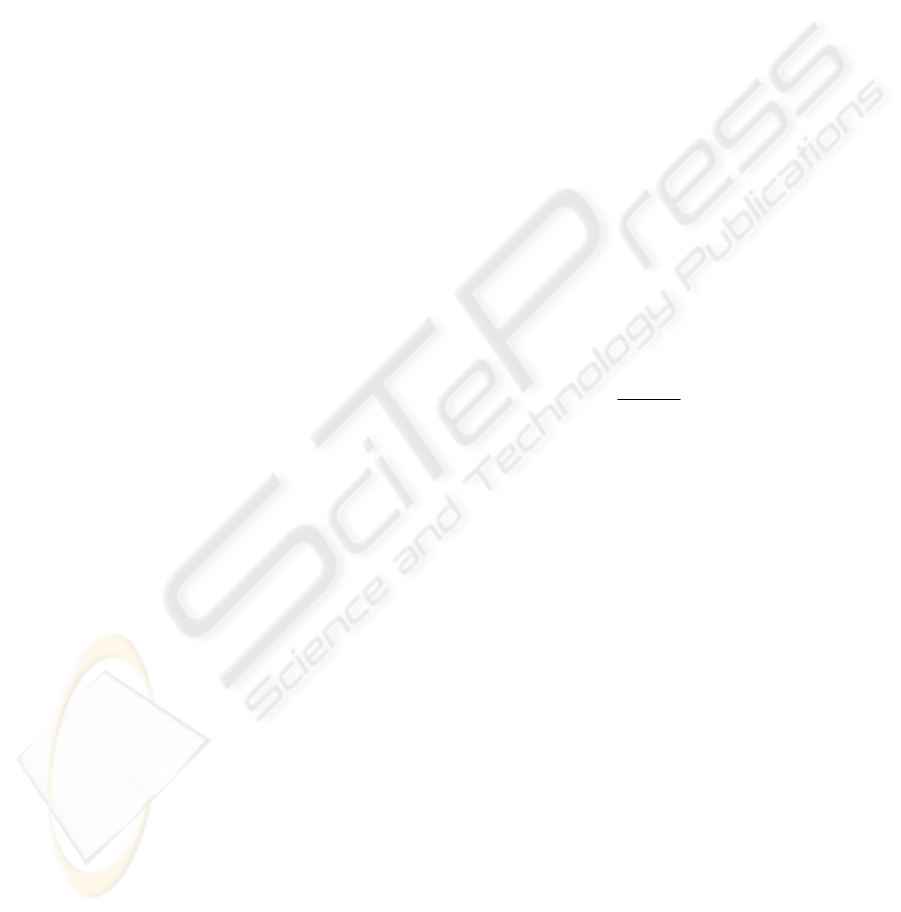
2 MDM: THEORETICAL BASIS
2.1 MDM Matrix
In any multicriterion analysis, the first step is the
information gathering phase that will apply to the
whole of the problem at hand, involving a survey of
the criteria and possible alternatives. Then, the
design phase consists of constructing the choice sets,
i.e. the alternatives, a finite and discrete set, in this
case.
Let us suppose there are m alternatives,
constituting the choice set A = {A
1
, A
2
,…, A
m
} and n
criteria, C
1
, C
2
,…, C
j
,…, C
n
. Thus, an mxn matrix of
evaluations, [a
ij
], characterizes a MCDM instance.
Each line of the matrix expresses the performance of
the alternative A
i
according to the n criteria, while
each column, C
j
, expresses the evaluations of all the
alternatives according to the criterion C
j
.
Leaving aside the problem of how to construct
the criteria proper, it is not an easy matter to
evaluate each alternative A
i
relative to a given
criterion C
j
, to obtain a coefficient a
ij
. In this paper,
we assume that these evaluations are known with
certainty.
Each of the referred criteria is originally
measured in its inherent unit (even we can have not
only numerical attributes). Thus, the MDM matrix
may presents evaluations of different nature. These
evaluations must be aggregated, taking into account
the preferences of the decision maker, to achieve a
global evaluation value for each alternative, on
which the overall ranking is based.
In our study, we consider the most widely known
aggregation method, i.e. the weighted (linear) sum,
also known as simple additive weight, whose main
advantage is that it is both, intuitive and simple to
apply. Although the method is very simple, we must
nevertheless carefully specify the starting data and
the transformation it undergo.
Weighted sum is a compensatory method.
Compensatory aggregation methods require the
different criteria evaluations and weights to be
settled down in compatible scale. This means that a
normalization procedure has to be executed to
transform figures of the matrix on a comparable
scale.
We suppose that the evaluations, a
ij
, result from
the nature of the attribute that criterion C
j
measures
on a numerical scale. We also assume that the
decision maker’s preferences can be stated by means
of positive weights, w
j
, which should be associated
to each criterion, C
j
.
For weights there is no problem because the
normalization is achieved making their sum equal to
1, dividing each weight, w
j
, by
j
w
∑
.
With respect to the evaluations, two classical
normalization procedures are considered for
comparison in the present study: proportionality
preservation and natural thresholds, which are
briefly described below.
For a given criterion C
j
, a normalization
procedure transforms the evaluations of the m
alternatives, (a
1j
, a
2j
,…, a
mj
), into a new vector,
(v
1j
, v
2j
,…, v
mj
), where v
ij
is the normalization of a
ij
.
Without loss of generality, we assume that all
criteria are going to be maximized and that values of
a
ij
are strictly positive (since, in the example of this
paper indeed it is).
2.2 MDM Matrix Normalization
Procedures
2.2.1 Proportionality Preservation
This procedure transforms the evaluation vector,
(a
1j
, a
2j
,…, a
mj
), of each criterion, C
j
, into a
normalized one by making
i
a
a
v
ij
i
ij
ij
∀= ,
max
(1)
Therefore, for each criterion, C
j
, the normalized
value of the best alternative is 1, and all the rest are
percentages of the maximum value, resulting in the
interval 0<v
ij
≤1, (we assume a
ij
>0).
It is the most widely used normalization
procedure. The main advantage of the method is that
the original proportion existing between the
evaluations of every pair of alternatives is preserved
after normalization, i.e. a
ij
/a
i´j
is equal to v
ij
/v
i´j
. This
is a very desirable property in many circumstances,
but it is not trivial to obtain, especially when dealing
with minimizing criteria. Even it may be impossible
to apply when there are evaluations with a zero
value or with different signs, because proportionality
is then not defined. The drawback of the result
vector is that the evaluations obtained by this
procedure are not forced to cover the complete
interval [0, 1].
2.2.2 Natural Thresholds
This procedure transforms the evaluation vector,
(a
1j
, a
2j
,…, a
mj
), of each criterion, C
j
, into a
normalized one by making
NORMALIZATION PROCEDURES ON MULTICRITERIA DECISION MAKING - An Example on Environmental
Problems
207