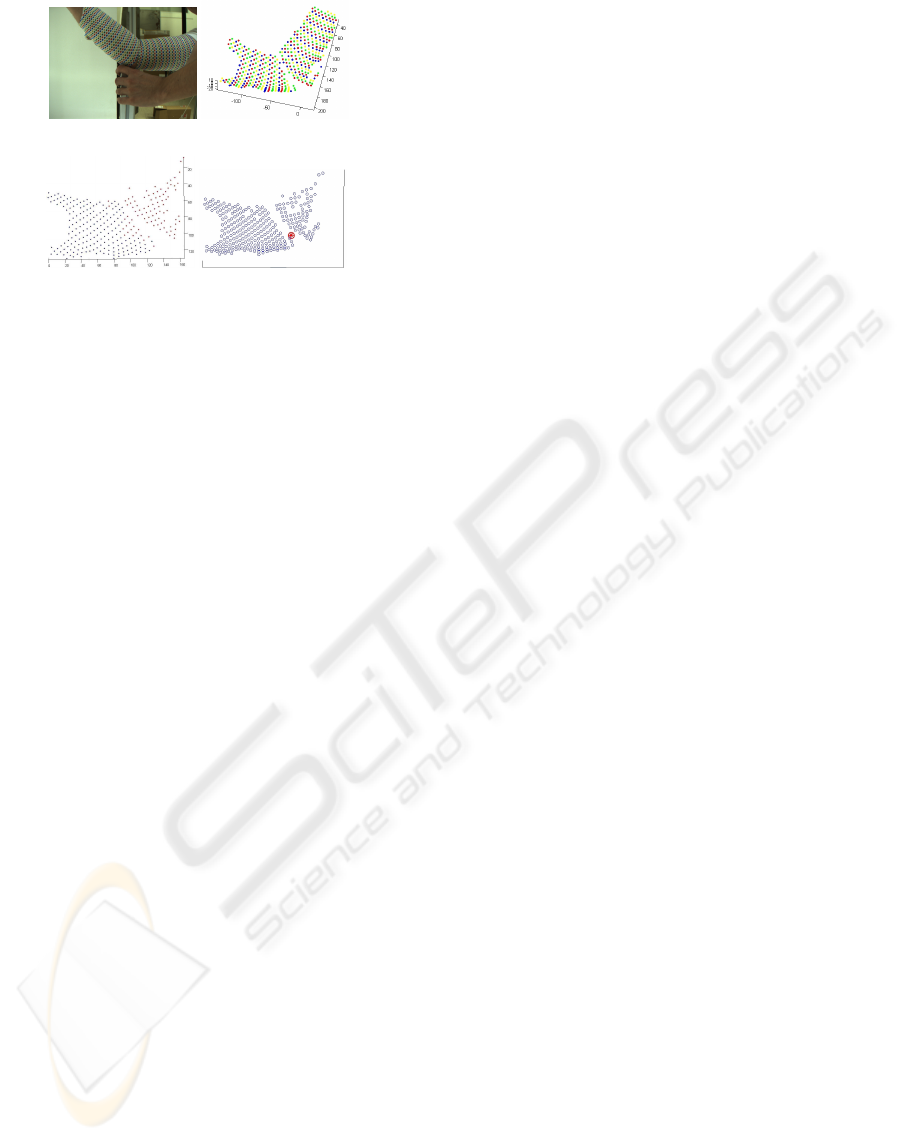
(a) Original image. (b) Sample frame.
(c) LSA segmentation. (d) Estimated Joint.
Figure 11: The second experiment setup. The images show:
a) a sample frame, b) a reconstructed frames, c) the segmen-
tation result and d) the estimated rotational joint (red).
7 CONCLUSIONS
In this work we address the problem of the 3D mo-
tion segmentation of a non-rigid pair of bodies (a hu-
man arm and forearm) connected by a rotational joint.
