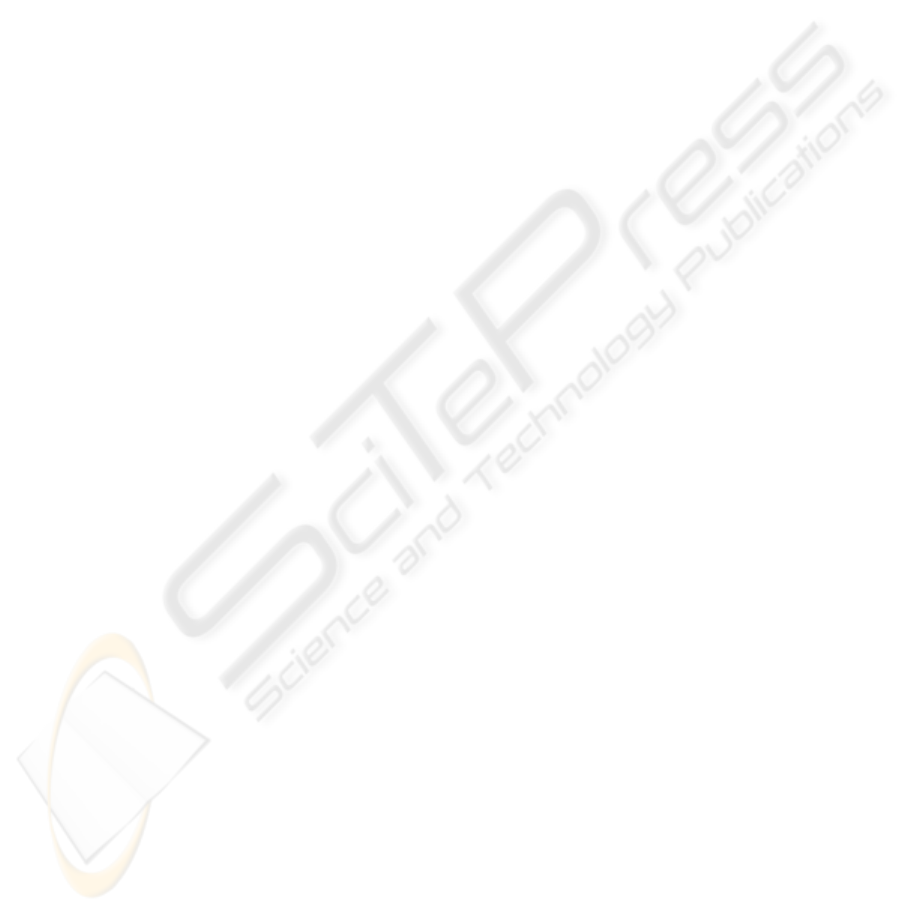
Active involvement in learning – "students who
learn are students who stay". Social learning,
where students learn in groups, is particularly
valuable, and can help foster friendship, which is
another factor that encourages student
persistence.
We are currently developing the intervention part
of MCMS, which includes a website to monitor
student, module, and course information. When a
student logs in, he/she can check the detail
information of all module results, his/her progress
chart, whether he/she is at risk and why. As for
tutors, they can login to find detail related module
information, which students are at risk and why; if
they want to contact the student, they could edit an
email which is automatically generated by the
system. They can also compare the information of
related modules in different semesters. Similarly,
program leaders and the head of school can also
login to find the information related to their
programme.
Different reports with different formats and
templates will be sent out automatically by the
system as well. For example, students are divided
into different group and different intervention rules
will be applied to different groups. Students will
receive a scheduled email once per month with their
detail of their progress of that month in a PDF
report. At different times of the semester, emails will
also be sent out automatically to provide students
with help, such as at the beginning of semester,
before exams etc. Triggered emails will be sent out
once students’ risky values are beyond the threshold,
and explain how he/she could make improvement,
and who should be contacted. Tutors not only get
PDF reports of all their students at scheduled times,
but they also receive triggered email once students
or modules that may need their attention have been
identified.
We are also working to improve the system. For
example, we are investigating whether there is a
pattern in each individual’s reasoning in the decision
to withdraw, and how we should implement the
intervention programme to increase student retention
REFERENCES
Committee of Public Accounts, 2001-02. Fifty-eighth
Report of Session Improving Student Achievement and
Widening Participation in Higher Education in
England, HC 588.
Cristianini, N., Shawe-Taylor, J., 2000. An Introduction to
Support Vector Machines and other kernel-based
learning methods. Cambridge University Press.
Gabrilson, S., Fabro, D. D. M., Valduriez, P., 2008.
Towards the efficient development of model
transformations using model weaving and matching
transformations, Software and Systems Modeling
2003. Data Mining with CRCT Scores. Office of
information technology, Geogia Department of
Education.
Han, J. W., Kamber, M., 2006. Data Mining: Concepts
and Techniques, 2
nd
Edition, The Morgan Kaufmann
Series in Data Management Systems, Gray, J. Series
Editor, Morgan Kaufmann Publishers.
Harry, Z., 2004. The Optimality of Naive Bayes,
FLAIRS2004 conference.
Herzog, S., 2006. Estimating student retention and degree-
completion time: Decision trees and neural networks
vis-a-vis regression, New Directions for Institutional
Research, p.17-33.
Kim, H., Zhang, Y., Oussena, S., and Clark, T., 2009. A
Case Study on Model Driven Data Integration for Data
Centric Software Development, In Proceedings of
ACM First International Workshop on Data-intensive
Software Management and Mining.
Luan, J., 2002. Data mining and knowledge management
in higher education – potential applications. In
Proceedings of AIR Forum, Toronto, Canada.
Mazon, J. N., Trujillo, J., Serrano, M., Piattini, M., 2005.
Applying MDA to the development of data
warehouses. DOLAP 2005
Minaei-Bidgoli, B., Kortemeyer, G., Punch,W.F., 2004.
Enhancing Online Learning Performance: An
Application of Data Mining Methods, In Proceeding
of Computers and Advanced Technology in Education.
Oizilbash. H., 2008. TVU System Overview, Thames
Valley University.
Oussena, S., 2008. Mining Courses Management Systems,
Thames Valley University.
Quinlan, J. R., 1986. Induction of Decision Trees.
Machine Learning 1, 1 pp.81-106.
Schönbrunn, K., Hilbert, A., 2006. Data Mining in Higher
Education, Studies in Classification, Data Analysis,
and Knowledge Organization Advances in Data
Proceedings of the 30th Annual Conference of the
Gesellschaft für Klassifikation e.V., Berlin.
Seidman, A., 1996. Spring Retention Revisited: RET = E
Id + (E + I + C)Iv. College and University, 71(4), 18-
20.
National Audition Office, 2007, Staying the course: the
retention of students in higher education.
Spasic, I., Ananiadou, S., McNaught, J., Kumar, A., 2005.
Text mining and ontologies in biomedicine: Making
sense of raw text. Briefings in Bioinformatics 6(3):
239-251.
Superby, J. F., Vandamme, J. P., Meskens, N., 2006.
Determination of factors influencing the achievement
of the first-year university students using data mining
methods. Workshop on Educational Data Mining.
ICEIS 2010 - 12th International Conference on Enterprise Information Systems
196