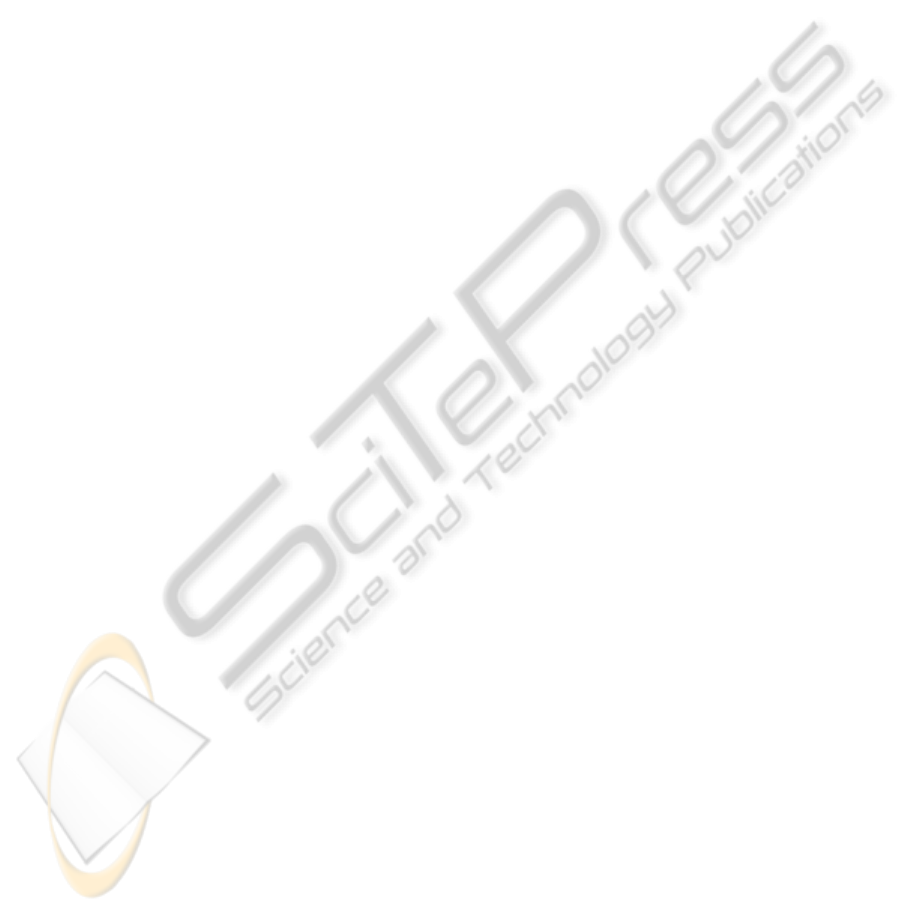
One way of adding semantics to SLAM may be
the introduction of human spatial concepts into
maps. Humans usually do not use metrics to locate
themselves but rather object-centric concepts (“I am
near the sink” and not “I am on [12, 59]”) and they
fluently switch between reference points rather than
using global coordinates. Moreover, the presence of
certain objects is often an important clue to recog-
nize a place. This problem is addressed in
(Vasudevan, et al., 2007). Here, the world is repre-
sented topologically; place recognition is performed
based on probability of presence of objects in an
indoor environment. The work shows a study aimed
to understand human concepts of place recognition.
It proposes that humans understand places by pres-
ence or absence of significant objects. Place classifi-
cation by presence of objects has been used by
(Galindo, et al., 2005), where low-level spatial in-
formation is linked to high-level semantics. Their
robot has interfaced with humans and performed
tasks based on high-level commands involving ro-
bots “understanding” of the meaning of place names
for path planning. However, object recognition is
black-boxed here. In (Persson, et al., 2007) a system
is developed to map an outdoor area, generating a
semantic map with buildings and non-buildings
labelled. In (Nüchter, et al., 2008), a more general
system is presented with a robot equipped by a 3D
laser scanner evolving in an indoor environment and
constructing a 3D semantic map. The processing is
based on Prolog clauses enveloping pre-designed
prior knowledge about the environment enabling the
robot to reason about the environment. In (Ekvall, et
al., 2006), object recognition is performed by a robot
equipped by a laser range finder and a camera. A
semantic structure is extracted from the environment
and integrated to robots map. Another semantic
mapping technique is shown in (Meger, et al., 2008)
including an attention system.
3 IMAGE SEGMENTATION AND
SCENE INTERPRETATION
Section 2 showed the pertinence of semantic SLAM
for state of the art robotic mapping. It is this field, on
which we are focusing our research. Our motivation
comes from the natural ability of human beings to
navigate seamlessly in complex environments. To
describe a place, we use often very fuzzy language
expressions and approximation (see (Vasudevan, et
al., 2007)) in contrast to current SLAM algorithms.
An interesting point is that people are able to infer
distance of an object using its apparent size and their
experience of object’s true size. Recognition of
objects and understanding their nature is an integral
part of “human SLAM”. We believe that application
of semantics and human inspired scene description
could bring a considerable benefit in development of
robust SLAM applications for autonomous robotics.
For scene interpretation, the image has to be
segmented first. Although many image segmentation
algorithms exist (see (Lucchese, et al., 2001) for a
reference), not all are suitable for mobile robotics
due to need of real-time processing. We implement a
fast algorithm that breaks the input image into parts
containing similar colors with less attention to the
brightness. We have chosen the YCbCr color model
with Y channel dedicated to the luminance compo-
nent of the image and other two channels Cb and Cr
containing respectively the blue and the red chro-
minance component. Unlike RGB, the YCbCr model
separates the luminance and the color into different
channels making it more practical for our purposes.
Our algorithm works in a coarse-to-fine manner.
First, the contrast is stretched and median filter is
applied to the Cr and Cb components. Then the first
available pixel not belonging to a detected compo-
nent is chosen as a seed point. Eq. 1 captures how a
seed point is used to extract the segment of interest
(S). P stands for all the pixels in the image, whereas
p is the actually examined pixel. Predicate C is true
if its arguments (p, p
s
) are in four-connectivity. I
stands for the pixel’s intensity. Seed pixel is denoted
by p
s
. A pixel of the image belongs to the segment S
if the difference of intensities of the current and the
seed pixel is smaller than a threshold and there exists
a four-connectivity between it and the seed pixel
∀p∈P; C(p, p
s
) & |I(p) – I(p
s
)| < ε → p ∈ S .
(1)
Using this on both chroma sub-images we obtain
segments denoted as S
Cr
and S
Cb
. A new segment S
is then obtained following Eq. 2 as the intersection
of segments found on both chroma sub-images with-
out pixels already belonging to an existing segment
S = S
Cr
∩ S
Cb
- S
all
. (2)
At the end of the scan, a provisory map of de-
tected segments is available, but the image is often
oversegmented. In the second step, all the segments
are sorted by their area and beginning with the larg-
est one the segmentation is run again. This time the
seed point is determined as the pixel from the skele-
ton whose distance to its closest contour pixel is
maximal. By this step, similar segments from the
TOWARDS HUMAN INSPIRED SEMANTIC SLAM
361