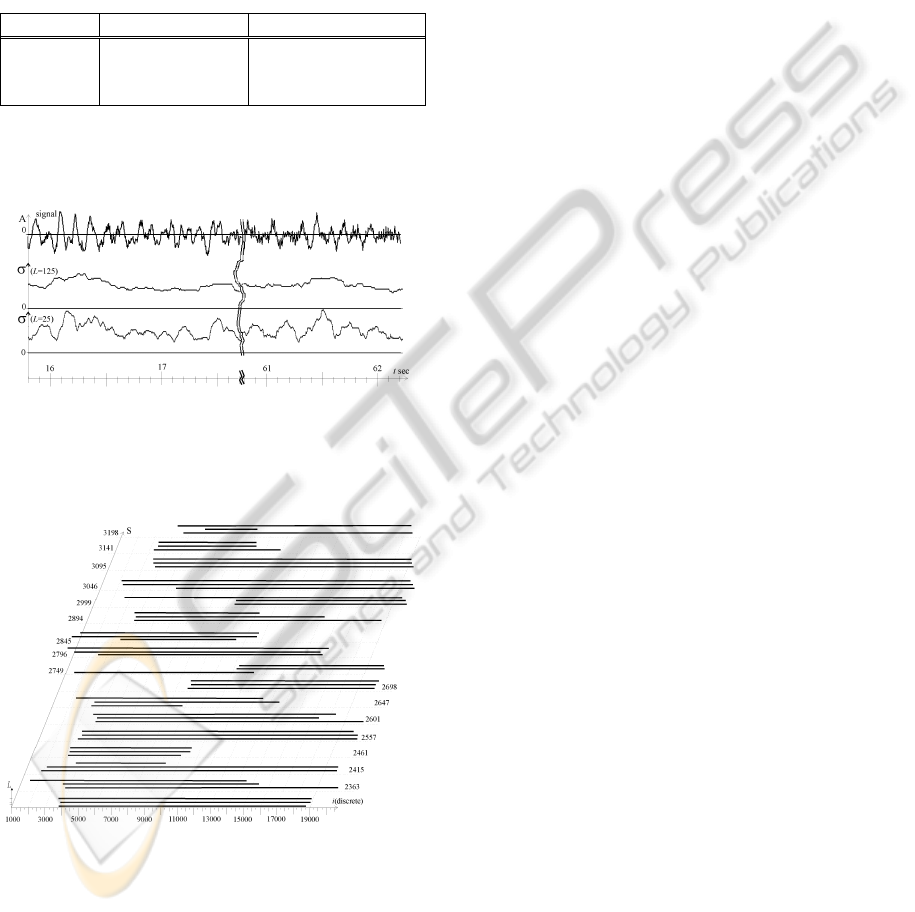
L∈{25, 75, 125}.
Estimations of signal locus in time for S∼0 m are
given in the Table 2 (all the representative
probabilities are equal to 1).
The processing and analysis data were obtained
by means of interactive computer system of
designing and support of one-dimensional weighed
order statistics filters (
V. I. Znak, 2009).
Table 2: Estimations of signal locus in time for S∼0 m.
L h
min
h
max
t
1
÷t
2
25 6÷415 3870÷18883
75 14÷396 3846÷18908
125 16÷390 3821÷18933
By way of example, an image of investigated
signal (for S=2647 m) and appropriate dispersions is
shown in Fig. 2.
Figure 2: An image of signal and appropriate dispersions
for L=25, L=125 (S=2647 m).
Estimations of the data from the Table 1 are
given in Fig. 3.
Figure 3: Estimations of the time locus of the signal for
running basis L∈{25, 75, 125} and for different distances.
5 CONCLUSIONS
We have considered the approach of cluster analysis
of periodic signals, proposed the formal conditions
which must be satisfied by a period of signal
existence, and given some results of analysis of real
data recorded in field conditions. Analysis of the
results obtained by studying real signals allows us to
say that the approach in question can result in close
estimations of a locus in time of a pure signal, and in
less close estimations of a locus in time of noisy
signals.
Our main objective was restricted by
development of the method of formalized analysis of
periodic signals for estimation of their period of
existence. We have not concerned methods of
improving signals as it is a theme of separate
investigation. We suppose that more exact decisions
can be attained by attracting analysis of the left and
the right uniformity of cluster families (Znak V. I.,
2009) and frequency processing (Znak V. I., 2005).
Cluster families, which reflect a locus of a signal on
its boundaries, must have a higher uniformity than
for others.
The work is supported by the grant 09-07-00100.
REFERENCES
Gurvich I. I., Boganic G. N., 1980. Seismic research.
Moscow, “Nedra” (in Russia).
Davidova E. A., Copilevich E. A., Mushin I. A., 2002.
Spectral-time method for a mapping of types of
geological layers, Reports of RAS, 385(5), pp. 682-
684, (in Russia).
Nikitin A. A., 2006. New tricks of geophysical data
processing and their well-known analogous.
Geophysics, No 4, pp. 11-15 (in Russia).
E. Baziw, 1994. Implementation of the Principle Phase
Decomposition Algorithm,” in Proc. IEEE
Transactions on signal processing. July 2007 45 (6),
1775–1785.
Znak V. I., and Grachev O. V., 2009. Some Issues in
improving quality of noisy periodic signals and
estimating their parameters and characteristics
numerically by using a cluster approach: problem
statement. Numerical Analysis and Applications, 2(1),
pp. 34–45.
Znak V. I., 2009. Some aspects of estimating the detection
rate of a periodic signal in noisy data and the time
position of its components. Pattern Recognition and
Image Analysis, 19(3), pp. 539-545.
Znak V. I., 2005. Co-Phased Median Filters, Some
Peculiarities of Sweep Signal Processing.
Mathematical Geology, 37(2), pp. 207–221.
V. I. Znak, 2009. Some Questions of Computer Support of
Designing and Accompanying of One-Dimensional
WOS Filters. Journal of Siberian Federal University,
Mathematics & Physics, 2(1), pp. 78–82 (in Russia).
ON SOME PECULIARITIES OF CLUSTER ANALYSIS OF PERIODIC SIGNALS
229