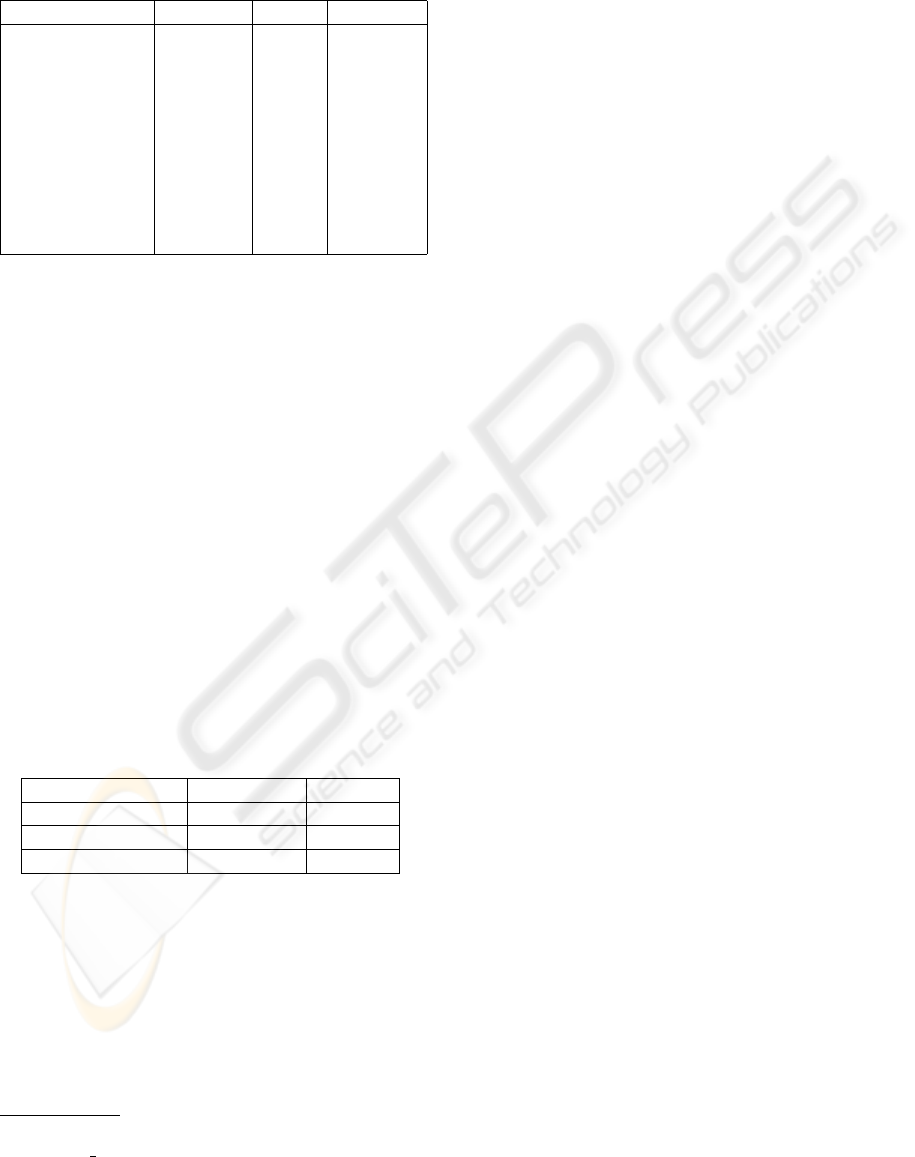
Table 1: Results of the classification experiments by test-
ing different PDD settings and SVM kernels. Best results
were achieved with 5x5 blocks, a search window of 10 and
a linear svm kernel.
