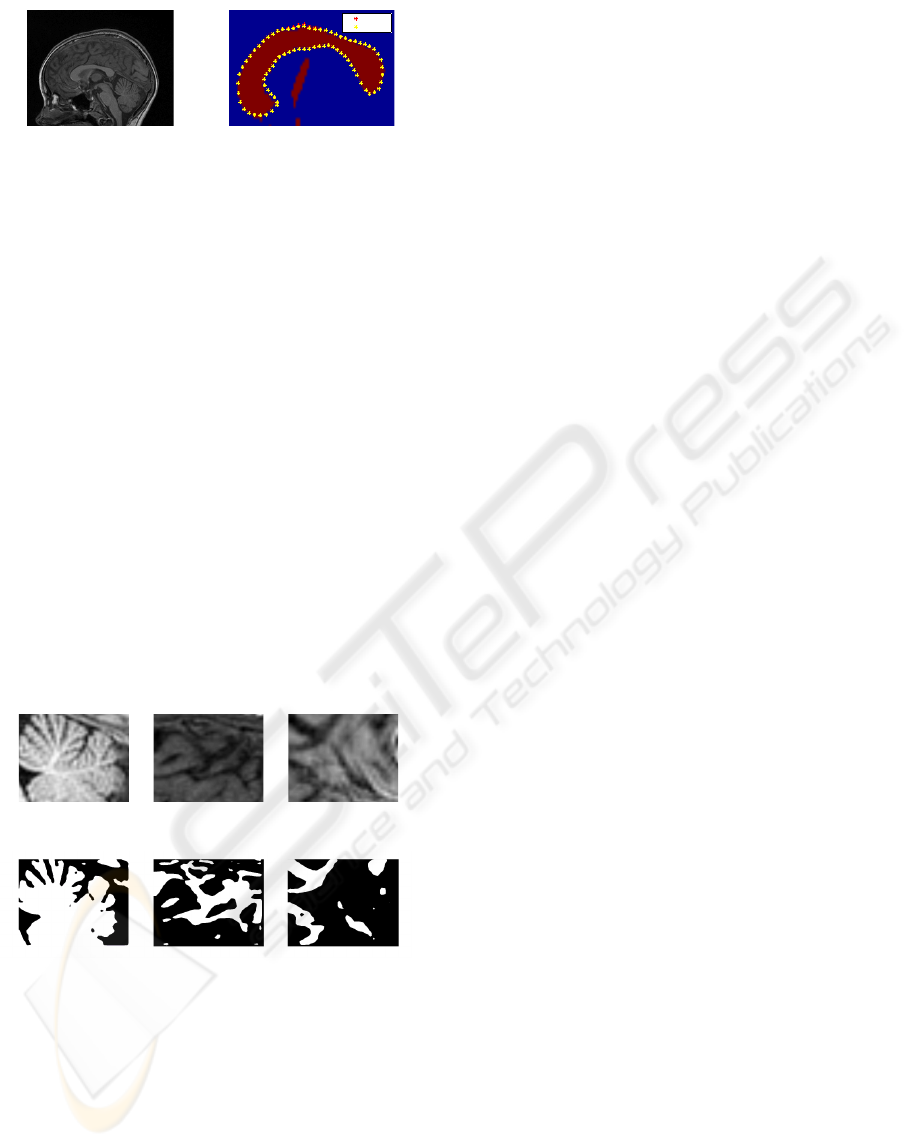
(a) MRI
(b) segmentation
Figure 10: (a)The midsagittal slice from an MRI of a head.
(b) This figure show the result of the segmentation of fig
10(a) using the method proposed in this paper. The red dots
are the segmentation achieved by the AAM and the yellow
the manual segmentation. The read part of the image is the
segmentation with the our method. This result show how
efficient this algorithm is for local segmentation.
property and us a smaller window, outliers become
more significant and we get better segmentation. By
switching to a more local neighborhood we get a im-
provement of almost 3% to 0.880 and the difference
is very significant (p << 0.01) using a paired t-test.
As a last test we separated the white and gray mat-
ter. The results are a little greedy, some gray matter is
classified as white matter. This is due to the fact that
the two density functions are somewhat overlapping
and that the dark regions actually is a 3 class segmen-
tation problem making it non-binary. Some improve-
ment can be obtained by adjusting the window size
and the p-value. The results in figure 11 were made
with p = 0.01 and a window of 40 × 40 with a sam-
pling resolution of 0.1 pixels.
(a) Image (b) Image (c) Image
(d) segmentation (e) segmentation (f) segmentation
Figure 11: Some results on segmenting the white matter.
The results are a little greedy including some gray matter.
5 SUMMARY AND
CONCLUSIONS
We have presented a local adaptive method for binary
segmentation. The methods has successfully been
tested on particle images for particle segmentation,
calibration images and and midsagittal slices of MRI
for segmentation of corpus callosum and gray mat-
ter white matter segmentation. The method is very
robust with respect to changes in intensity across the
image and statistically characterizes the resulting seg-
mentation. We have shown that compared to manual
segmentation of the Corpus Callosum we can achieve
a dice coefficient of 0.88 on using a mosaic of 5
patches. The method is directly extendable to 3D,
other types of distribution. The hypothesis test and
FDR should be extended to higher dimensions that
the one dimensional case discussed here and tested on
several other types of images. furthermore the algo-
rithm should be implemented such that it can handle
multiple classes and segment a whole image at once.
REFERENCES
Bretzner, L. and Lindeberg, T. (1998). Feature tracking with
automatic selection of spatial scales. Computer Vision
and Image Understanding, 71(3):385–392.
Darkner, S., Paulsen, R. R., and Larsen, R. (2007). Analy-
sis of deformation of the human ear and canal caused
by mandibular movement. In Medical Image Com-
puting and Computer Assisted Intervention MICCAI
2007, pages 801–8, B. Brisbane, Australia, Springer
Lecture Notes.
Efron, B. (2004). Large-scale simultaneous hypothesis test-
ing: the choice of a null hypothesis. Journal of the
American Statistical Association, 99(465):96–104.
Hastie, T., Tibshirani, R., and Friedman, J. (2001). The Ele-
ments of Statistical Learning: Data Mining, Inference,
and Prediction. Springer-Verlag.
Ng, H. (2006). Automatic thresholding for defect detection.
Pattern Recognition Letters, 27(14):1644–1649.
Otsu, N. (1975). A threshold selection method from gray-
level histograms. Automatica, 11:285–296.
Ryberg, C., Stegmann, M. B., Sj¨ostrand, K., Rostrup, E.,
Barkhof, F., Fazekas, F., and Waldemar, G. (2006).
Corpus callosum partitioning schemes and their effect
on callosal morphometry.
Sezan, M. (1990). A peak detection algorithm and its
application to histogram-based image data reduction.
Computer Vision, Graphics, and Image Processing,
49(1):51.
Sezgin, M. and Sankur, B. (2004). Survey over image
thresholding techniques and quantitative performance
evaluation. Journal of Electronic Imaging, 13(1):146–
168.
Sørensen, T. (1948). A method of establishing groups of
equal amplitude in plant sociology based on similarity
of species content and its application to analyses of the
vegetation on Danish commons. Biologiske Skrifter,
(5):1–34.
Stockman, G. and Shapiro, L. (2001). Computer Vision.
Prentice Hall PTR, Upper Saddle River, NJ, USA.
VISAPP 2010 - International Conference on Computer Vision Theory and Applications
220