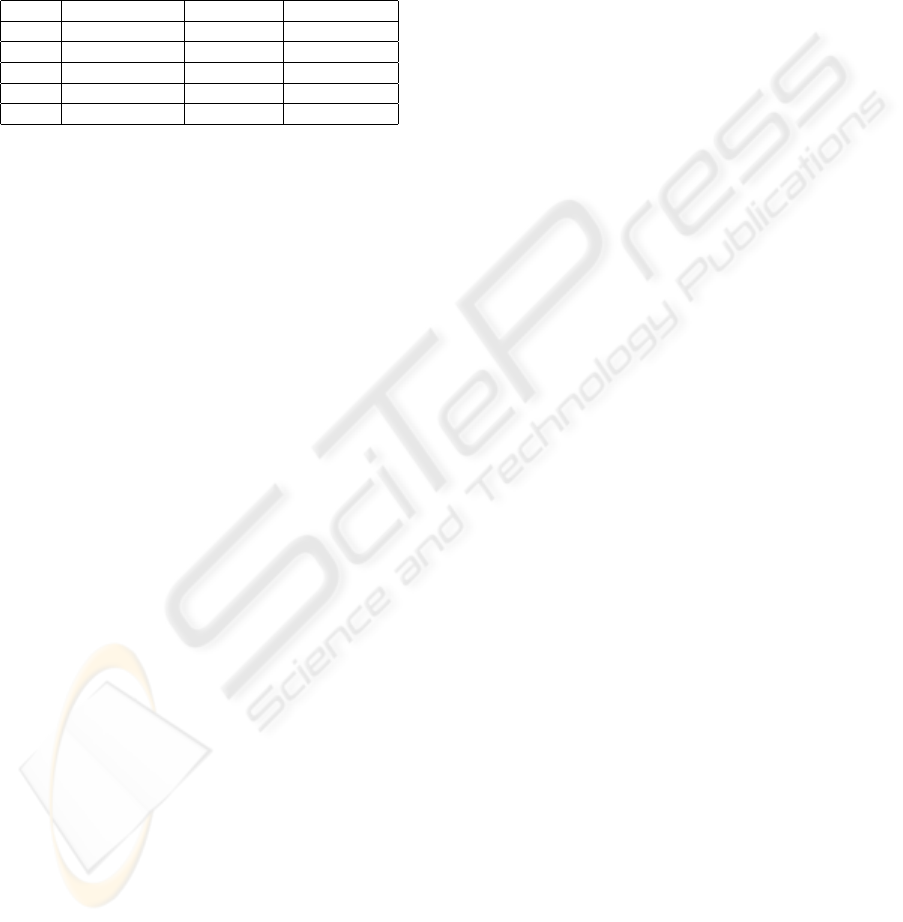
score of each group the choice of positive samples for
each sub-sequent group is quite sensitive task. The
results are quite promising and provide sufficient mo-
tivation to explore and optimize the sub-sequent tree
nodes by improving the decision function. The recog-
nition rate is provided in the Table 1.
Table 1: VOC2006 results - The group scores are obtained
on VAL dataset while the rest are on test dataset.
Groups Wheeled Vehicle Carnivore Hoofed Mammal
83.28 70.24 69.65
Classes Bicycle—53.17 Cow—15.97 Cat—34.61
Bus— 43.07 Horse—11.95 Dog—30.77
Car— 61.06 Sheep—26.36
Motorbike—25.16
5 CONCLUSIONS
We have analyzed and found that the proposed algo-
rithm provide a comparable accuracy for classifica-
tion when used to classify a particular group. The ini-
tial idea worked quite well but thereafter need to be
refined to identify the cause of failure when moving
to the sub-group and then to the base classifier. Es-
pecially for those classes where we already have very
few training samples in the whole dataset; if not iden-
tified correctly at root-node fails completely when
reaching down to the base classifier. The available ob-
ject classification datasets for Pascal VOC challenges
contain very few positive examples for some classes
and are not balanced. Although training SVM or
boosting algorithm with very few training examples is
an active area of research for machine learning com-
munity (Hu et al., 2007), (Mutch and Lowe, 2008)
and (Janez Brank and Mladenic, 2003). A compara-
tive study could be carried out using existing learning
techniques like boosting to training the hierarchical
classification tree and to compare them with SVM ap-
proach.
ACKNOWLEDGEMENTS
This work was supported in part by the Austrian Sci-
ence Fund FWF (S9104-N13 SP4). The research
leading to these results has also received funding from
the European Communitys Seventh Framework Pro-
gramme (FP7/2007- 2013) under grant agreements n
216886 (PASCAL2 Network of Excellence).
REFERENCES
Cheng, L. Y. J. Y. N. Z. H. (2009). Layered object catego-
rization. In ICPR 2008. 19th International Conference
on Pattern Recognition, pages 1–4.
Deng, J., Dong, W., Socher, R., Li, L.-J., Li, K., and Fei-
Fei, L. (2009). ImageNet: A Large-Scale Hierarchical
Image Database. In CVPR09.
G., M., D., G., and I., C. (2009). A multi-class svm classifier
utilizing binary decision tree. Informatica, 33.
Hu, Q., Yu, D., and Xie, Z. (2007). Selecting samples and
features for svm based on neighborhood model. In
RSFDGrC ’07: Proceedings of the 11th International
Conference on Rough Sets, Fuzzy Sets, Data Min-
ing and Granular Computing, pages 508–517, Berlin,
Heidelberg. Springer-Verlag.
Janez Brank, Marko Grobelnik, N. M.-F. and Mladenic, D.
(2003). Training text classifiers with svm on very few
positive examples. Technical Report.
Lowe, D. G. (2004). Distinctive image features from scale-
invariant keypoints. International Journal of Com-
puter Vision, 60:91–110.
Maillot, N., Thonnat, M., and Hudelot, C. (2004). Ontology
based object learning and recognition: application to
image retrieval. In Tools with Artificial Intelligence,
2004. ICTAI 2004. 16th IEEE International Confer-
ence on, pages 620–625.
Marszałek, M. and Schmid, C. (2007). Semantic hierar-
chies for visual object recognition. In Conference on
Computer Vision & Pattern Recognition.
Mutch, J. and Lowe, D. (2008). Object class recognition
and localization using sparse features with limited re-
ceptive fields. International Journal of Computer Vi-
sion, 80(1):45–57.
VISAPP 2010 - International Conference on Computer Vision Theory and Applications
536