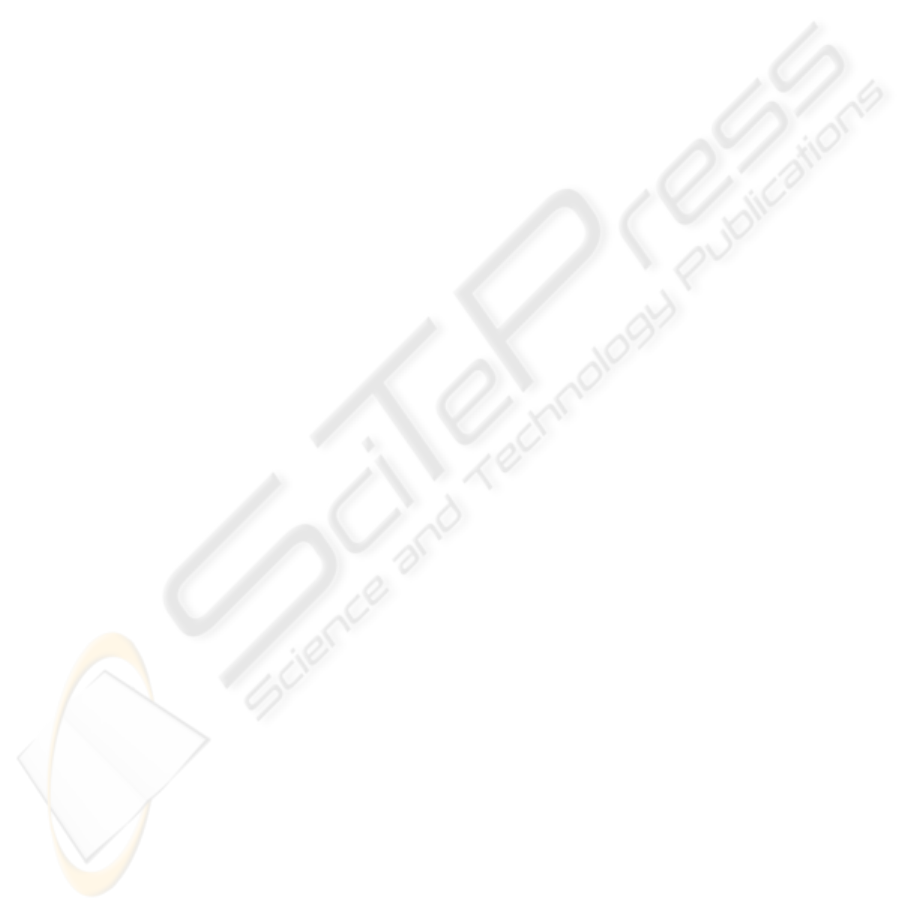
hicles being spatially close together even if the corre-
sponding regions in the disparity map are intercon-
nected. The method breaks such a horizontal link
apart and present vehicles are extracted in combina-
tion with the road profile. The combination of these
two iterative stages has shown to be an excellent de-
tection technique.
These algorithms were tested on both stereo vi-
sion systems in urban traffic and autobahn scenarios.
The stereo sensor B has shown a better performance,
which goes back to the larger baseline. The smaller
baseline of the system A would demand a more ag-
gressive filtering stage for outlier removal due to the
depth resolution of the stereo configuration. This
eliminates important feature points which makes ve-
hicle detection unreliable for the automotive usage.
The baseline of the system B is variable and was cho-
sen as twice the size of system A. This enabled a reli-
able detection and depth reconstruction of up to 30m
and vehicles could even be identified at higher dis-
tances, but with inaccurate dimensions. The vehicle
recognition quality was steady over the speed range
30 km/h - 130 km/h. This approach produces suitable
output for a vision-based ACC application. Parts of
this article have also been published as part of (Neve,
2009).
REFERENCES
Bertozzi, M., Broggi, A., Fascioli, A., and Nichele, S.
(2000). Stereo vision-based vehicle detection.
