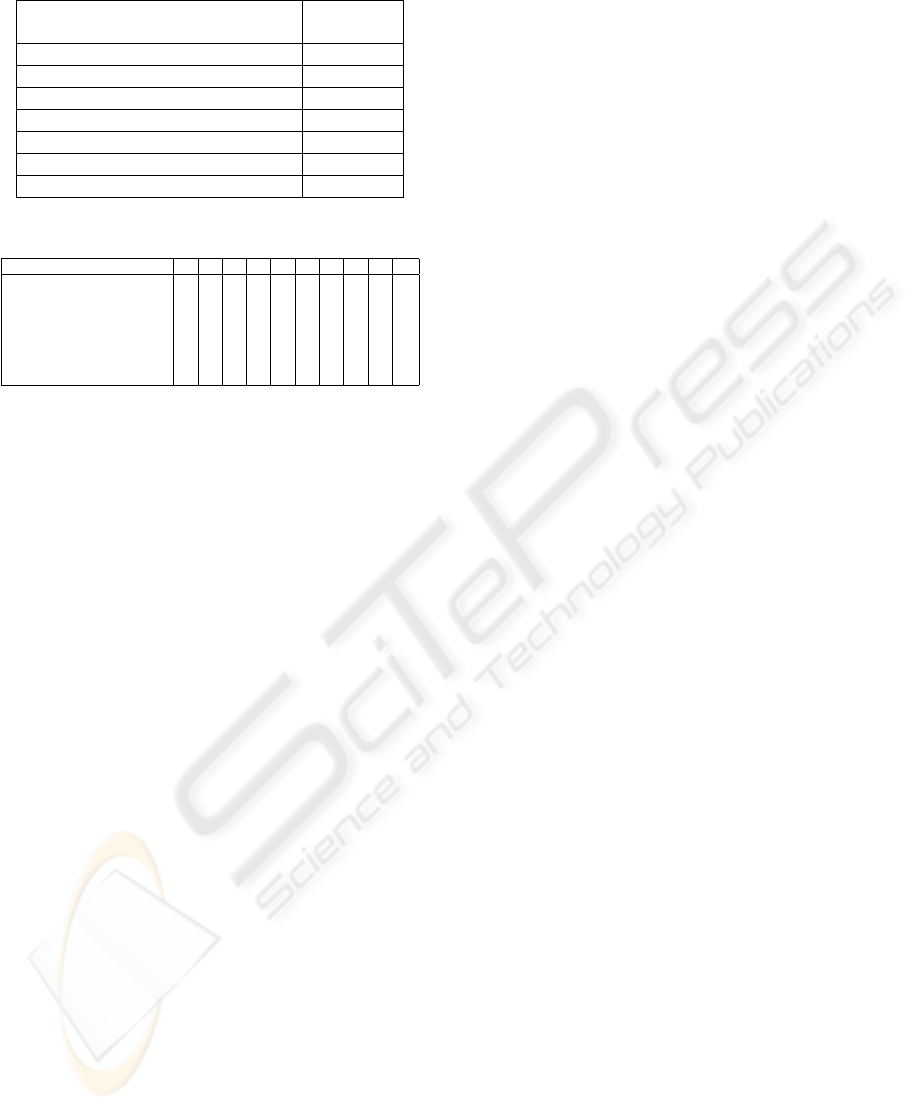
Table 1: Results comparison in MPEG-7 dataset.
Descriptors Retrieval
Rate
BAS (Arica and Vural, 2003) 82.37%
CFD 84.43%
DSW (Alajlan et al., 2007) 85.03%
IDSC+DP (Ling and Jacobs, 2007) 85.40%
DSW+Global (Alajlan et al., 2007) 87.23%
Graph Trans. (Yang et al., 2008) 91.00%
CFD+DistOpt 92.56%
Table 2: Results of experiments in Kimia dataset.
