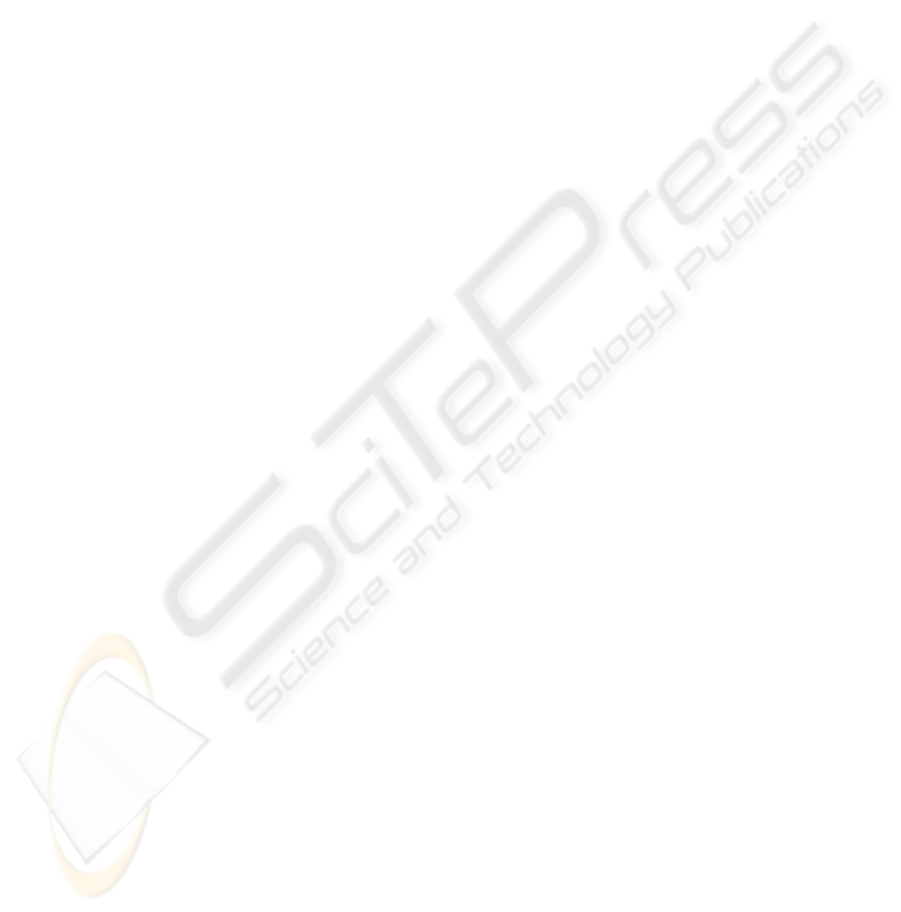
7 CONCLUSIONS AND FUTURE
WORK
The fundamental conclusion of this work is that the
described process presents a way to train fuzzy
systems in wind parameters downscaling. It is
clearly visible the improvement of the obtained wind
frequency distribution with regard to the regional
one. This fact implies that the optimized fuzzy
system contains information about how to correct
the wind direction over Jimena using the terrain
slopes. This acquired knowledge is the best
statistical solution founded through Genetic Fuzzy
Learning according to the variables and conditions
imposed to solve this particular problem in this
location. But the ultimate objective of this technique
should be the development of a generalized fuzzy
system able to work in many enviroments and
expanded to correct the wind speed, the most
important variable in wind resource evaluation. In
order to get the best agreement, this system should
evaluate more terrain characteristics as roughness,
heights and distances from obstacles to the target
point, topographic complexity, etc. Despite this
parameter inclusion, this new extended problem is
essentially similar to the one described in this paper.
The differences are related to the number of inputs
and outputs of the fuzzy system and the number and
characteristics of the terrains used in the training
process.
Since fuzzy logic is able to work with vague data, an
interesting application of this technique lies in
training fuzzy systems to work with low quality
stations. In fact, the stations used in this study can be
considered poor, because wind vanes and
anemometers are placed at 2 meters above ground
level and reported data could be affected by
obstacles and roughness. Another problem is that the
frequency of the provided mean wind speed and
direction is daily, far from the recommended ten-
minutes interval. In opposition to these
inconvenients, the use of this information allows to
acquire easily a considerable quantity of long term
time series of real measurements of the area. So,
once this general fuzzy system is obtained, the
duration and requirements of the wind resource
evaluation of large areas could be strongly reduced.
Finally, the technique exposed could be also applied
to all that processes in which wind and terrain are
closely relationed as fire propagation or erosion.
REFERENCES
Boehme, Thomas A.Robin Wallace, Hindcasting Hourly
Wind Power across Scotland Based on Met Station
Data, Wind Energy 2008; 11: 233-244.
Palma, J.M.L.M., F.A. Castro, L.F. Ribeiro, A.H.
Rodrigues, A.P. Pinto; Linear and nonlinear models in
wind resource assessment and wind turbine micro-
siting in complex terrain; Journal of Wind Engineering
and Industrial Aerodynamics 96 (2008) 2308– 2326.
Gastón, Martín, Edurne Pascal, Laura Frías, Ignacio Martí,
Uxue Irigoyen, Elena Cantero, Sergio Lozano,
Yolanda Loureiro; Wind resources map of Spain at
mesoscale. Methodology and validation. Wind Energy
Department, National Renewable Energy Center
(CENER).
Gutiérrez, J. M., Rafael Cano, Antonio S. Cofiño, Carmen
M. Sordo; Redes probabilísticas y neuronales
aplicadas a las ciencias atmosféricas, INM,
Ministerio de Medio Ambiente, Madrid (2006).
Louka, P., G. Galanis, N. Siebert, G. Kariniotakis, P.
Katsafados, I. Pytharoulis, G. Kallos. Improvements
in wind speed forecasts for wind power prediction
purposes using Kalman filtering; Journal of Wind
Engineering and Industrial Aerodynamics 96 (2008)
2348– 2362.
Cellura, M., G. Cirrincioneb, A. Marvuglia, A. Miraouic;
Wind speed spatial estimation for energy planning in
Sicily: A neural kriging application; Renewable
Energy 33 (2008) 1251-1266.
Matheron, G. , Principles of geostatistics, Econ. Geol. 58
(1963) 1246-1266
Thiebaux, H.J., M.A. Pedder, Spatial Objective Analysis
with Applications in Atmospheric Sciences, Academic
Press, New York, 1987, 299 pp.
Sen Zekai, Ahmet Duran Sahin, Wind energy evaluation
in some parts of Turkey, J. Wind Eng. Ind. Aerodyn.,
74-76 (1998) 345-353.
Agüera, A., J. G. Ramiro, J. Melgar, J. J. G. de la Rosa,
J.C. Palomares, A. Moreno. “Categorization of
minimum error forecasting zones using a geostatistic
wind model”; Proceedings Clean Electrical Power
2009 Capri (Italy), IEEE.
Zadeh, Lofti A.; Fuzzy sets, fuzzy logic and fuzzy systems,
Advances in fuzzy Systems – Applications and Theory
vol..6. World Scientific. 1997.
TRAINING A FUZZY SYSTEM IN WIND CLIMATOLOGIES DOWNSCALING
243