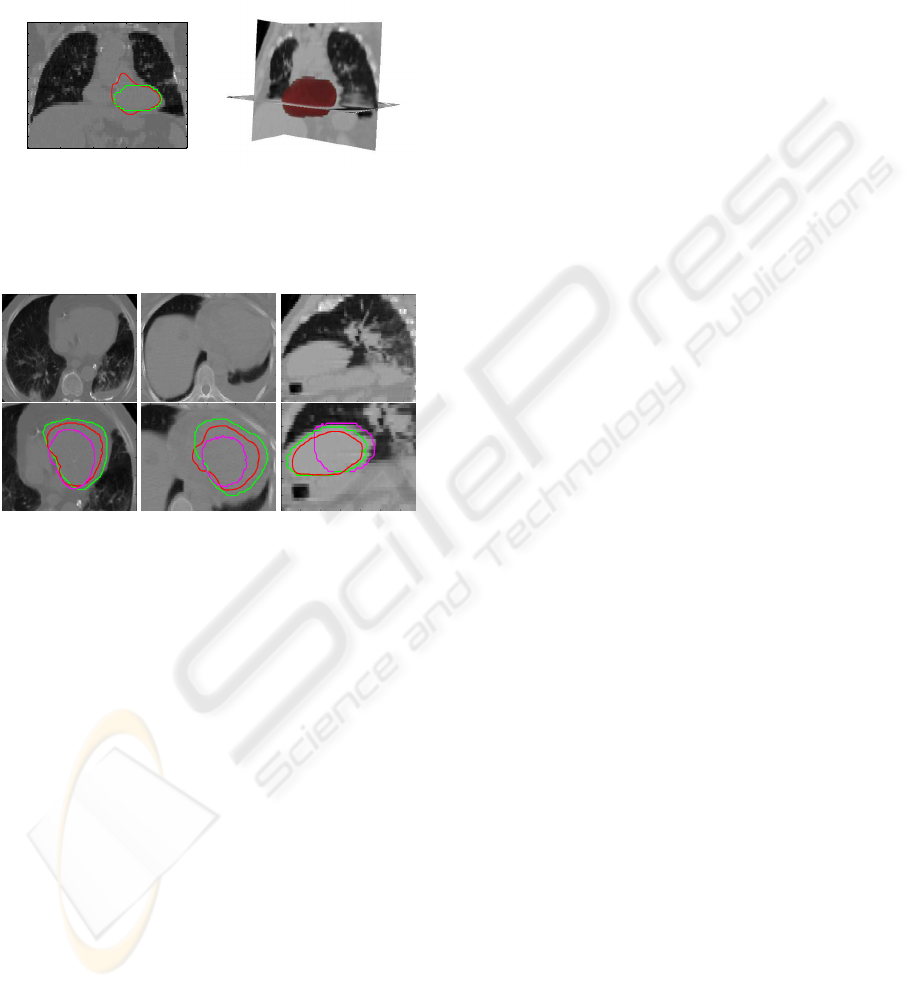
cept for the heart 4 (Figure 8 column 2), the speci-
ficity index is generally higher than the sensitivity. It
means that our automatic segmentation often provides
a larger region than the manual one (it is mainly due
to the fact that a small part of the aorta is often in-
cluded in the segmentation of the heart, as illustrated
in Figure 7(a).
50 100 150 200
5
10
15
20
25
30
35
40
45
50
55
(a) (b)
Figure 7: (a) Automatic segmentation (in red) includes
small part of the aorta (green: manual segmentation). (b)
A 3D view of a whole heart segmentation.
Figure 8: Examples of segmentation results. First row:
original image, second row: image superimposed with seg-
mentations (green expert segmentation, magenta Moreno et
al segmentation, red our automatic segmentation).
5 CONCLUSIONS
We have adapted a fuzzy region competion frame-
work for the segmentation of the heart in non-contrast
CT images by adding a shape constraint. Shape infor-
mations was encoded with Legendre moments. Since
we work with CT images (which are calibrated), we
use hard a priori on the image intensity. The ini-
tialization is performed semi-automatically using a
spherical approximation of the heart. Several tests
on clincal cases provide satisfying results. In par-
ticular, the shape constraint allows us to achieve a
good separation between the heart and surrounding
organs (liver, aorta), improving the initial fuzzy re-
gion competition model. When compared to another
method (Moreno et al., 2008) using structural knowl-
edge (but no shape information) the results are also
improved. This framework could be extended in a se-
quential way to segment other organs in the thorax
like the aorta.
ACKNOWLEDGEMENTS
This work was partially funded by the Medicen Pˆole
de Comp´etitivit´e within the Miniara project.
REFERENCES
Chan, T. F., Esedoglu, S., and Nikolova, M. (2005). Finding
the global minimum for binary image restoration. In
ICIP, pages I: 121–124.
Chan, T. F. and Vese, L. A. (2001). Active contours with-
out edges. IEEE Trans. Image Processing, 10(2):266–
277.
Cremers, D., Rousson, M., and Deriche, R. (2007). A re-
view of statistical approaches to level set segmenta-
tion: Integrating color, texture, motion and shape. In-
ternational Journal of Computer Vision, 72(2):195–
215.
Ecabert, O., Peters, J., and etal (2008). Automatic model-
based segmentation of the heart in CT images. IEEE
Trans. Medical Imaging, 27(9):1189–1201.
Foulonneau, A., Charbonnier, P., and Heitz, F. (2006).
Affine-invariant geometric shape priors for region-
based active contours. IEEE Trans. Pattern Analysis
and Machine Intelligence, 28(8):1352–1357.
Gastaud, M., Barlaud, M., and Aubert, G. (2004). Combin-
ing shape prior and statistical features for active con-
tour segmentation. IEEE Trans. Circuits and Systems
for Video Technology, 14(5):726–734.
Hosny, K. M. (2007). Exact Legendre moment compu-
tation for gray level images. Pattern Recognition,
40(12):3597–3605.
Leventon, M. E., Grimson, W. E. L., and Faugeras, O. D.
(2000). Statistical shape influence in geodesic active
contours. In CVPR, pages I: 316–323.
Moreno, A., Takemura, C. M., Colliot, O., Camara, O., and
Bloch, I. (2008). Using anatomical knowledge ex-
pressed as fuzzy constraints to segment the heart in
CT images. Pattern Recognition, 41(8):2525–2540.
Mory, B. and Ardon, R. (2007). Fuzzy region competi-
tion: A convex two-phase segmentation framework.
In Scale Space and Variational Methods in Computer
Vision, pages 214–226.
Paragios, N. and Deriche, R. (1999). Geodesic active con-
tours for supervised texture segmentation. In CVPR,
pages II: 422–427.
Poupon, F., Mangin, J. F., Hasboun, D., Poupon, C., and
Magnin, I. (1998). Multi-object deformable templates
dedicated to the segmentation of brain deep structures.
In Medical Image Computing and Computer-Assisted
Intervention - MICCAI 1998, pages 187–196.
Rose, J.-L., Revol-Muller, C., Charpigny, D., and Odet, C.
(2009). Shape prior criterion based on Tchebichef mo-
ments in variational region growing. In ICIP.
Teague, M. R. (1980). Image analysis via the general theory
of moments. Journal of the Optical Society of Amer-
ica, 70(8):920–930.
VISAPP 2010 - International Conference on Computer Vision Theory and Applications
214