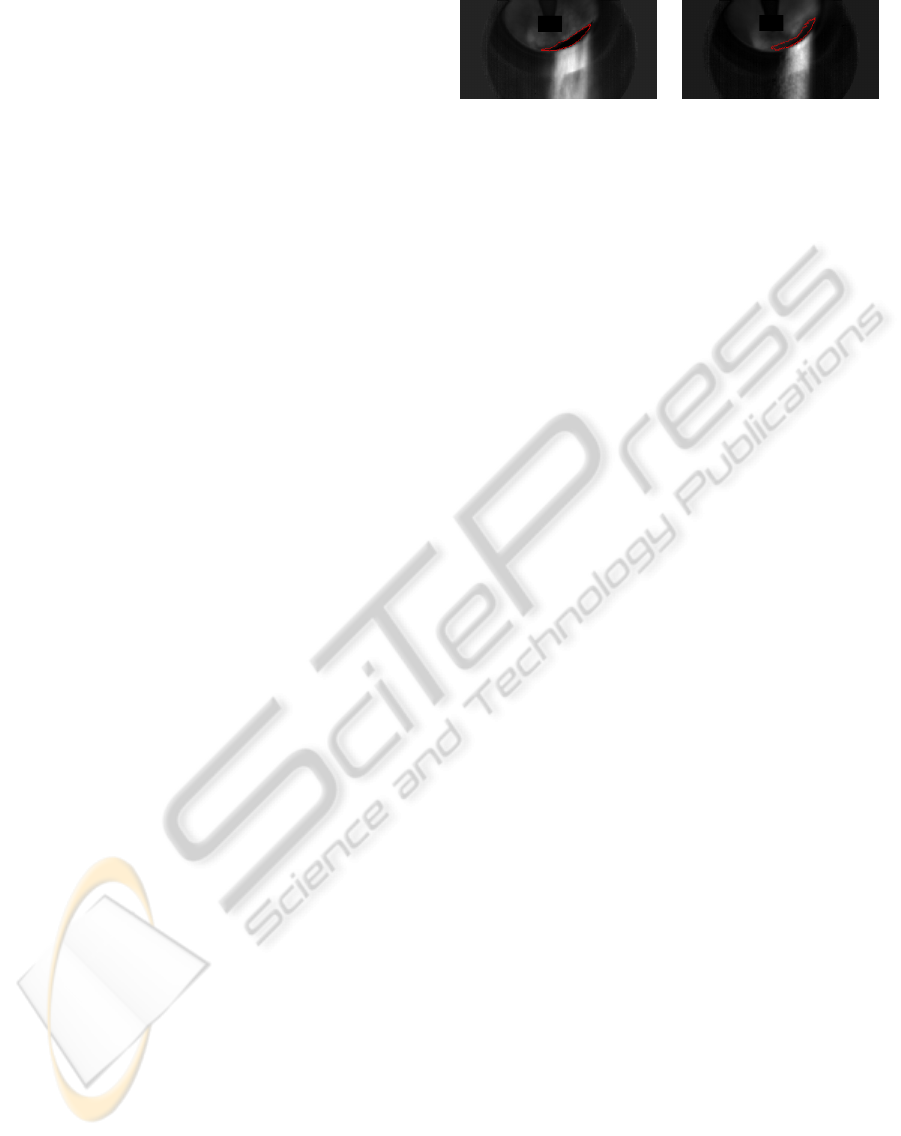
mentation result the intensity-based part is addressed
again. The merging step is the key element of the al-
gorithm. The preliminary segmentation result of the
intensity-based part is combined with the dynamic-
based computations. Cold lumps within the solid bed
are always either segmented solely or together with
the rest of the bed by the automatic threshold step due
to their low temperatures. The automatic threshold-
ing is done with both images which are used for the
respective differencing step. The pixels which are de-
fined as solid bed in at least one of the images are
approved as solid bed region in the disjunction step,
i.e. a relocation of a lump can be captured. Now,
all pixels included in the identified intensity-based
solid bed region are set to 0 in the current difference
image. This merging situationally leads to an en-
hanced distinguishability between the gas phase and
solid bed in the averaged difference images. If the
mean temperature of the kiln is high, the segmentation
algorithm is predominantly based on the dynamics-
based part since the intensity-based part rarely de-
tects the solid bed region. When there is a high con-
trast between solid bed and gas phase in the input im-
ages the intensity-based part boosts the results of the
dynamics-based part due to the merging step. Ad-
ditionally, high difference values at the contours of
moving lumps are prevented through this combina-
tion. For the final segmentation step a region growing
algorithm is implemented.
3.2 Results and Discussion
In figure 2 segmentation results at the frontal view
position with an fixed threshold segmentation are il-
lustrated. The intensity-based threshold segmentation
succeeds to detect the solid bed region in image se-
quences with low mean temperatures of the kiln. As
soon as the temperature rises this method fails. Even
an adaptive thresholding is not applicable at higher
mean temperatures, as there are no intensity differ-
ences between gas phase and solid bed. Figure 4
shows segmentation results with the combined seg-
mentation algorithm. They are each based on 200
frames. It can be seen that the modified averaged dif-
ference images possess high contrasts between solid
bed and gas phase regions also at high mean temper-
atures of the kiln. This enables an accurate final seg-
mentation with the region growing algorithm.
The developedcombinedsegmentation filter chain
proved to be a reliable and precise method to detect
the solid bed in scenario 1 infrared images. This al-
lows the extraction of process relevant solid bed fea-
tures in future works. It is e.g. possible to define a
circle segment with two parameters corresponding to
Figure 4: Segmentation with modified averaged difference
images at low (left) and high (right) mean temperatures.
filling height and repose angle. In an iterative opti-
mization process both parameters are adapted to find
the best-fit of circle segment and segmented solid bed.
Besides acquiring these two parameters, the remain-
ing error between circle segment and solid bed region
can be used to discriminate the bed movement (e.g.
slumping or rolling).
4 Image Segmentation - Scenario 2
Conversely to scenario 1 in this case the solid bed has
to be distinguished from the inner kiln wall instead of
the gas phase. Intensity values cannot be used for the
segmentation since the temperatures of kiln wall and
solid bed are similar. Especially at the upper border
of the solid bed, where solid parts stick to the wall,
an intensity-based segmentation has little prospect of
success. The filter chain we developed makes use of
the different dynamic properties of the kiln wall and
the solid bed. In particular we discriminate the steady
rotating movement of the wall from the specific mix-
ing movement of the solid bed.
4.1 Method
The first step of our segmentation process is a map-
ping. Two circles in the acquired images are set, in
order to define the geometry of the inner surface of
the kiln. Then a geometric mapping of the inner sur-
face to a rectangle is performed (Figure 5 left). With
the mapped intensity values a movement analysis is
conducted. An optical-flow algorithm (Brox et al.,
2004) computes a vector field out of two successive
mapped images (Figure 5 right). Each vector spec-
ifies the direction and the magnitude of the move-
ment of a pixel between two frames. Due to the map-
ping step the rotation of the kiln is transformed in a
rightward movement. Consequently, discriminating
the solid bed from the kiln wall corresponds to de-
tecting the image region that is not constantly mov-
ing to the right. Since the material of the solid bed is
also transported up along the kiln wall before it col-
lapses respectively slides back in the opposite direc-
tion, an discrimination via movement is not possible
at all times. Thus we implemented a moving aver-
age filter which averages the movements of the last
SEGMENTATION OF THE SOLID BED IN INFRARED IMAGE SEQUENCES OF ROTARY KILNS
219