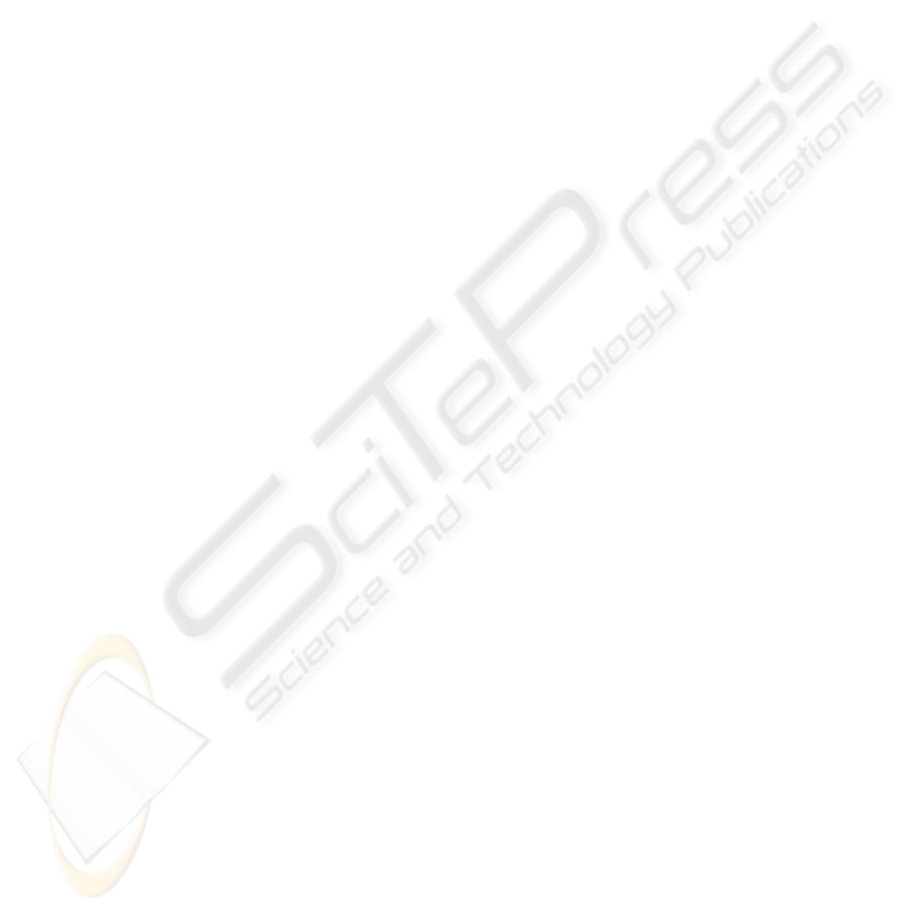
accountants’ point of view, related to the financial
perspective, while the second one is coming from
the managerial point of view.
Based on the idea that each firm is different from
another, we proposed a single firm model that will
help the managers to decide which component of
collaborative learning they should improve on their
enterprises based on their purpose. For this reason
the qualitative variables taken into account will be
ordered using grey systems theory and ϕ-fuzzy sub-
set theory as we shall see in the following.
2 COLLABORATIVE LEARNING
THROUGH E-LEARNING IN
ENTERPRISES
Over the past years, work becomes more
interdisciplinary, complicated and nevertheless
knowledge-based. In this sophisticated environment,
in which enterprises carry out their activity, e-
learning succeeded through the different interaction
tools to offer ample opportunities for learners to
collaborate with peers, experts, professionals or with
other learners.
The evolution of knowledge-based society
involves the development of enterprises through a
collaborative learning environment. Collaboration is
an important dimension when it comes to sharing
and integrating the experiences and training courses
of different groups of learners. Supervisors,
instructors, and learners from enterprises play
different roles in the learning process. They need to
work in the same environment, collaboratively
instead of individually, to perform an adaptive
learning strategy. (Yi, Schwaninger, & Gall, 2008)
In an enterprise, the use of collaborative learning
can develop higher level thinking skills, social
interaction skills, responsibility for each other and
even promote higher achievement. (Chang & Lee,
2009)
Interaction between learners is indispensable to
collaborative learning, and learners need to do real
work together in which they promote each other's
success by sharing resources, discussing, helping,
and congratulating each other's efforts to achieve.
(Wu, Zhengbing, Yang, & Liu, 2009)
The collaborative learning process from an
enterprise may be achieved through a virtual campus
or an intranet e-learning platform. The intranet
collaborative learning system should focus on how
to instruct and stimulate learners to achieve
knowledge, and the system is to visualize traditional
classroom education and learning environment in
web. (Xiuhua & Wenfa, 2008)
The intranet collaborative learning system is a
virtual organizational structure of collaborative type
in which interact three target groups:
The target group of learners, composed by
participants in tele-activities of training, testing,
documentation, participation in online meetings,
forums communication;
The target group of teachers who complete
multimedia teaching materials for virtual
campus training, evaluates papers submitted
online, update databases proper evaluations;
The target group of people outside the system,
which informs about the performance on
collaborative learning system and which allows
the selection on learners by interact and
conveying the information. (Ciurea, 2009)
Related to the idea of collaboration through
virtual knowledge spaces some systems have been
developed that insure more flexible mechanisms to
foster communication and cooperation in learning
and work processes. (Eßmann, Gotz, & Hampel,
2006)
Based on recent researches, some quantitative
and qualitative characteristics regarding the
collaborative learning through e-learning can be
identified, with a peculiar impact on enterprise’s
performance.
Some of qualitative characteristics related to the
knowledge acquisition (at individual, group and
enterprise level) and learning flow (exploration and
exploitation) are listed in the following: (Kreijns,
Kirschner, & Jochems, 2003) (Baker & Sinkula,
1999), (Prieto & Revilla, 2006) (Bodea & Dascalu,
2009)
The degree of group cohesion gained through
collaborative learning;
The development of critical thinking, shared
understanding, and long term retention of the
learned material;
The capability of the collaborative learners to
resolve effectively the conflicts;
The capacity of sharing successes and failures
within the collaborative group;
The degree of confidence and responsibility felt
by individuals about doing their work;
The degree to which the quality of the
enterprise’s market-oriented behaviours are
improved by collaborative learning through e-
learning;
ICEIS 2010 - 12th International Conference on Enterprise Information Systems
6