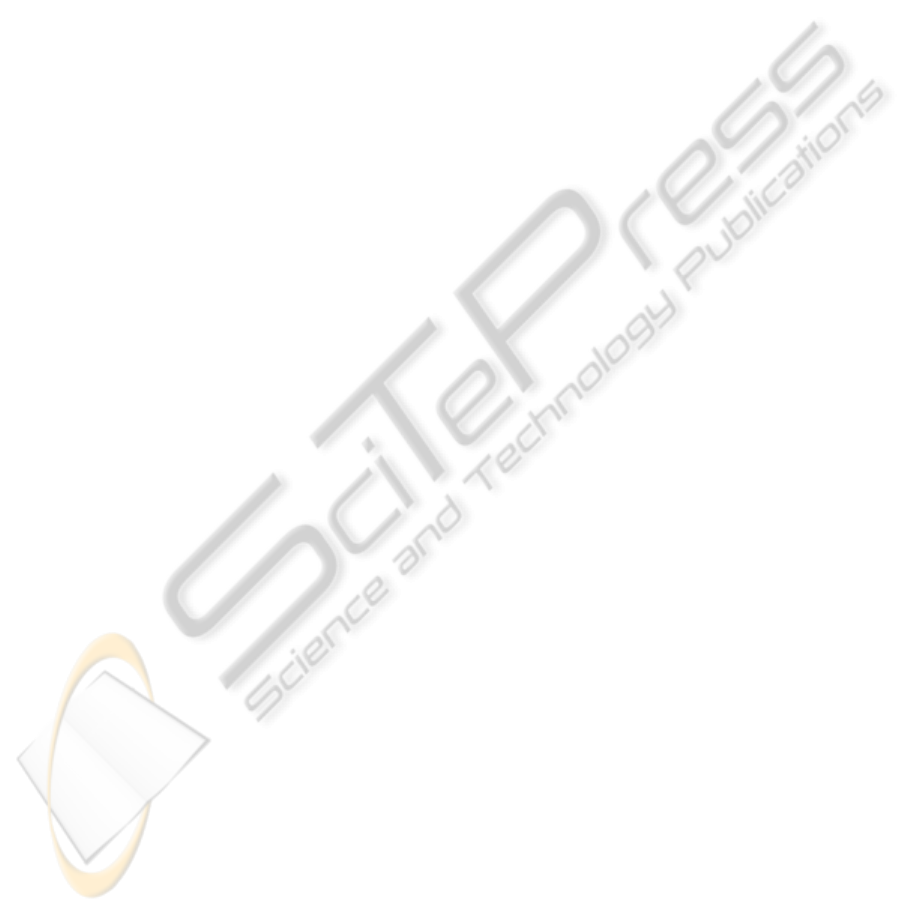
simplification is that depending on the kind of fruits
to be transported, it is required to tune the algorithm
by a correct selection of γ which has to be known in
advance. An improvement can be observed in the
accuracy of the predictions if γ is set according to
the position of the pallets inside the container.
From the simulation results it is concluded that
the FH identification algorithm is efficient when the
cargo emits organic heat. The method of FH of order
1 is optimal to achieve all figures of merit. It makes
accurate predictions only after three days of training
and maintains low dimensions of matrices.
However, if the linear method is applied to the
banana datasets, a comparable accuracy can only be
achieved after more than five days of training. Also,
it is concluded that when the goods to transport are
free of organic heat, such as in the case of cheese, it
is preferable to use a linear system instead.
ACKNOWLEDGEMENTS
The authors would like to express their gratitude to
Prof. Rainer Laur, Dirk Hentschel, Mehrdad
Babazadeh, and Chanaka Lloyd for all their help.
This research was supported by the German
Research Foundation (DFG) as part of the
Collaborative Research Centre 637 “Autonomous
Cooperating Logistic Processes”. Further project
information can be found at
www.intelligentcontainer.com.
REFERENCES
Babazadeh, M., Kreowski, H.-J., Lang, W, 2008. Selective
Predictors of Environmental Parameters in Wireless
Sensor Networks. In International Journal of
Mathematical Models and Methods in Applied
Sciences Vol.2, pages 355-363.
Guo, F., 2004. A New Identification Method for Wiener
and Hammerstein System. Ph.D. Thesis, Institut für
Angewandte Informatik Forschungzentrum Karlsruhe.
Karlsruhe.
Jedermann, R., Becker, M., Görg, C., Lang, W., 2010.
Field Test of the Intelligent Container, In European
Conference on Wireless Sensor Networks
EWSN2010, 16-19 February 2010 in Coimbra,
Portugal.
Landau, I.D., Zito G., 2006. Digital Control Systems:
Design, identification and implementation. Springer.
London, 1st edition.
Mercantila. 1989. Guide to food transport: fruit and
vegetables. Mercantila Publ. Copenhagen.Moureh J.,
Flick, D., 2004. Airflow pattern and temperature
distribution in a typical refrigerated truck
configuration loaded with pallet. In International
Journal of Refrigeration, V. 27, Issue 5, pages 464-
474.
Palafox J., 2009. Prediction of temperature in the transport
of perishable goods based in On-line System
Identification. M.Sc. Thesis, University of Bremen.
Bremen.
Rouaud, O., Havet, M., 2002. Computation of the airflow
in a pilot scale clean room using K-ε turbulence
models, In International Journal of Refrigeration, Vol.
25, Issue 3, pages 351-361.
Shaikh, N. I., Prabhu, V., 2007.Mathematical modeling
and simulation of cryogenic tunnel freezers. In Journal
of Food Engineering, Vol. 80, Issue2, pages 701-710.
Smale, N. J., Moureh,J., Cortella, G., 2007.A review of
numerical models of airflow in refrigerated food
applications. In International Journal of Refrigeration,
Vol. 29, Issue 6, pages 911-930.
LIST OF ABBREVIATIONS
ARMAX
ARX
CFF
DG
FF
FH
MA
MSE
PAA
WN
WSN
Auto Regressive Moving Average
with External input.
Auto Regresive with External input.
Constant Forgetting Factor
Decreasing Gain
Forgetting Factor
Feedback Hammerstein
Moving Average
Mean Squared Error
Parameter Adaptation
AlgorithmWhite Noise
Wireless Sensor Node
PREDICTION OF TEMPERATURE INSIDE A REFRIGERATED CONTAINER IN THE PRESENCE OF
PERISHABLE GOODS
27