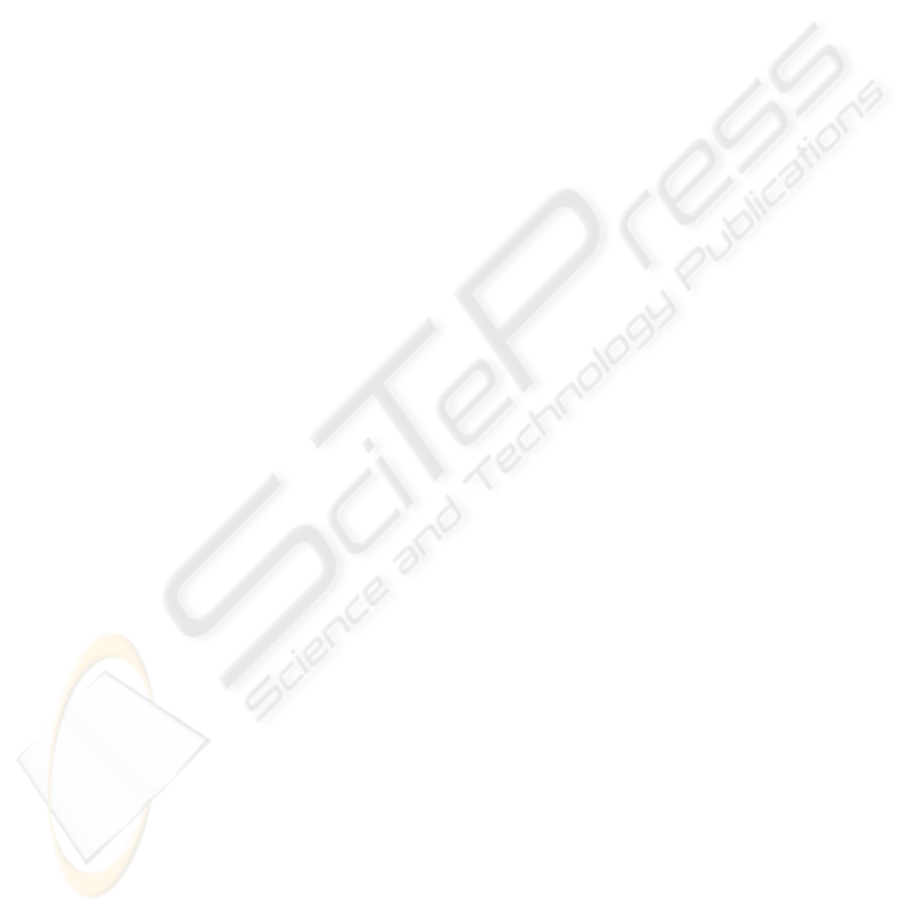
5 CONCLUSIONS
AND FURTHER WORK
Currently, in the ICU environment, the decision
making is based on severity scores like SOFA and in
the intensivists’ empirical experience. However, this
process has some limitations. It is based on
probabilistic scores and doesn’t take into account
import information that might be hidden regarding
the patient’s clinical status and its evolution (e.g.
bedside monitored physiological parameters).
Research has evolved towards the inclusion of
new variables and DM techniques in order to predict
organ dysfunction/failure and outcome.
This paper presented new DM models for the
INTCare system, with data gathered in real-time at
the ICU of HGSA, in Porto, Portugal. The
techniques tested have been used before in the
research of the INTCare. The novelty of this
approach relies on the use of real-time data and a
finer grained prediction, i.e. the prediction of organ
failure and outcome for the next hour. Moreover,
new variables were used for the models generation
(e.g. racios). The experiments carried out focused on
finding the best combination of techniques and
features selection. For each technique, several
configurations were tested in order to achieve the
best results possible.
In this paper, we have proven that it is possible
to use online and real-time data to generate highly
accurate models, which is a great advance in the
context of a situated intelligent decision support
system like INTCare. The main goal in ICU is to
avoid or reverse organ failure. We expect that, with
an hourly prediction of the patients’ clinical status, it
will be possible for intensivists to have a timely
intervention so that worst complications may be
avoided.
As pointed in Section 3, the data used came from
three sources, including a paper-based nursing
record. However, we are developing an ENR that
collects hourly and in real-time all the necessary
data to feed the DM models (Santos, 2009).
These models must be tested in the ICU with
new data in real-time and new models will be
generated in order to try to achieve the best results
possible.
Advances in the prediction of organ failure and
outcome might include the development of new
strategies presented in this paper regarding the
features selection (e.g., the inclusion of new
variables like the SOFA score of the other systems,
and ratios). Moreover, it should be pointed that the
effort was put into the prediction of each organ
system individually and not the systems altogether.
Hence, it is expected that different systems have
different variables and techniques for their
prediction.
Although we have achieved very good results, it
should be stressed out that the dataset used was a
small population of patients. We believe that it will
be possible to maintain a good degree of prediction
because it is known that clinical data is very stable
(Silva, 2007).
The next step is the deployment of the models
and the analysis of their impact on the ICU
environment.
REFERENCES
Abelha, A., Machado, J., Alves, V., Neves, J., 2004. Data
warehousing through multi-agent systems in the
medical arena. In 1st international Conference on
Knowledge Engineering and Decision Support. Porto,
Portugal.
Amaral, A.C., Andrade, F.M., Moreno, R., 2005. Use of
the Sequential Organ Failure Assessment score as a
severity score. In Intensive Care Med, 31, pp. 243-249.
Bellazzi, R., Zupan, B., 2008. Predictive Data Mining in
clinical medicine: current issues. In Int J Med Inform,
pp. 81-97.
Cios, K., Moore, G., 2002. Uniqueness of medical data
mining. In Artificial Intelligence in Medicine 26, 1–24.
Fayyad, U., 1998. Mining databases: Towards algorithms
for knowledge discovery. In IEEE Data Eng. Bull., pp.
39-48.
Fayyad, U., Piatetsky-Shapiro, G., Smyth, P., 1996. The
KDD process for extracting useful knowledge from
volumes of data. In Communications of the ACM, 39,
pp. 27-34.
Gago, P., Santos, M. F., 2008. Towards an intelligent
decision support system for intensive care units. In
The 18th european conference on artificial
intelligence.
Gago, P., Santos, M. F., Silva, Á., Neves, J., Gomes, L.,
2006. INTCare: A knowledge discovery based
intelligent decision support system for intensive care
medicine: an overview. In Journal of decision
systems, pp. 241-259.
Lasco, T., Bhagwat, J., Zou, K., Ohno-Machado, L., 2005.
The use of receiver operating characteristic curves in
biomedical informatics. In J. of Biomedical
Informatics, 38(5):404–415.
Le Gall, J., 2005. The use of severity scores in the
intensive care unit. In Intens Care Med 2005;31:1618-
23.
Miranda, R., 1998. The effect of harmonizing and
standardizing the nursing tasks on intensive care units
of the European Community, second European
intensive care unit study (EURICUS-II). In Technical
report BMH4-CT96-0817, Health services research
unit – Groningen university hospital, The Netherlands.
HOURLY PREDICTION OF ORGAN FAILURE AND OUTCOME IN INTENSIVE CARE BASED ON DATA MINING
TECHNIQUES
275