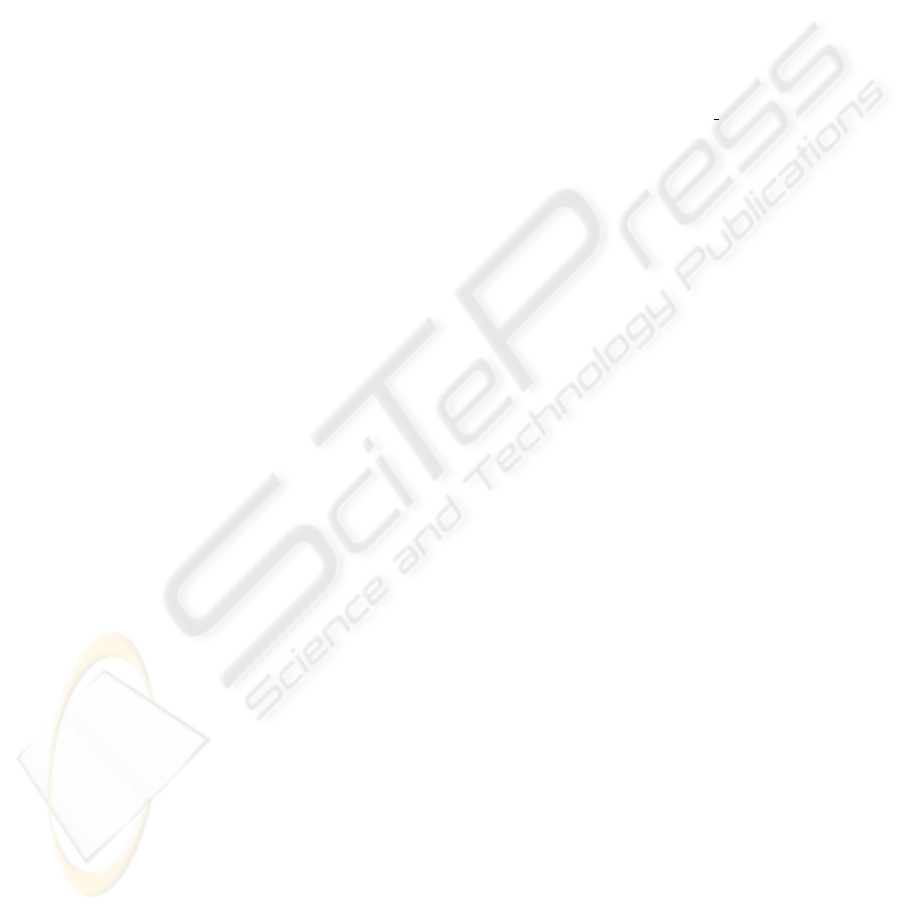
pairs whose UCPs had a similarity of 55% and above,
92% showed content similarities during our manual
comparison. This content similarity was only weakly
indicated when comparing the objects metadata (e.g.
title, description and tags) automatically. Focusing on
objects’ usage contexts therefore seems a promising
base for recommendations and will be further investi-
gated by us.
We are also currently working on utilizing the
rough set theory to classify user activities. The rough
set theory (Pawlak, 1982; Pawlak, 1991), as an ex-
tension of the classical set theory, is a mathematical
framework to analyse data under uncertainty. It pro-
vides some methods for data reduction and the ap-
proximation of concepts, e.g. the indiscernibility re-
lation, the reduct generation and the lower and upper
approximation respectively. These tools could be suit-
able to support self-monitoring and self-reflection as
suggested in the following way: the indiscernibility
relation is a parameterising equivalence relation with
respect to the used attribute set. Thus, it can be ap-
plied to extract and represent behaviour patterns of a
group of learners or an individual one. Furthermore,
it can be used as an initial partition to develop an
agglomerative hierarchical clustering algorithm with
a reasonable distance measure or metric respectively
for CAM. Some expedient measures have been re-
viewed in (Grimmer and Mucha, 1998; Maimon and
Rokach, 2005), but we are not limited to the listed
ones. So, on the one hand our framework can be
enriched with an unsupervised learning process for
CAM observations. On the other hand the learned
classification of user activities can be evaluated with
the given concept approximation of the rough set the-
ory which could push the qualitiy of CAM analysis
for our research. Our first results on the rough set the-
ory in combination with object-relational databases
have been documented in (Beer, 2009). They show
that the automatically classified activities indeed cor-
respond to users’ manually recorded activities during
the day.
6 CONCLUSIONS
We have presented a framework that records usage
beahviour and stores a learner’s computer-based ac-
tivities as Contextualized Attention Metadata which
can then be exploited by analysis applications. First
results have shown that the collected CAM can be pre-
sented to a learner in such a way that supports self-
regulated learning. The recorded attention metadata
can also be used as a basis for recommendations or
learning activity classification. We now want to ex-
tend our findings and explore further possibilities of
CAM analysis. Weighing of context when basing rec-
ommendations on the usage context is one aspect, for
example. We also want to detect actions, e.g. whether
a learner is reading a document, and combine these
findings with pedagogical models. Evaluation of all
our current results with test beds is another very im-
portant aspect we are looking into at the moment.
REFERENCES
Ariadne (2006). Alocom tools. Retrieved Jan-
uary 19, 2010, from http://www.ariadne-
eu.org/index.php?option=com content&task=view&id
=65&Itemid=9.
Beer, F. (2009). Objektrelationale Datenbanken und Rough
Sets f
¨
ur die Analyse von Contextualized Attention
Metadata. Master’s thesis, University of Applied
Sciences Bonn-Rhein-Sieg (Department of Computer
Science).
Boekaerts, M., Pintrich, P., and Zeidner, M., editors (2000).
Handbook of self-regulation. Academic Press, San
Diego.
Butler, D. and Winne, P. (1995). Feedback and self-
regulated learning: A theoretical synthesis. Review
of Educational Research, 65(3):245–281.
Fowler, M. (2002). Patterns of Enterprise Application Ar-
chitecture. Addison-Wesley.
Fowler, M. (2004). Inversion of control con-
tainers and the dependency injection pat-
tern. Retrieved January 19, 2010, from
http://martinfowler.com/articles/injection.html.
Friedrich, M., Niemann, K., Scheffel, M., Schmitz, H.-C.,
and Wolpers, M. (2009). Object Recommendation
based on Usage Context. Workshop about Context-
aware Recommendation for Learning at the STEL-
LAR Alpine Rendez-Vous 2009.
Gamma, E., Helm, R., and Johnson, R. E. (1995). Design
Patterns - Elements of Reusable Object-Oriented Soft-
ware. Addison-Wesley.
Gress, C., Fior, M., Hadwin, A., and Winne, P. (i.p.). Mea-
surement and assessment in computer-supported col-
laborative learning. Computers in Human Behavior,
in press(corrected proof).
Grimmer, U. and Mucha, H.-J. (1998). Datensegmentierung
mittels Clusteranalyse. In Nakhaeizadeh, G., editor,
Data Mining - Theoretische Aspekte und Anwendun-
gen, pages 109–141. Physica Verlag.
Hack, S. and Lindemann, M. (2007). Enterprise SOA
einf
¨
uhren. Galileo Press.
Kitsantas, A. (1997). Self-monitoring and attribution influ-
ences on self-regulated learning of motoric skills. Pa-
per presented at the annual meeting of the American
Educational Research Association.
ANALYSING CONTEXTUALIZED ATTENTION METADATA FOR SELF-REGULATED LEARNING - A Supporting
Framework for Self-monitoring and Self-reflection
345