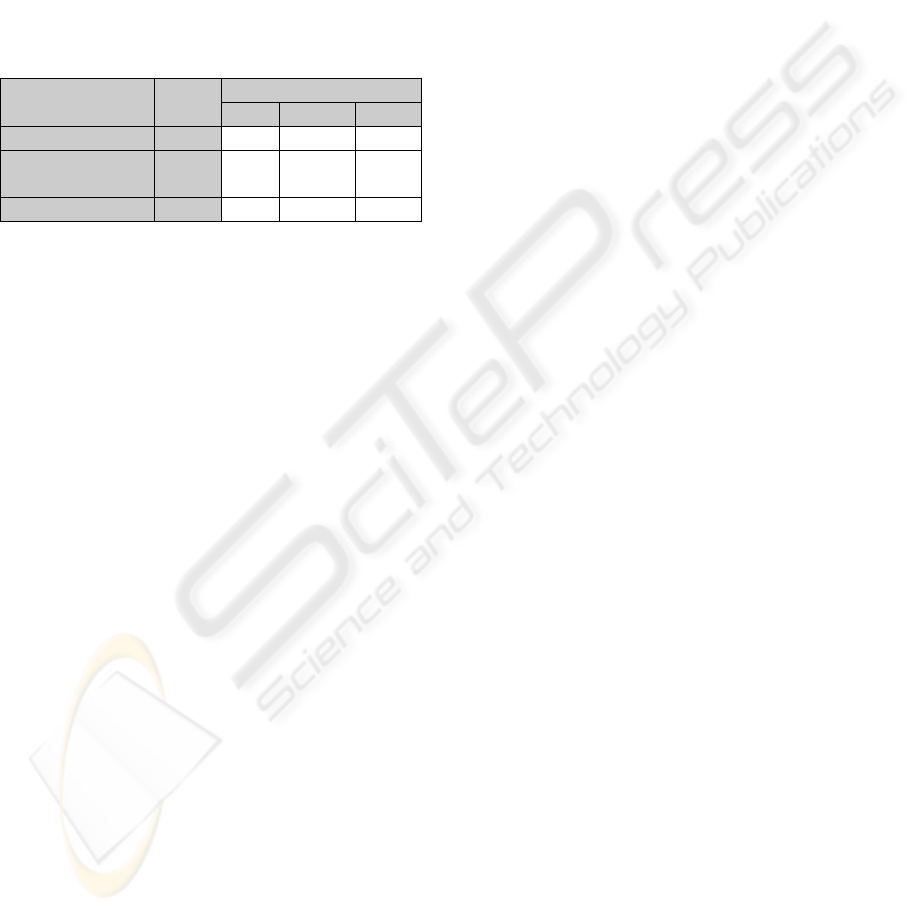
We compared our results with Cerezo and
Hupont, (2006). They used 10 characteristic MPEG4
feature points to extract emotional information to
detect six basic emotions. They worked on static
images and manually selected facial points. They
tested Hammal’s method (Hammal, Couvreur,
Caplier and Rombaut, 2005) on FG-NET dataset.
Although the evaluation criteria and feature
extraction method are not the same (manual vs.
automatic), Table 2 shows general information about
results achieved on FG-NET dataset.
Table 2: Previous studies on FG-NET dataset.
Method
Feature
Extract
Accuracy in %
Happy Surprise Neutral
Hammal’s method Man. 87,2 84,4 88,0
Cerezo and
Hupont,2006
Man.
36,8 57,8 100,0
Our method Auto 74,1 22,2 88,9
In terms of speed, our hybrid approach runs at
28fps on Intel Core2Duo 2.8 GHz laptop for 26fps
mpeg sized videos. In addition current prototype
delivers 24.5fps for webcam video frames of size
640×480. Therefore the suggested approach is
suitable for real-time processing.
4 CONCLUSIONS AND FUTURE
WORK
In this paper, we proposed a hybrid approach for
facial feature detection for emotion recognition in
video. Our system is detects seven facial feature
points (eyebrows, pupils, nose, and corners of
mouth) from grayscale images.
Experimental results showed that our system
works well on faces with no occlusions thus we get
acceptable emotion recognition results. On the other
hand, different occlusions on facial area slightly
affect the performance of the system.
As future work, we are planning to detect finer
locations for eyebrows and radius of the pupillary
area in terms of feature extraction and planning to
work on hard cases (hair occlusion, etc.). In case of
eyebrows, the shape of the eyebrow will give useful
information about different emotion.
ACKNOWLEDGEMENTS
This study is supported by the Multimodal Interfaces
for Disabled and Ageing Society (MIDAS) ITEA 2
– 07008 project.
REFERENCES
Cerezo, E. and Hupont, I., (2006). Emotional Facial
Expression Classification for Multimodal User
Interfaces. LNCS, (Vol. 4069, pp. 405-413).
Cootes, T. F., Edwards, G. J. and Taylor, C. J. (1998).
Active appearance models. In H.Burkhardt and B.
Neumann, editors, 5th European Conference on
Computer Vision, (Vol. 2, 484–498), Springer, Berlin.
Ekman, P. and Friesen, W. V. (1982). Felt, false, and
miserable smiles. Journal of Nonverbal Behavior, 6,
238–252.
Hammal, Z., Couvreur, L., Caplier, A. and Rombaut, M.
(2005). Facial Expressions Recognition Based on the
Belief Theory: Comparison with Different Classifiers.
In Proceedings of 13th International Conference on
Image Analysis and Processing, Italy.
Izard, C. E. (1991). The psychology of emotions. New
York: Plenum Press.
Kass, M., Witkin, A. and Terzopoulos, D. (1987). Snake:
Active Contour Model, International Journal of
Computer Vision. (Vol. 1, pp. 321-331).
Milborrow, S. and Nicolls, F. (2008). Locating facial
features with an extended active shape model. In:
Forsyth, D., Torr, P., Zisserman, A. (eds.) ECCV
2008, Part IV. LNCS, (Vol. 5305, pp.504–513).
Springer, Heidelberg.
Rowley, H. A., Baluja, S. and Kanade T. (1998). Neural
Network-Based Face Detection. IEEE Transactions on
Pattern Analysis and Machine Intelligence, (Vol. 20,
p. 23-38), http://vasc.ri.cmu.edu/NNFaceDetector
Savran, A., Alyüz, N., Dibeklioğlu, H., Çeliktutan, O.,
Gökberk, B., Sankur, B. and Akarun L. (2008).
Bosphorus Database for 3D Face Analysis, The First
COST 2101 Workshop on Biometrics and Identity
Management (BIOID 2008), Roskilde University,
Denmark.
Viola, P. and Jones, M. (2001). Rapid object detection
using a boosted cascade of simple features. In
Proceedings of Computer Vision and Pattern
Recognition, (Vol. 1, pp. 511–518).
Wallhoff, F. (2006). FG-NET Facial Expressions and
Emotion Database. Technische Universität München.
Retrieved from: http://www.mmk.ei.tum.de/~waf/
fgnet/feedtum.html.
VISAPP 2010 - International Conference on Computer Vision Theory and Applications
412