Descriptors 1
o
2
o
3
o
4
o
5
o
6
o
7
o
8
o
9
o
10
o
SC (Sebastian et al., 2004) 97 91 88 85 84 77 75 66 56 37
CFD 99 98 98 99 97 90 86 86 68 56
IDSC+DP 99 99 99 98 98 97 97 98 94 79
Shape Tree (Felzenszwalb
and Schwartz, 2007)
99 99 99 99 99 99 99 97 93 86
CFD + DistOpt 98 99 99 99 98 99 99 97 98 99
Graph Trans. 99 99 99 99 99 99 99 99 97 99
6 EXPERIMENTAL RESULTS
We conducted experiments in two image databases,
both widely used in the literature.
The MPEG-7 data set consists of 1400 silhouette im-
ages grouped into 70 classes. Each class has 20 dif-
ferent shapes. The retrieval rate is measured by the
number of shapes from the same class among the
40 most similar shapes. The following values were
defined for the parameters used in the distance op-
timization method: top
n
= 40, th
cohesion
= 70, and
top
nToAdd
= 10. Table 1 shows the results of sev-
eral descriptors using the MPEG-7 data set. The pro-
posed methods are named CFD and CFP + DistOpt.
CFP + DistOpt considers both the feature descrip-
tion approach and the proposed distance optimiza-
tion method. As it can be observed, CFP + DistOpt
has the best effectiveness performace when compared
with several well-known shape descriptors.
We also present experimental results on the Kimia
Data Set. This data set contains 99 shapes grouped
into nine classes. In this case, the following param-
eters’ values used in the distance optimization were
used: top
n
= 15, th
cohesion
= 7, and top
nToAdd
= 4.
The retrieval results are summarized as the number of
shapes from the same class among the first top 1 to
10 shapes (the best possible result for each of them
is 99). Table 2 lists the number of correct matches of
several methods. Again we observe that our approach
yields a very high retrieval rate, being superior to sev-
eral well-know shape descriptor.
7 CONCLUSIONS
In this paper, we have presented a shape description
approach that combines features extracted from ob-
ject contour. This paper has also presented a new op-
timization approach for reranking results in image re-
trieval systems. Several experiments were conducted
using well-known data sets. Results demonstrate that
the combination of both methods yield very high ef-
fectiveness performance when compared with impor-
tant descriptors recently proposed in the literature.
Future work includes the investigation of new fea-
tures to be incorporated into the proposed description
framework. We also plan to use the distance optimiza-
tion method with color and texture descriptors.
ACKNOWLEDGEMENTS
Authors thank CAPES, FAPESP and CNPq for finan-
cial support.
REFERENCES
Adamek, T. and OConnor, N. E. (2004). A multiscale
representation method for nonrigid shapes with a sin-
gle closed contour. IEEE Trans. Circuits Syst. Video
Techol., 14 i5:742–753.
Alajlan, N., El Rube, I., Kamel, M. S., and Freeman, G.
(2007). Shape retrieval using triangle-area represen-
tation and dynamic space warping. Pattern Recogn.,
40(7):1911–1920.
Arica, N. and Vural, F. T. Y. (2003). Bas: a perceptual shape
descriptor based on the beam angle statistics. Pattern
Recogn. Lett., 24(9-10):1627–1639.
Felzenszwalb, P. F. and Schwartz, J. D. (2007). Hierarchical
matching of deformable shapes. CVPR, pages 1–8.
Ling, H. and Jacobs, D. W. (2007). Shape classification
using the inner-distance. PAMI, 29(2):286–299.
Sebastian, T. B., Klein, P. N., and Kimia, B. B. (2004).
Recognition of shapes by editing their shock graphs.
PAMI, 26(5):550–571.
Yang, X., Bai, X., Latecki, L. J., and Tu, Z. (2008). Improv-
ing shape retrieval by learning graph transduction. In
ECCV, pages 788–801.
VISAPP 2010 - International Conference on Computer Vision Theory and Applications
202