Chang, P., Hirvoven, D., Camus, T., and Southall, B.
(2005). Stereo-Based Object Detection, Classification
and Quanititative Evaluation with Automotive Appli-
cations. In Proceedings of the 2005 IEEE Computer
Science Conference on Computer Vision and Pattern
Recognition (CVPR’05).
Dorin Comaniciu and Peter Meer (1998). Distribution Free
Decomposition of Multivariate Data. In SSPR/SPR,
pages 602–610.
Dorin Comaniciu and Peter Meer (2002). Mean Shift:
A Robust Approach Toward Feature Space Analysis.
IEEE Transactions on Pattern Analysis and Machine
Intelligence, 24:603–619.
Gwenaelle Toulminet, Massimo Bertozzi, Stephane Mous-
set, Abdelaziz Bensrhair, and Alberto Broggi (2006).
Vehicle detection by means of stereo vision-based ob-
stacles features extraction and monocular pattern anal-
ysis. In Image Processing, IEEE Transactions on, vol-
ume 15, pages 2364– 2375.
Hong Wang, Qiang Chen, W. C. (2006). Shape-based
Pedestrian/Bicyclist Detection via Onboard Stereo Vi-
sion. In Computational Engineering in Systems Ap-
plications, IMACS Multiconference on, pages 1776–
1780, Beijing, China.
Huang, Y., Fu, S., and Thompson, C. (2005).
Stereovision-Based Object Segmentation for Au-
tomotive Applications. EURASIP Journal on
Applied Signal Processing, 2005(14):2322–2329.
doi:10.1155/ASP.2005.2322.
Labayrade, R., Aubert, D., and Tarel, J.-P. (2002). Real
Time Obstacle Detection on Non Flat Road Geom-
etry through ‘V-Disparity’ Representation. In Pro-
ceedings of IEEE Intelligent Vehicle Symposium, Ver-
sailles, France.
Neve, A. (2009). 3D Object Detection for Driver Assistance
Systems in Vehicles. PhD thesis, Technische Universi-
taet Muenchen.
Pang, C., Lam, W., and Yung, N. (2004). A novel method
for resolving vehicle occlusion in a monocular traffic-
image sequence. In Intelligent Transportation Sys-
tems, IEEE Transactions, volume 5, pages 129 – 141.
Pasquale Foggia, Alessandro Limongiello, and Mario
Vento (2005). A Real-Time Stereo-Vision System For
Moving Object and Obstacle Detection in AVG and
AMR Applications. In CAMP, pages 58–63.
Richard Hartley and Andrew Zisserman (2003). Multiple
View Geometry in Computer Vision. Cambridge Uni-
versity Press.
Sergiu Nedevschi, Radu Danescu, Dan Frentiu, Tiberiu
Marita, Florin Oniga, Ciprian Pocol, Rolf Schmidt,
and Thorsten Graf (2004). High accuracy stereo vi-
sion system for far distance obstacle detection. In In-
telligent Vehicles Symposium, 2004 IEEE, pages 292
– 297.
Stephan Lanser, Christoph Zierl, and Roland Beutlhauser
(1995). Multibildkalibrierung einer CCD-Kamera.
Technical report, Technische Universitt Mnchen.
Sun, Z., Bebis, G., and Miller, R. (2006). on-road vehicle
detection using optical sensors: A review. In IEEE
Transactions on pattern analysis and machine intelli-
gence, volume 28.
Thomas A. Runkler (2000). Information Mining. Vieweg.
Vogel, K. (2007). High-accuracy reference data acquisition
for evaluation of active safety systems by means of a
rtk-gnss-surveying system. In Proceedings of the 6th
European Congress and Exhibition on ITS, Aalborg.
Denmark.
Vogel K., Schwarz D., W. C. (2008). Reference maps of
adas scenarios by application of a rtk-gnss system.
In Proceedings 7th European Congress and Exhibi-
tion on Intelligent Transport Systems and Services,
Geneva. Switzerland.
VISAPP 2010 - International Conference on Computer Vision Theory and Applications
438