH-ISY-R-2326, Link
¨
oping
University, Sweden.
Cascia, M. L., Sclaroff, S., and Athitsos, V. (2000). Fast,
reliable head tracking under varying illumination: An
approach based on registration of texture-mapped 3d
models. In IEEE Trans. Pattern Analysis and Machine
Intelligence (PAMI), volume 22.
Chen, Y. and Davoine, F. (2006). Simultaneous tracking of
rigid head motion and non-rigid facial animation by
analyzing local features statistically. In British Ma-
chine Vision Conf. (BMVC), volume 2.
Cootes, T., Edwards, G., and Taylor, C. (1998). Active ap-
pearance models. In European Conf. Computer Vision
(ECCV), volume 2.
Cootes, T., Taylor, C., Cooper, D., and Graham, J. (1995).
Active shape models - their training and applica-
tion. Computer Vision and Image Understanding,
61(1):38–59.
DeCarlo, D. and Metaxas, D. (2000). Optical flow con-
straints on deformable models with applications to
face tracking. Int. Journal of Computer Vision,
38(2):99–127.
Dornaika, F. and Davoine, F. (2006). On appearance based
face and facial action tracking. In IEEE Trans. On Cir-
cuits And Systems For Video Technology, volume 16.
Gross, R., Matthews, I., and Baker, S. (2006). Active ap-
pearance models with occlusion. Image and Vision
Computing Journal, 24(6):593–604.
Jepson, A., Fleet, D., and El-Maraghi, T. (2003). Ro-
bust online appearance models for visual tracking.
IEEE Trans. Pattern Analysis and Machine Intelli-
gence (PAMI), 25(10):1296– 1311.
Lef
`
evre, S. and Odobez, J. (2009). Structure and appear-
ance features for robust 3d facial actions tracking. In
Int. Conf. on Multimedia & Expo.
Li, Y. (2004). On incremental and robust subspace learning.
Pattern Recognition, 37(7):1509–1518.
Matthews, I., Ishikawa, T., and Baker, S. (2004). The tem-
plate update problem. IEEE Trans. Pattern Analysis
and Machine Intelligence (PAMI), 26(6):810 – 815.
Morency, L.-P., Whitehill, J., and Movellan, J. (2008). Gen-
eralized adaptive view-based appearance model: In-
tegrated framework for monocular head pose estima-
tion. In IEEE Int. Conf. on Automatic Face and Ges-
ture Recognition (FG).
Tu, J., Tao, H., and Huang, T. (2009). Online updat-
ing appearance generative mixture model for mean-
shift tracking. Machine Vision and Applications,
20(3):163–173.
Vacchetti, L., Lepetit, V., and Fua, P. (2004). Stable real-
time 3d tracking using online and offline information.
IEEE Trans. Pattern Analysis and Machine Intelli-
gence (PAMI), 26(10):1385–1391.
Xiao, J., Baker, S., and Matthews, I. (2004). Real-time com-
bined 2d+3d active appearance models. In IEEE Conf.
Computer Vision and Pattern Recognition (CVPR).
Xiao, J., Moriyama, T., Kanade, T., and Cohn, J. (2003).
Robust full-motion recovery of head by dynamic tem-
plates and re-registration techniques. Int. Journal of
Imaging Systems and Technology, 13(1):85–94.
VISAPP 2010 - International Conference on Computer Vision Theory and Applications
230