In such regard we develop all the stages of the mo-
tion segmentation procedure from the acquisition to
the joint parameters estimation. The main novelty of
the presented approach resides in the 3D point match-
ing stage which has to cope with soft tissue artifacts
in the data. The multi camera facility is able to esti-
mate the position in 3D space of each marker with an
accuracy of about 0.5mm and a maximum resolution
of 5 markers per square centimeter. The 95% ellipse
of uncertainty associated with each marker location
is estimated taking into account both the setup intrin-
sic/extrinsic parameters and the accuracy of the mark-
ers acquired images. We also carried out an evalua-
tion of standard motion segmentation algorithm in the
case of articulated bodies which present soft-tissue
artifacts. The LSA approach is the best performing
method for the test case showed in this work but more
experimental evidence is required to asses the algo-
rithms with different body parts.
To evaluate the localization of the joint we used
both the human arm sequence and the sequence of
two bodies constrained by a rotational joint. In the
latter case the outcome was an accurate estimation of
the joint location. In the first case we performed two
relative motions between arm and forearm. When the
wrist rotates together with the elbow the joint estima-
tion as a single degree of freedom constraint failed to
estimate the correct location of the elbow. This can
be easily explained due to the complex relative mo-
tion involving at least two degrees of freedom. When
the wrist is held at a constant attitude with respect to
the elbow the joint was correctly estimated.
REFERENCES
Boujou (2009). Boujou. http://www.vicon.com/boujou/.
Corazza, S., M
¨
undermann, L., and Andriacchi, T. (2007).
A framework for the functional identification of
joint centers using markerless motion capture, vali-
dation for the hip joint. Journal of Biomechanics,
40(15):3510–3515.
De Cecco, M., Pertile, M., Baglivo, L., Lunardelli, M.,
Setti, F., and Tavernini, M. (2009). A unified frame-
work for uncertainty, compatibility analysis and data
fusion for multi-stereo 3d shape estimation. IEEE
Transactions on Instrumentation Measurements. Ac-
cepted for publication.
Fayad, J., Del Bue, A., Agapito, L., and Aguiar, P. (2009).
Human body modelling using quadratic deformations.
In 7th EUROMECH Solid Mechanics Conference, Lis-
bon, Portugal.
Fayad, J. K. and P. M. Q. Aguiar, A. D. B. (2009). A
weighted factorization approach for articulated mo-
tion modelling. In Multibody Dynamics 2009, War-
saw, Poland, volume 2, pages 1110–1115.
Fischler, M. A. and Bolles, R. C. (1987). Random sample
consensus: A paradigm for model fitting with applica-
tions to image analysis and automated cartography. In
Fischler, M. A. and Firschein, O., editors, Readings in
Computer Vision: Issues, Problems, Principles, and
Paradigms, pages 726–740. Los Altos, CA.
Ioannidis, D., Tzovaras, D., Damousis, I. G., Argyropoulos,
S., and Moustakas, K. (2007). Gait recognition using
compact feature extraction transforms and depth infor-
mation. IEEE Transactions on Information Forensics
and Security, 2:623–630.
Kanatani, K. (1996). Statistical optimization for geometric
computation: theory and practice. Elsevier Science
Inc. New York, NY, USA.
Scholz, V. and Magnor, M. (2006). Multi-view video
capture of garment motion. Proceedings of IEEE
Workshop on Content Generation and Coding for 3D-
Television, pages 1–4.
Tresadern, P. and Reid, I. (2005). Articulated structure from
motion by factorization. In Proc. IEEE Conference on
Computer Vision and Pattern Recognition, San Diego,
California, volume 2, pages 1110–1115.
Tron, R. and Vidal, R. (2007). A benchmark for the compar-
ison of 3-d motion segmentation algorithms. In IEEE
conference on computer vision and pattern recogni-
tion, volume 4.
Vidal, R., Ma, Y., and Sastry, S. (2005). Generalized
principal component analysis (gpca). IEEE Transac-
tions on Pattern Analysis and Machine Intelligence,
27(12):1945–1959.
White, R., Crane, K., and Forsyth, D. (2007). Capturing
and animating occluded cloth. ACM Transaction on
Graphics.
Yan, J. and Pollefeys, M. (2008). A factorization-based
approach for articulated non-rigid shape, motion and
kinematic chain recovery from video. IEEE Transac-
tions on Pattern Analysis and Machine Intelligence,
30(5).
VISAPP 2010 - International Conference on Computer Vision Theory and Applications
372