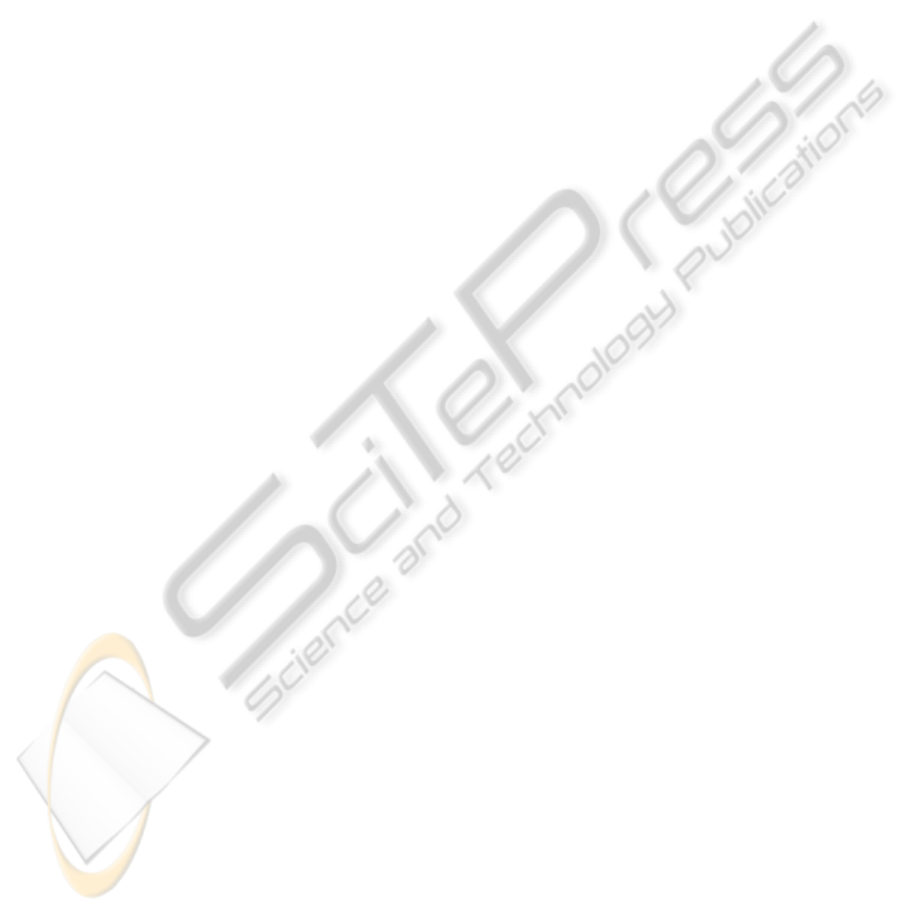
presented some essential theorems in order to
identify the defining hyperplanes of constant returns
to scale (CRS) technology. These theorems enable
us to recognize whether a hyperplane obtained by
the optimal solution of the multiplier form of CCR
model is a defining hyperplane.
Furthermore, one of the most important task of
defining hyperplanes of production possibility set is
sensitivity analysis that enable us to determine the
amounts of perturbations of data that can be
tolerated by a DMU on efficient frontier before
becoming inefficient. Also, we can utilize the
concept defined in this paper in order to evaluate the
efficiency of DMUs by using the defining
hyperplanes of PPS, which efficient DMUs are on
them.
Some of the characteristics presented in this
paper are more conceptual, however others are more
practical. Furthermore, the conceptual point of view
of theorems presented in this paper, enable us to
interpret the characteristics of defining hyperplanes
of CRS technology. Although some of the theorems
are so practical and one can easily utilize them in
practice. Not only, the conceptual point of view of
theorems is essential and is so useful to
interpretation of defining hyperplanes of CRS
technology, but also the practical point of view of
theorems is a necessity and enable us to utilize the
characteristics in practice.
The aim of this paper is to use the conceptual
point of view of some parts of topology and convex
analysis and a combination of them with DEA to
present some conceptual and practical characteristics
in order to determine when a hyperplane of PPS is a
defining hyperplane. The main idea of this paper is
based on the geometrical interpretation of efficient
facets of the highest dimension of the frontier that
the DMU under assessment contributes to span. In
particular a defining hyperplane is a full dimensional
efficient facet (FDEF) and may be found in Olesen
and Peterson (2003). These geometrical
interpretations enable us to establish the presented
characteristics. Some of these characteristics are
conceptual that we will not be able to utilize them in
practice. Although, we use these conceptual
characteristics in order to establish some practical
characteristics that one may easily utilize them in
practice.
The sections of this paper are organized as
follows. In the next section, Section 2, we provide
additional background of our paper. In Section 3, we
give basic concepts of some parts of topology,
convex analysis and DEA models. Section 4
investigates the characteristics of defining
hyperplanes of constant returns to scale (CRS)
technology. In Section 5, we present an example to
illustrate the characteristics.
2 BACK GROUND
As previously noted, this paper is dealt with the
characteristics of defining hyperplanes of CRS
technology in DEA. These defining hyperplanes
play an important role in DEA as previously
mentioned.
In this paper, we restrict attention to geometrical
differences between defining hyperplanes of CRS
technology and those supporting hyperplanes of
CRS technology that are not defining. As we know,
these two kinds of hyperplanes play a crucial role in
DEA, since they are generally utilized to determine
different types of concepts such as efficiency, bench
mark DMUs, rates of substitution and
transformation, returns to scale, sensitivity analysis
and etc.
The main idea of this paper is based on
geometrical interpretation of defining hyperplanes of
CRS technology. In order to state a geometrical
characteristics of defining hyperplanes of CRS
technology, we use a combination of different kinds
of concepts such as interior points of a set, an
-
neighborhood around a point and geometrical
interpretation of CRS technology efficient frontier to
state a specific relation between the dimension of
intersection of each defining hyperplanes with the
production possibility set (PPS) of CRS technology
that we use this characteristics to show the truth of
others stated characteristics.
Secondly, we utilize a model proposed by
Cooper et al. (2007) to determine a hyperplane that
is binding at the maximum number of extreme
efficient units. With utilizing the abovementioned
hyperplane namely
∗
, we define a created DMU
obtained by center of gravity of extreme efficient
units that the abovementioned hyperplane
∗
is
binding at them. Eventually, a set of feasible
directions obtained by connecting the created DMU
to each extreme efficient unit that the hyperplane
∗
is binding at them has been defined to present a
practical characteristic.
ICINCO 2010 - 7th International Conference on Informatics in Control, Automation and Robotics
66