Precision Recall Accuracy
3x3 linear Ω 5 0.575 0.993 0.583
3x3 rbf Ω 5 0.562 1.000 0.562
3x3 linear Ω 10 0.644 0.913 0.667
3x3 rbf Ω 10 0.615 0.941 0.636
5x5 linear Ω 5 0.527 0.466 0.465
5x5 rbf Ω 5 0.570 0.634 0.526
5x5 linear Ω 10 0.816 0.658 0.725
5x5 rbf Ω 10 0.659 0.589 0.598
7x7 linear Ω 5 0.563 0.550 0.507
7x7 rbf Ω 5 0.534 0.640 0.485
Figure 6 illustrates the foreground segmentation
and detection results for some sequences
2
. The se-
vere illumination changes and bad contrast hinder the
foreground segmentation as can be seen in Figure 6C.
The proposed method is quite robust to that difficulty
since, while the contour of the person is disturbed, we
use the periodicity observed in the whole bounding
box.
We experimented also with persons viewed from
different angles. Moving away and towards the cam-
era has another pattern as moving side-ways. Thus the
method produces less robust results since the SVM
was trained with people moving parallel to the cam-
era (Figure 6D).
Figure 6F shows a wrong classification result. In
this sequence the person is swinging the handbag in
the same frequency and amplitude as its leg so the re-
sulting pattern is the same as a pattern learned with no
baggage. Therefore an incorrect decision was taken.
Table 2: Detection carried objects result on 25 sequences.
no Baggage Baggage
total 11 14
false detected 0 2
correctly detected 11 12
4 CONCLUSIONS
It this paper we proposed a novel method for detect-
ing people carrying objects. Although the results ob-
tained in our first experiments are very promising, the
system can be further improved in many directions.
A more elaborated background model can allow the
2
available at http://www.nue.tu-berlin.de/menue/ mitar-
beiter/tobias
senst/
achievement of more precise bounding boxes. Se-
mantic information could be used to determine the
start and endpoint for the trajectories for each bound-
ing box. Furthermore, the computation load of the
similarity plot can be reduced by using other mea-
sures based for instance on PCA.
REFERENCES
Abdelkader, C. B. and Davis, L. (2002). Detection of peo-
ple carrying objects: A motion-based recognition ap-
proach. Automatic Face and Gesture Recognition,
IEEE International Conference on, pages 378–383.
Abdelkader, C. B., Davis, L., and Cutler, R. (2002). Motion-
based recognition of people in eigengait space. Auto-
matic Face and Gesture Recognition, IEEE Interna-
tional Conference on, pages 267–272.
Damen, D. and Hogg, D. (2008). Detecting carried objects
in short video sequences. In ECCV ’08: Proceedings
of the 10th European Conference on Computer Vision,
pages 154–167, Berlin, Heidelberg. Springer-Verlag.
Ekinci, M. and Aykut, M. (2007). Human gait recognition
based on kernel pca using projections. J. Comput. Sci.
Technol., 22(6):867–876.
Haritaoglu, I., Cutler, R., Harwood, D., and Davis, L. S.
(1999). Backpack: Detection of people carrying ob-
jects using silhouettes. In Computer Vision and Image
Understanding, pages 102–107.
Haritaoglu, I., Harwood, D., and Davis, L. S. (2000). W4:
Real-time surveillance of people and their activities.
IEEE Transactions on Pattern Analysis and Machine
Intelligence, 22(8):809–830.
Horprasert, T., Harwood, D., and Davis, L. S. (2000). A ro-
bust background subtraction and shadow detection. In
In Proceedings of the Asian Conference on Computer
Vision.
Pathan, S., Al-Hamadi, A., Senst, T., and Michaelis, B.
(2009). Multi-object tracking using semantic analy-
sis and kalman filter. In ISPA ’09: Proceedings of 6th
International Symposium on Image and Signal Pro-
cessing and Analysis, pages 271–276.
Stauffer, C. and Grimson, W. E. L. (1999). Adaptive back-
ground mixture models for real-time tracking. In
CVPR, pages 2246–2252.
Webb, D., Tuttle, R. H., and Baksh, M. (1994). Pendular
activity of human upper limbs during slow and normal
walking. American Journal of Physical Anthropology,
93:477–489.
TOWARDS DETECTING PEOPLE CARRYING OBJECTS A Periodicity Dependency Pattern Approach
529