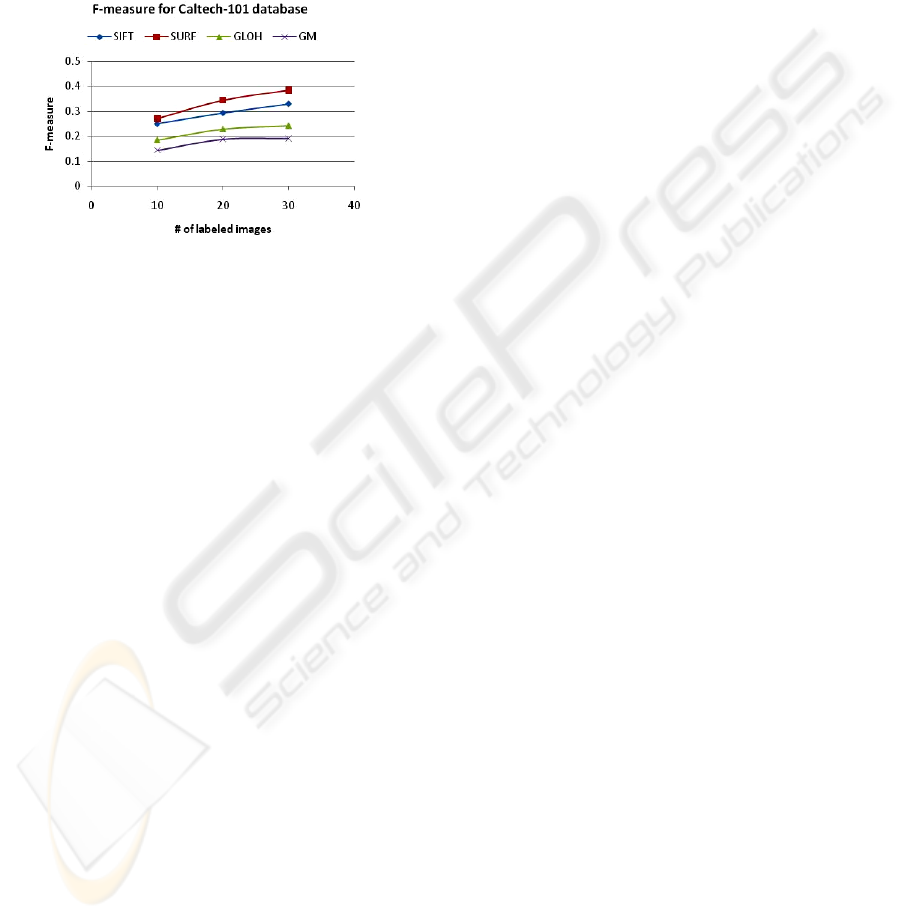
Here we considered only best four LIFs obtained
from experiments on ETH-80. Figure 3 shows the F-
measures for all the classes. As before, generally the
performance is improved with increased number of
labelled objects. Individually the performance for
SURF is the best followed by SIFT, GLOH, and GM
as well. However, the F-measure drops sharply with
respect to ETH-80 dataset (e.g. to 0.38 from 0.89 for
experiments with 30 labelled images).
Figure 3: F-measures for Caltech-101 database.
5 CONCLUSIONS
In this paper, we have proposed a useful method in
evaluation of existing local image features for object
class recognition. The proposed method is based on
a simple nearest neighbor method. In this work,
eight prominent and frequently used local features
are evaluated using two popular databases. We have
used F-measure criterion to analyze the performance
of the LIFs
It is found that average individual performance
for SURF and SIFT are quite satisfactory (with F-
measure of 0.89 and 0.84 respectively) on ETH-80
database. They outperform the individual performers
of different global features as considered in (Leibe
and Schiele, 2003). Here we used considerably
lower number of labelled images. GLOH and GM
features are the next best features for object class
recognition. However, on Caltech-101 database this
performance drops sharply (e.g. to 0.29 from 0.84
for SIFT). This may caused by different reasons.
Most obvious among them is that without any
quantization the feature space gets more crowded
with the increase of object class and thereby the
chance of misclassification increases. However, it
the evident that we need to extract more
complementary image features or alternatively to
combine several features for better performance of
object class recognition.
ACKNOWLEDGEMENTS
The research presented in this paper is a part of
A*STAR Science \& Engineering Research Council
grant 072 134 0052. The financial support of SERC
is gratefully acknowledged.
REFERENCES
Asbach, M., Hosten, P. and Unger, M. (2008). An
Evaluation of Local Features for Face Detection and
Localization. Ninth Int. Workshop on Image Analysis
for Multimedia Interactive Services.
Bay, H., Ess, A., Tuytelaars, T. and Gool, L. V. (2008).
Speeded-up Robust Features (SURF). Computer
Vision and Image Understanding 110, 346-359
Boiman, O., Shechtman, E. and Irani, M. (2008). In
Defense of Nearest-Neighbor Based Image
Classification. IEEE Conf. on CVPR, 1 - 8.
Freeman, W. and Adelson, E. (1991). The Design and Use
of Steerable Filters. IEEE Transactions on Pattern
Analysis and Machine Intelligence: 13, 891-906.
Leibe, B. and Schiele, B. (2003). Analyzing Appearance
and Contour Based Methods for Object
Categorization. IEEE Conf. on CVPR, Wisconsin.
Li, J. and Allinson, N. M. (2008). A Comprehensive
Review of Current Local Features for Computer
Vision. Neurocomputing, 71, 1771-1787.
Lowe, D. G. (2004). Distinctive Image Features from
Scale-Invariant Keypoints. IJCV: 60, 91-110.
Mikolajczyk, K., Leibe, B. and Schiele, B. (2006).
Multiple Object Class Detection with a Generative
Model. IEEE Conf. on CVPR, 26 - 36
Mikolajczyk, K. and Schmid, C. (2005). A Performance
Evaluation of Local Descriptors. IEEE Transactions
on Pattern Analysis and Machine Intelligence: 27,
1615-1630.
Mikolajczyk, K., Tuytelaars, T., Schmid, C., Zisserman,
A., Matas, J., Schaffalitzky, F., Kadir, T. and Gool, L.
V. (2005). A Comparison of Affine Region Detectors
IJCV: 65, 43-72
Mindru, F., Tuytelaars, T., Gool, L. V. and Moons, T.
(2004). Moment Invariants for Recognition under
Changing Viewpoint and Illumination. Computer
Vision and Image Understanding: 94, 3-27.
Reiss, T. H. (1993). Recognizing Planar Objects Using
Invariant Image Features, Springer-Verlag.
Stark, M. and Schiele, B. (2007). How Good Are Local
Features for Classes of Geometric Objects. IEEE 11th
International Conference on Computer Vision, 1 - 8.
Zhang, H., Berg, A. C., Maire, M. and Malik, J. (2006).
Svm-Knn: Discriminative Nearest Neighbor
Classification for Visual Category Recognition. IEEE
Conf. on CVPR, 2126- 2136.
Zhang, J. and Marszalek, M. (2006). Local Features and
Kernels for Classification of Texture and Object
Categories: A Comprehensive Study. IJCV: 73, 213 -
238.
AN EVALUATION OF LOCAL IMAGE FEATURES FOR OBJECT CLASS RECOGNITION
523